Robust category recognition based on deep templates for educational mobile applications.
2023 IEEE International Conference on Big Data (BigData)(2023)
摘要
Popularity of mobile vision applications constantly increases. These Artificial Intelligence technology-driven solutions exhibit high potential for the dynamically-changing landscape of education, in which they could be used as powerful tools to engage and motivate learners. The integration of game-like elements into educational software can be a very effective approach to capture the attention of students by providing interactive and immersive learning experiences. That is why in this study we present a category recognition method for computationally-limited devices such as smartphones to be used in a cost-efficient manner in the areas connected to interactive education. We use small and efficient ImageNet-trained Convolutional Neural Networks and Deep Template Matching to create a classification solution. To test our solution, we construct a specialized image dataset with different toys and items that could be suitable for early-stage education to engage young learners. We perform an extensive study on how the number of images used to create templates and different similarity/distance measures applied for matching impact the accuracy to provide practical observations that can be used to create real-life game-like elements for educational software. Our results indicate that utilizing more templates and similarity measures results in better generalization, representativeness, and robustness, particularly when it comes to rotation, which is crucial for mobile applications. We release our data and code via our GitHub repository at https://github.com/iitis/EduToyz.
更多查看译文
关键词
Deep Template Matching,CNN,category recognition,Education
AI 理解论文
溯源树
样例
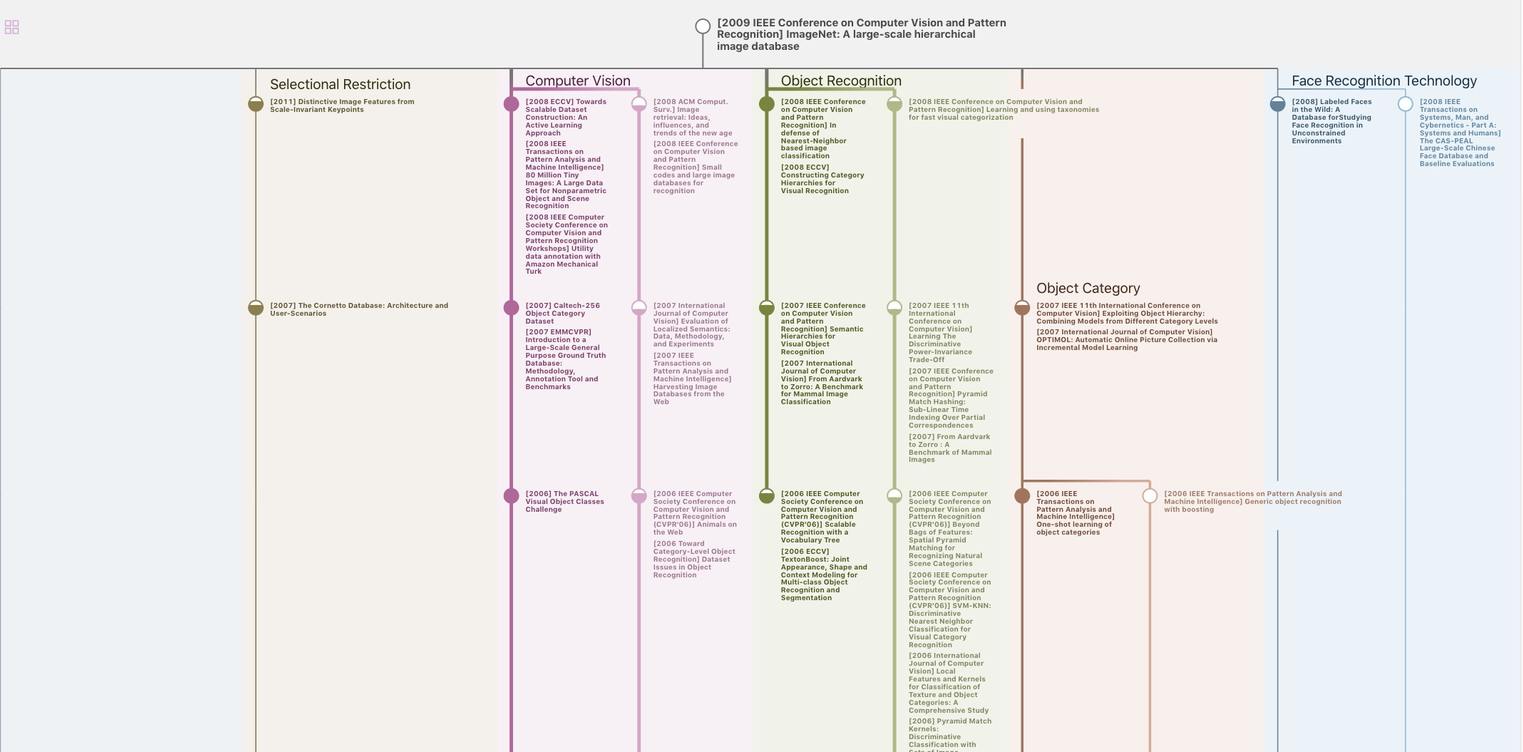
生成溯源树,研究论文发展脉络
Chat Paper
正在生成论文摘要