Learning Cataract Severity Using the Contrast Sensetivity Scale: A Thick Data Approach.
2023 IEEE International Conference on Big Data (BigData)(2023)
摘要
Cataract image severity classification according to contrast sensitivity chart like VTSC represents a challenge to classical machine learning. Typically cataract images hide significant retina features because of the fogy nature of these images. Contrast sensitivity measurement provides important information on the functioning of the visual system that cannot simple detected by the visual acuity tests. In this paper, we are introducing a framework for segmenting fundus images into regions of salient contrast. The framework start by converting the RGB color channels into joint entropy where it can be used to categorized anchor images into contrast based classes. It has been found that classes like normal fundus, fundus with cataract or fundus having cataract with additional complications like availability of cotton-wool spots or fibrosis. We found that contrast sensitivity scoring correlate nicely with the joint entropy scoring. For this purpose, our last level of the framework employs a triplet loss Siamese neural network that has been trained on few contrast classified fundus images to detect the severity of the cataract. We used a similar contrast sensitivity scale like those used by the optometrist with classification rate that reaches 72%.
更多查看译文
关键词
Thick Data Analytics,Cataract Severity,Siamese Neural Network,Contrast Sensitivity Scoring,Joint Entropy
AI 理解论文
溯源树
样例
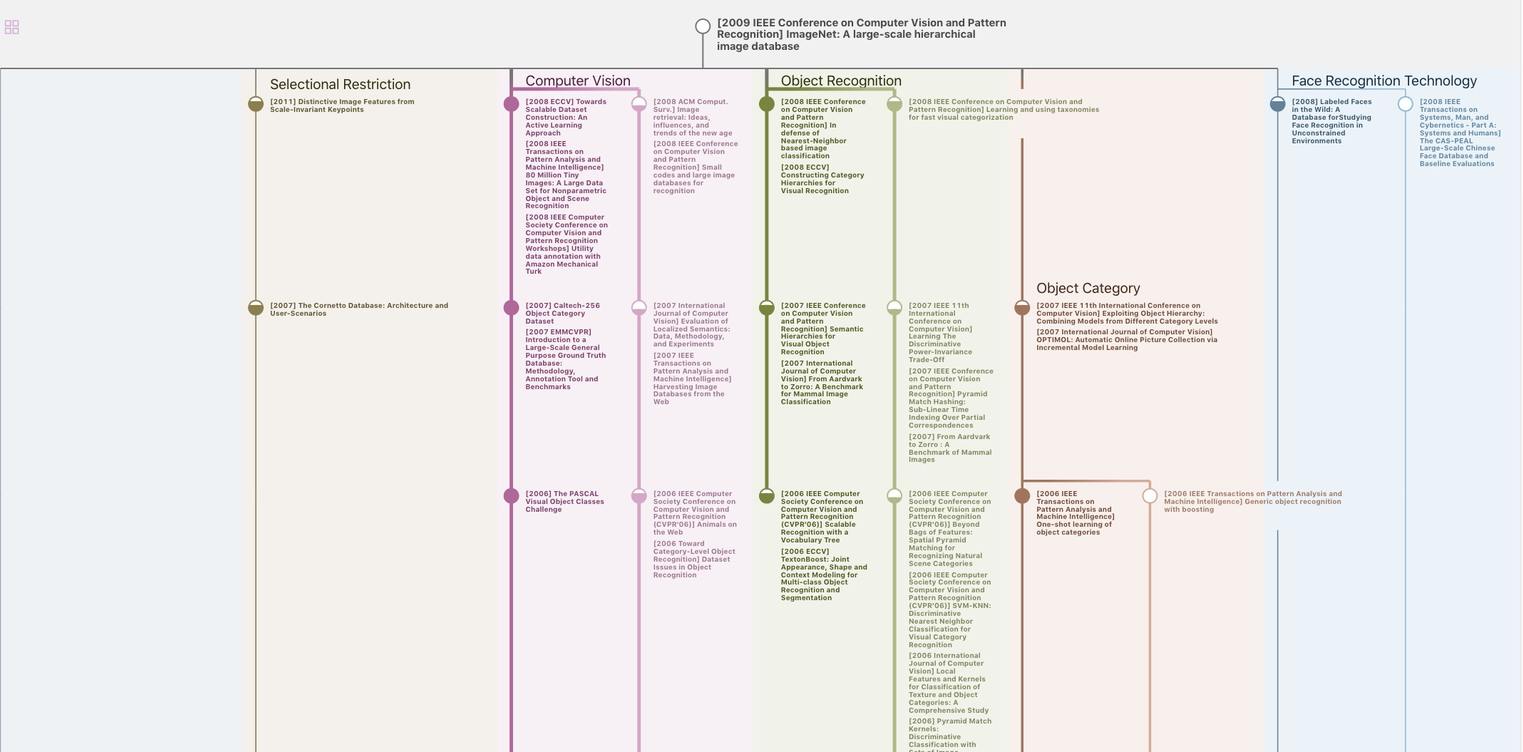
生成溯源树,研究论文发展脉络
Chat Paper
正在生成论文摘要