Predictive Analytics in IoT and CPS: Enhancing Industrial Machinery Reliability through Sensor Data-Driven Remaining Useful Life Estimation.
2023 IEEE International Conference on Big Data (BigData)(2023)
摘要
The rise of the Internet of Things (IoT) and Cyber-Physical Systems (CPS) has brought about a new era of connectivity, building intelligence into the very structure of our society. There are both challenges and possibilities that come with this change, especially when it comes to maintaining industrial machinery. When it comes to big, important machines like electrical transformers, predictive maintenance is very important because the costs of unplanned downtimes can be too high to bear. This essay looks at how the IoT can be used to actively keep an eye on these tools, with a focus on how sensor data can be collected and used to keep operations running smoothly. We look at how machine learning (ML) and deep learning (DL) can be used to look at this data and guess how much useful life a piece of machinery still has. This lets the machine be shut down for repair and keeps it from breaking down without warning. Even though these technologies have clear benefits, there isn’t much written about how to combine them into a unified business process for predictive analytics. This study fills in the blanks by proposing a business process made just for analyzing sensor data and predicting Remaining Useful Life (RUL) of industrial machinery. To facilitate testing of the proposed business process, we provide a prototype implementation and discuss its details. We also present a way to evaluate this business process by applying the prototype implementation to a real-world dataset. The evaluation results show that the proposed business process is promising.
更多查看译文
关键词
IoT,CPS,predictive maintenance,RUL estimation,sensor data analytics,deep learning,industrial reliability,business process design
AI 理解论文
溯源树
样例
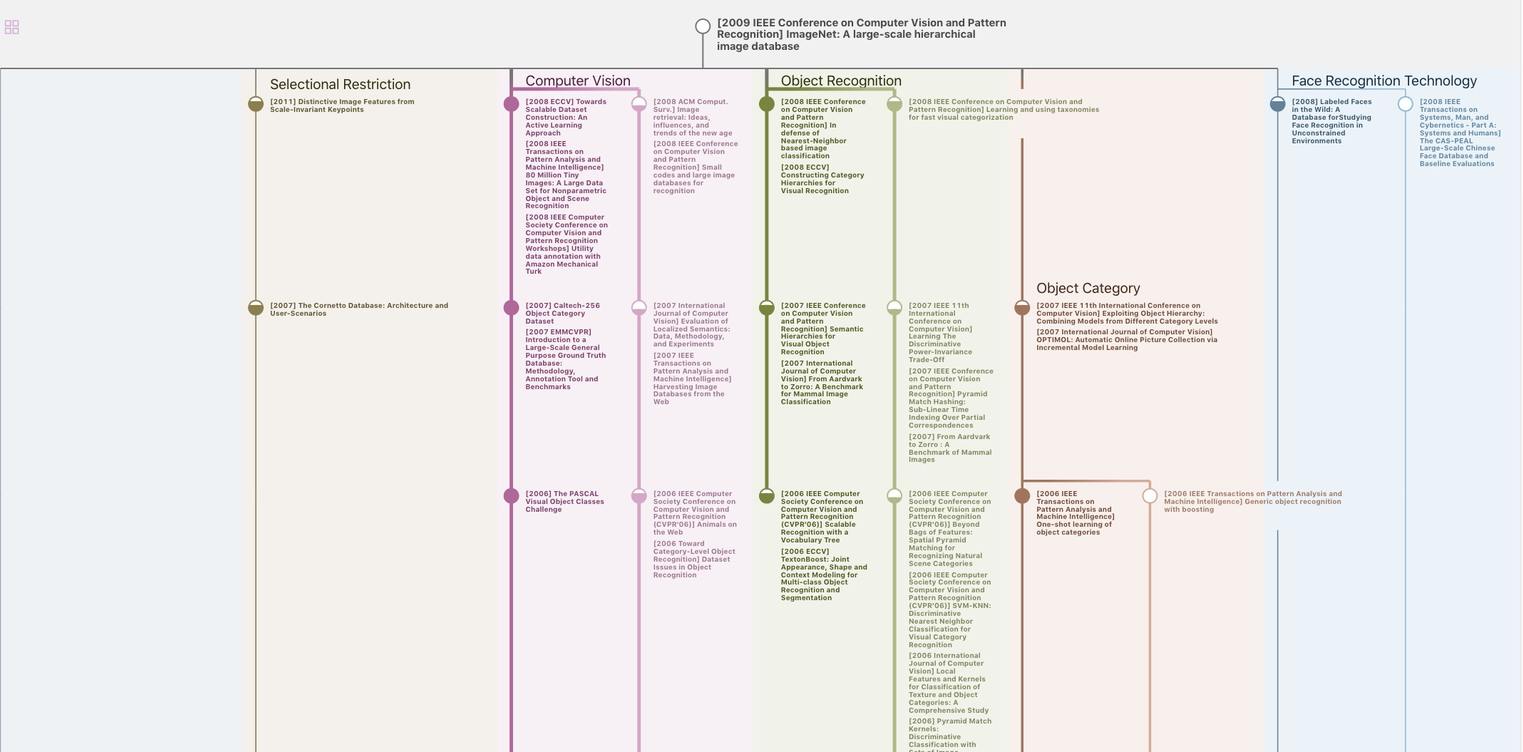
生成溯源树,研究论文发展脉络
Chat Paper
正在生成论文摘要