Hybrid Attack Graph Generation with Graph Convolutional Deep-Q Learning.
2023 IEEE International Conference on Big Data (BigData)(2023)
摘要
Critical infrastructure such as power grids are becoming increasingly complex, connected, and vulnerable. Effective risk mitigation for these cyber-physical energy systems (CPES), requires preemptive knowledge of likely adversarial attack scenarios. However, the scarcity of documented attack sequences hinders this process. We propose a data-driven Graph Convolutional Deep-Q Network (GCDQ) to address this lack of data through generating Hybrid Attack Graphs (HAGs), a graphical representation of CPES attack sequences. By leveraging limited real-world observations from the MITRE ATT&CK knowledge base, our GCDQ model synthesizes realistic graphs with the targeted attribute of minimum detectability via reinforcement learning. This generative model is the first step in creating a tool to substantially boost the attack sequence dataset and enhance the performance of CPES defense-related tasks by providing insights into likely attack sequences with given attributes.
更多查看译文
关键词
graph generation,cyber attack modeling,attribute targeting
AI 理解论文
溯源树
样例
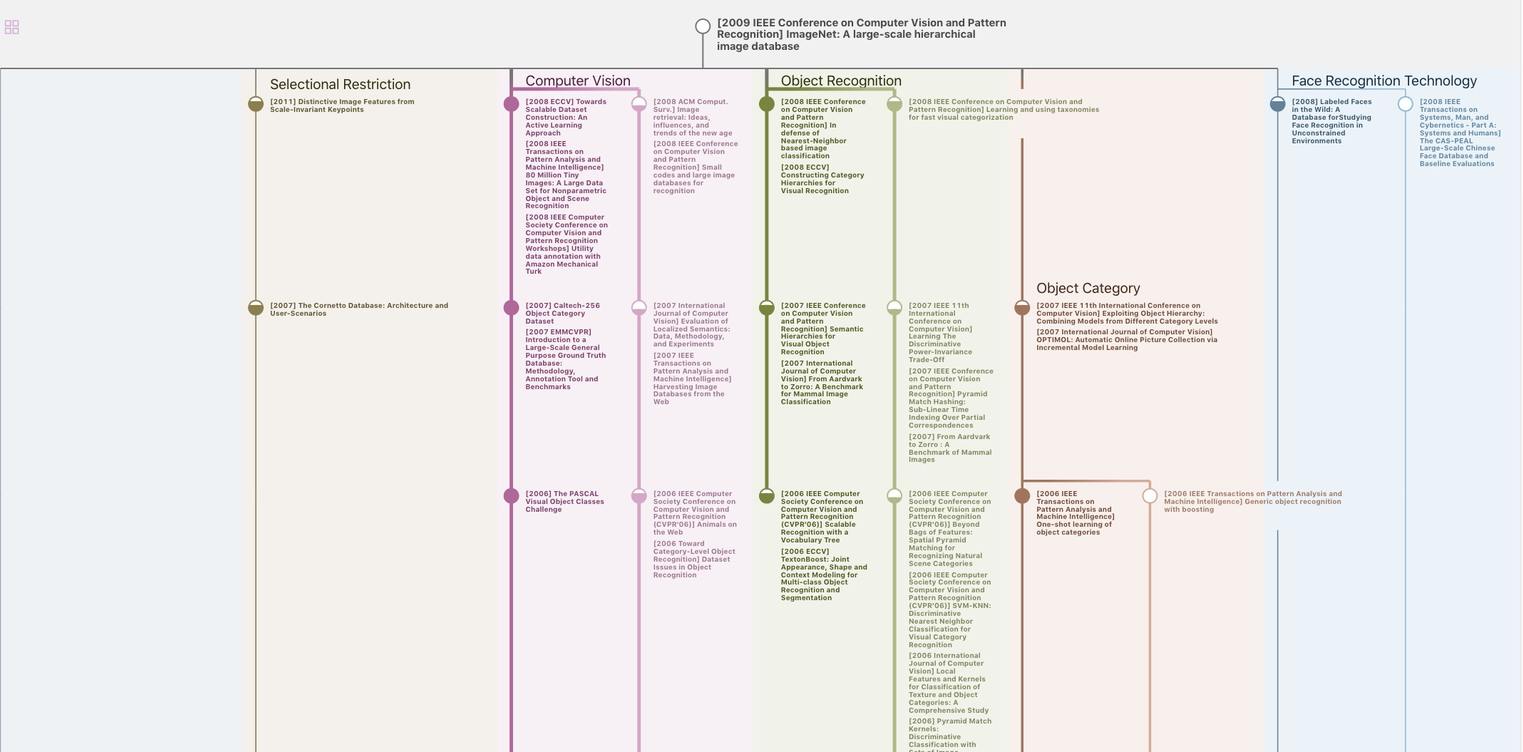
生成溯源树,研究论文发展脉络
Chat Paper
正在生成论文摘要