Dual-Path Side Information Fusion for Sequential Recommendation.
2023 IEEE International Conference on Big Data (BigData)(2023)
摘要
Sequential recommendations are designed to capture user preferences based on their past actions and predict the items they may interact with in the next moment. Benefiting from the self-attention mechanism, methods that utilize side information (such as item categories or brand) to improve the prediction performance of sequential recommendation have yielded promising results. Previous approaches typically directly fuses side information embeddings into item embeddings as inputs to the model. However, this fusion approach overlooks the distinctions in various types of information in sequential pattern inference, and also failing to fully model the relationship between items and side information. In this work, we propose a Dual-Path Side Information Fusion method (DPIF) to better utilize side information for improved recommendation performance. Our model employs two parallel paths for side information fusion modeling. One path obtains the relationship representation within the items and the side information, and the other path obtains the relationship representation between the items and the side information. Subsequently, an attention-based adaptive fusion module is utilized to combine inter-attribute relationship and intra-attribute relationship representation, generating the final user preferences. Extensive experiments were conducted on four real-world datasets, demonstrating the effectiveness of the introduced model. Our source code is available at https://github.com/ZhangYu-x/DPIF.
更多查看译文
关键词
Sequential Recommendation,Attention Mechanism,Side Information Fusion
AI 理解论文
溯源树
样例
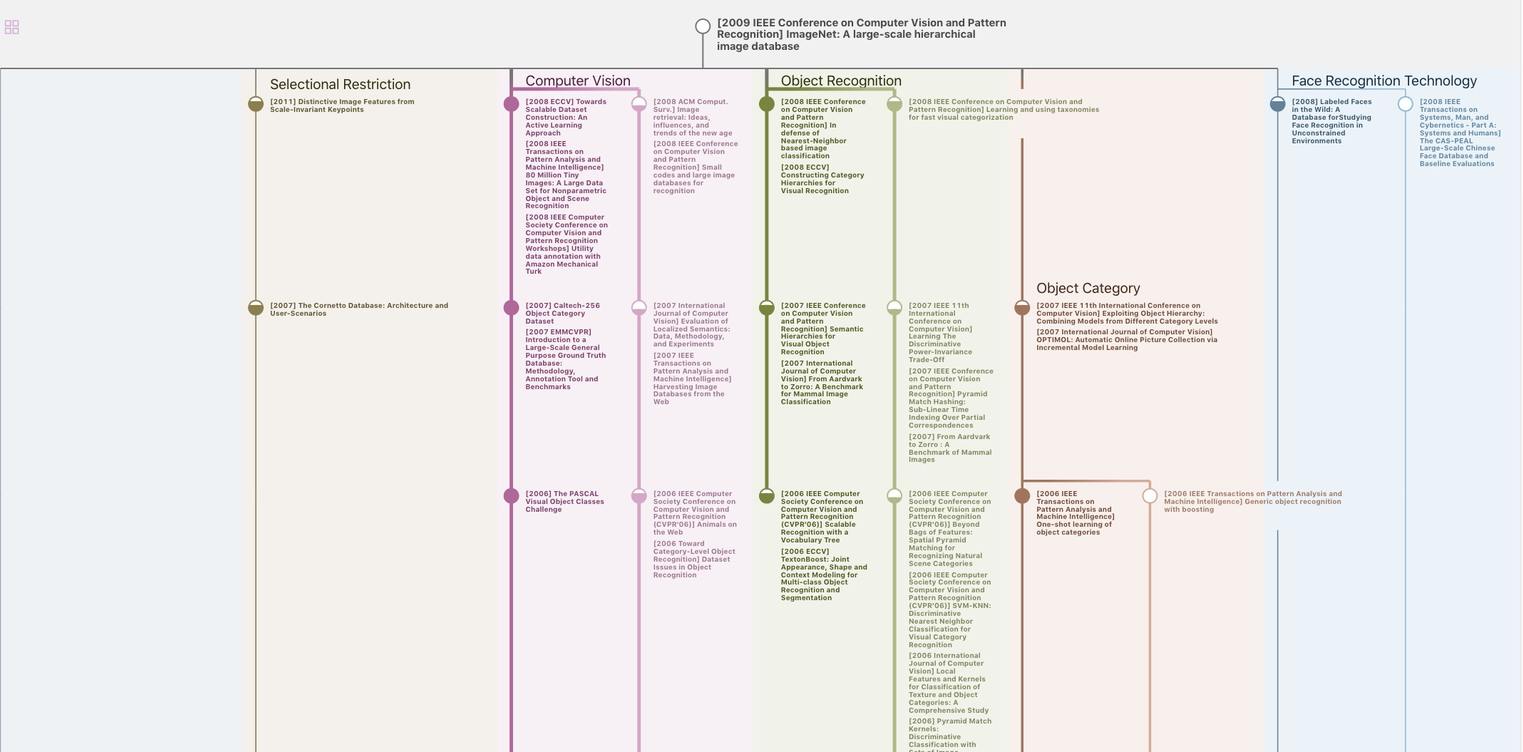
生成溯源树,研究论文发展脉络
Chat Paper
正在生成论文摘要