An Augmentation-agnostic Semantic Preserving Technique for Data Generation.
2023 IEEE International Conference on Big Data (BigData)(2023)
摘要
Data generation is becoming an increasingly important issue in the field of machine learning due to the high cost of data collection and the privacy concerns associated with raw data. Generating new data with high fidelity is extremely challenging because even a minor perturbation in high-dimensional data may alter its semantic meaning. The challenge becomes particularly acute in multivariate time-series data as it often exhibits human-imperceptible temporal patterns and lacks standard representation. To address this issue, we propose a straightforward yet effective technique that helps preserve the fidelity of the original data. Experimental results demonstrate that our technique enhances the performance of time-series forecasting model.
更多查看译文
关键词
Data Augmentation,Data Generation,Synthetic Data,Data Interpolation
AI 理解论文
溯源树
样例
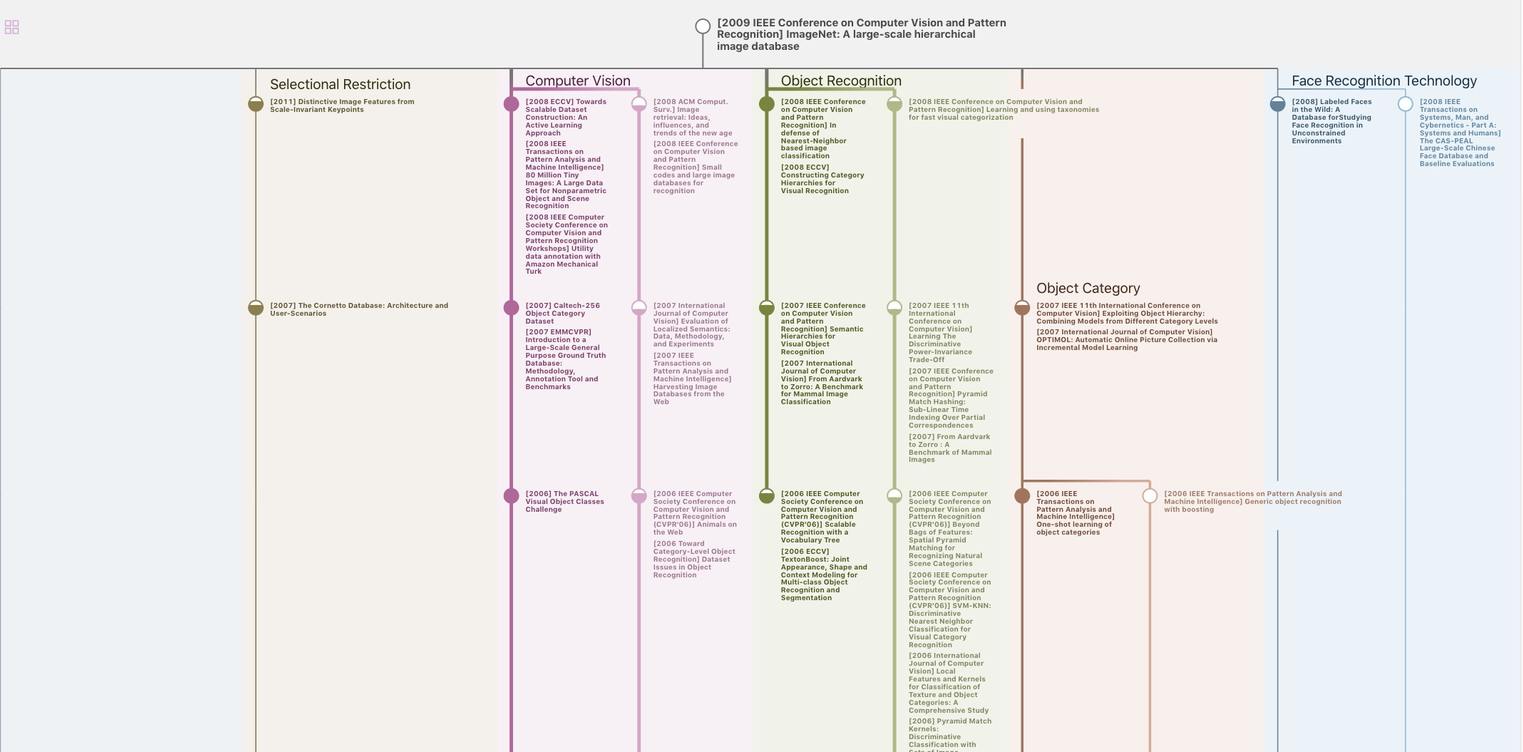
生成溯源树,研究论文发展脉络
Chat Paper
正在生成论文摘要