A Prior-Guided Meta-Weight Network for Long-tailed Noisy Data in Item Categorization.
2023 IEEE International Conference on Big Data (BigData)(2023)
摘要
Item categorization task aims to automatically infer the product category of an item based on its textual description. As a fundamental task in eCommerce domain, item categorization is widely adopted in many important applications such as product search, query understanding and product recommendation. However, this task faces several challenges. First, eCommerce data usually suffers from noisy facts since many key product values are self-reported by individual sellers and cannot be fully verified by experts. Second, eCommerce data usually follows the long-tail data distribution in which class distribution is highly imbalanced. To handle these challenges, some existing efforts simply combine approaches that are developed for noisy data and long-tail data separately. However, such a straightforward combination may not achieve satisfactory performance. In this paper, we propose a performance-driven Prior-Guided Meta-Weight Network (PGMWN) which handles the two challenges in a principled way. The proposed framework involves a meta re-weighting strategy to estimate the weights of samples mainly based on performance changes. Moreover, we leverage important data statistics to guide the meta reweighting mechanism towards distribution-aware weights. A self-supervised representation learning component is utilized to further improve the framework’s ability to address those two issues. To evaluate the effectiveness of the proposed PGMWN framework, comprehensive experiments are conducted on three public real-world datasets collected from Amazon. The proposed framework outperforms several state-of-the-art baselines in terms of various evaluation metrics. The experimental results show that the proposed model is able to handle the long tail data distribution and label noise issues and is effective in the item categorization task.
更多查看译文
关键词
item categorization,meta weight learning,long tail,noisy label
AI 理解论文
溯源树
样例
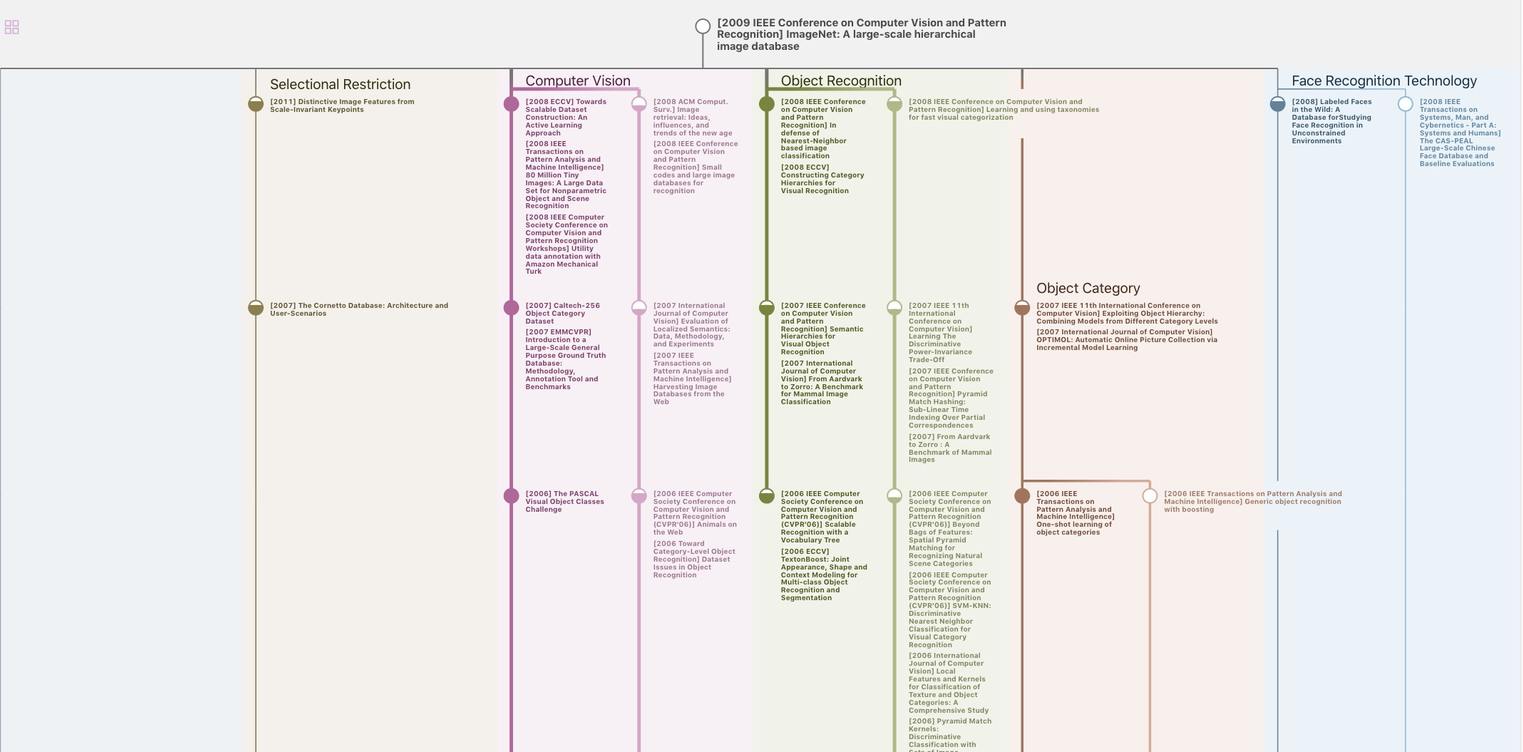
生成溯源树,研究论文发展脉络
Chat Paper
正在生成论文摘要