SEED: An Effective Model for Highly-Skewed Streamflow Time Series Data Forecasting.
2023 IEEE International Conference on Big Data (BigData)(2023)
摘要
Accurate time series forecasting is crucial in various domains, but predicting highly-skewed and heavy-tailed univariate series poses challenges. We introduce the Segment-Expandable Encoder-Decoder (SEED) model, designed for such time series. SEED incorporates segment representation learning, Kullback-Leibler divergence regularization, and an importance-enhanced sampling policy. We tested our model on the 3-day ahead single-shot prediction task on four hydrologic datasets. Experimental results demonstrate SEED’s effectiveness in optimizing the forecasting process (10-30% of root mean square error reductions over state-of-the-art methods), underlining its notable potential for practical applications in univariate, skewed, long-term time series prediction tasks.
更多查看译文
关键词
deep learning,representation learning,sampling policy,streamflow prediction,time series
AI 理解论文
溯源树
样例
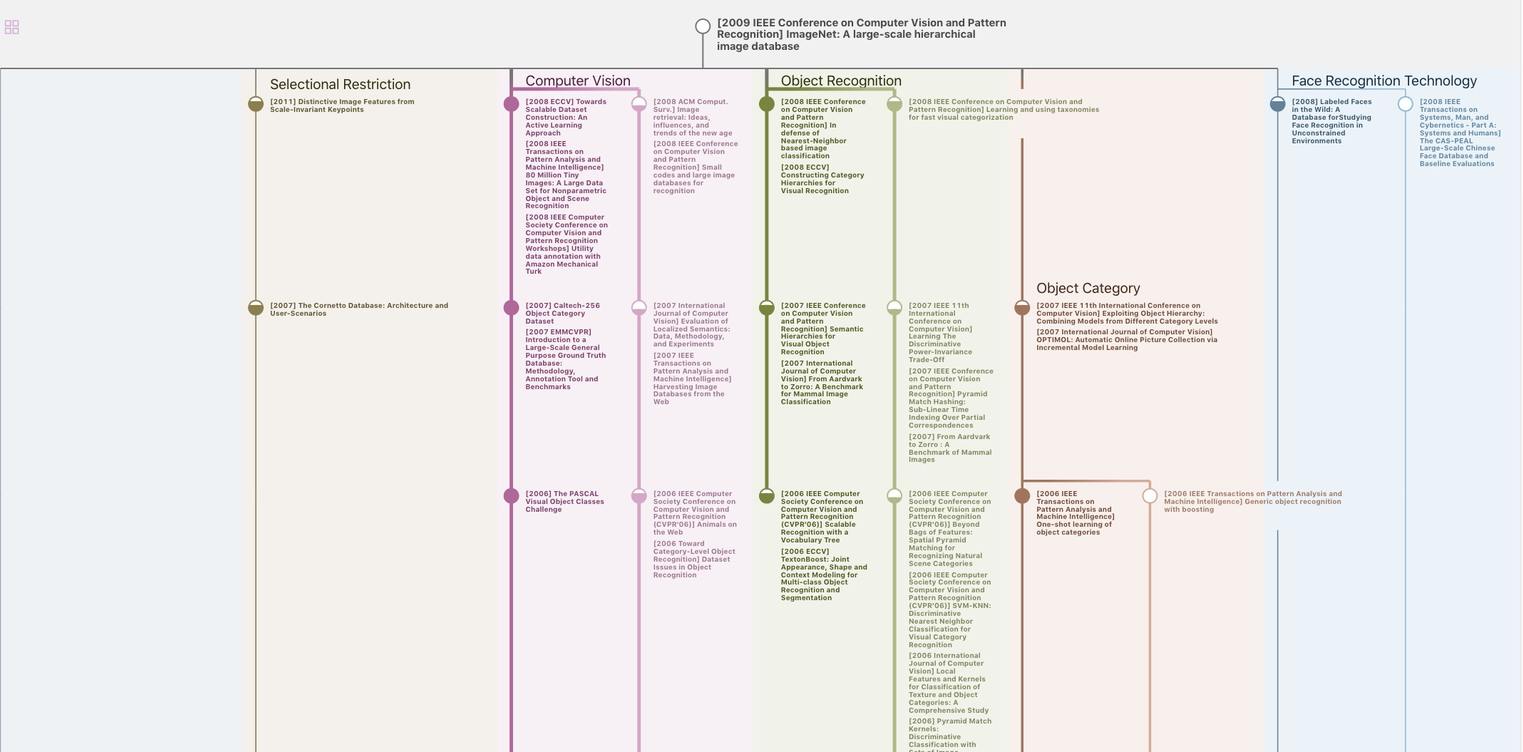
生成溯源树,研究论文发展脉络
Chat Paper
正在生成论文摘要