Scalable Homology Classification through Decomposed Euler Characteristic Curves.
2023 IEEE International Conference on Big Data (BigData)(2023)
摘要
Topological Data Analysis (TDA) has demonstrated notable success in data mining by measuring the presence of topological structure in data. Persistent Homology (PH), one popular tool of TDA, examines the nested sequence of graphs formed over a data filtration to characterize unique homology classes. PH suffers from exponential complexity, limiting the approach to relatively small data sets and lower homology class applications. The Euler Characteristic Curve (ECC), a metric closely related to PH, can be computed more efficiently and, in some cases, can be used as a direct replacement to the applications of PH. A recent technique has been introduced to separate the ECC into dimensional components representing the homology classes identified by PH. This study examines the dimensional ECC, provides an improved algorithm, and introduces interpretation of the results as proximity-series representations of topological features; exploration of how to compare and classify ECC curves is detailed and shown in the context of MRA Brain Artery scan classification. Experimental results with ECC demonstrate the effectiveness and scalability to big data sets beyond that of current persistent homology applications.
更多查看译文
关键词
Topological Data Analysis,Euler Characteristic Curve,Persistent Homology,Discrete Morse Theory,Time-Series Classification
AI 理解论文
溯源树
样例
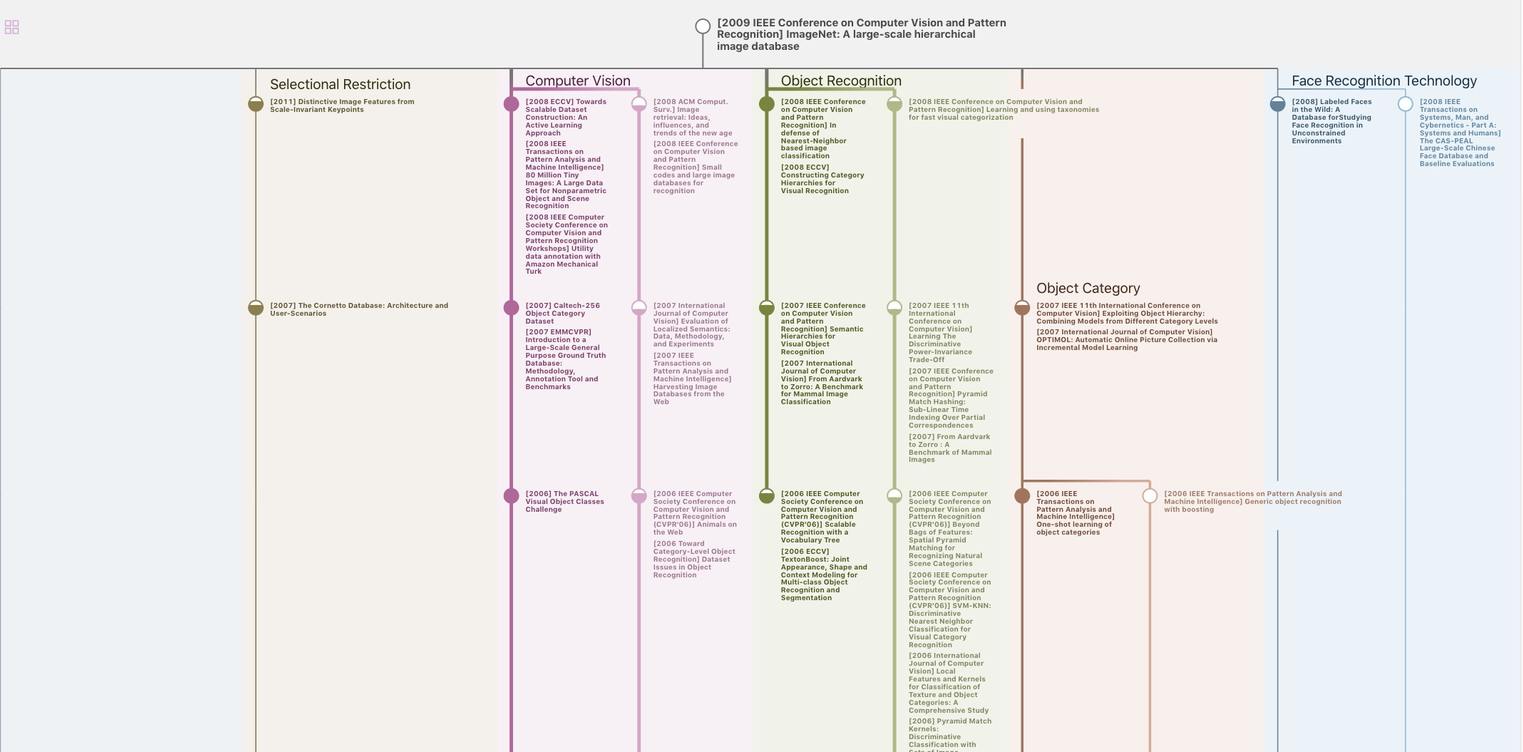
生成溯源树,研究论文发展脉络
Chat Paper
正在生成论文摘要