Neurosymbolic Knowledge Distillation.
2023 IEEE International Conference on Big Data (BigData)(2023)
摘要
The rapid advancement of neural networks has permeated industries worldwide. However, this remarkable success often faces adversity while deploying on devices with limited resources due to the high computational power demands and storage requirements. To address these challenges, a model compressing technique such as knowledge distillation (KD) has emerged as an effective process for training compact models. However, due to the lack of interpretability, knowledge distillation is often hard to explain. In this paper, our focus is to implement a knowledge distillation framework that can provide interpretability alongside improved performance. With the help of our experiments, we demonstrate that this can be achieved by integrating first-order logical formulas into a neurosymbolic learning approach within the knowledge distillation framework. Diverging from the prior research, in this paper, we introduce a Neurosymbolic Knowledge Distillation Framework (KD-LTN), which is a composition of Logic Tensor Network (LTN) and Knowledge Distillation (KD). Notably, our KD-LTN network not only enhances interpretability but also achieves accuracy improvements, compared with the conventional knowledge distillation framework.
更多查看译文
关键词
domain knowledge,knowledge distillation,neurosymbolic,AI,neural networks
AI 理解论文
溯源树
样例
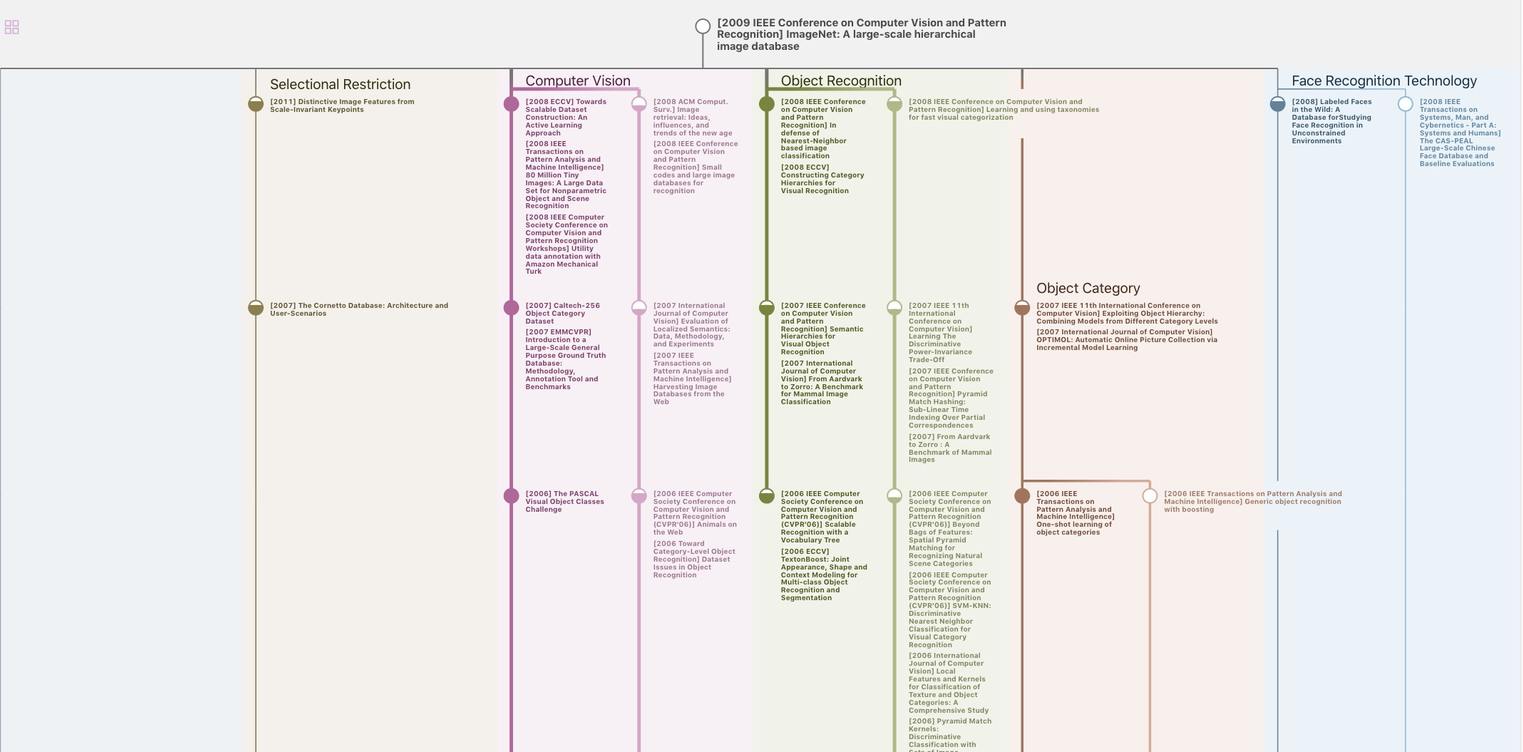
生成溯源树,研究论文发展脉络
Chat Paper
正在生成论文摘要