Traffic Demand Prediction Based on Multi-dimensional Graph Convolutional Network.
2023 IEEE International Conference on Big Data (BigData)(2023)
摘要
Traffic demand prediction is of great importance for traffic management, yet it is also a challenging problem since the traffic data are usually with complex spatial-temporal dependencies and nonlinear relationships. In this paper, to better characterize and utilize the spatial and temporal features, we propose a Multi-dimensional Graph Convolutional Network (M-GCN) to capture dynamic spatial-temporal dependence of traffic data. M-GCN captures the explicit spatio-temporal dependencies by establishing a spatial adjacency graph and a temporal adjacency graph, and captures the hidden spatiotemporal dependencies by establishing a spatial adaptive graph and a temporal adaptive graph. We leverage graph convolutional networks for complex spatial and temporal dependencies modeling and design a gated fusion module to obtain the interactive spatial-temporal dependence. Besides, an attention mechanism is applied to alleviate the error propagation problem in long-term traffic prediction. Experimental results on two real-world traffic datasets demonstrate the superiority of M-GCN. The proposed M-GCN outperforms baseline methods by up to 4.6% improvement in MAE on the dataset TaxiNYC, and the training time and predicting time of $\mathrm{M}-\mathrm{GCN}$ are reduced by nearly half.
更多查看译文
关键词
traffic demand prediction,graph convolutional network,attention mechanism
AI 理解论文
溯源树
样例
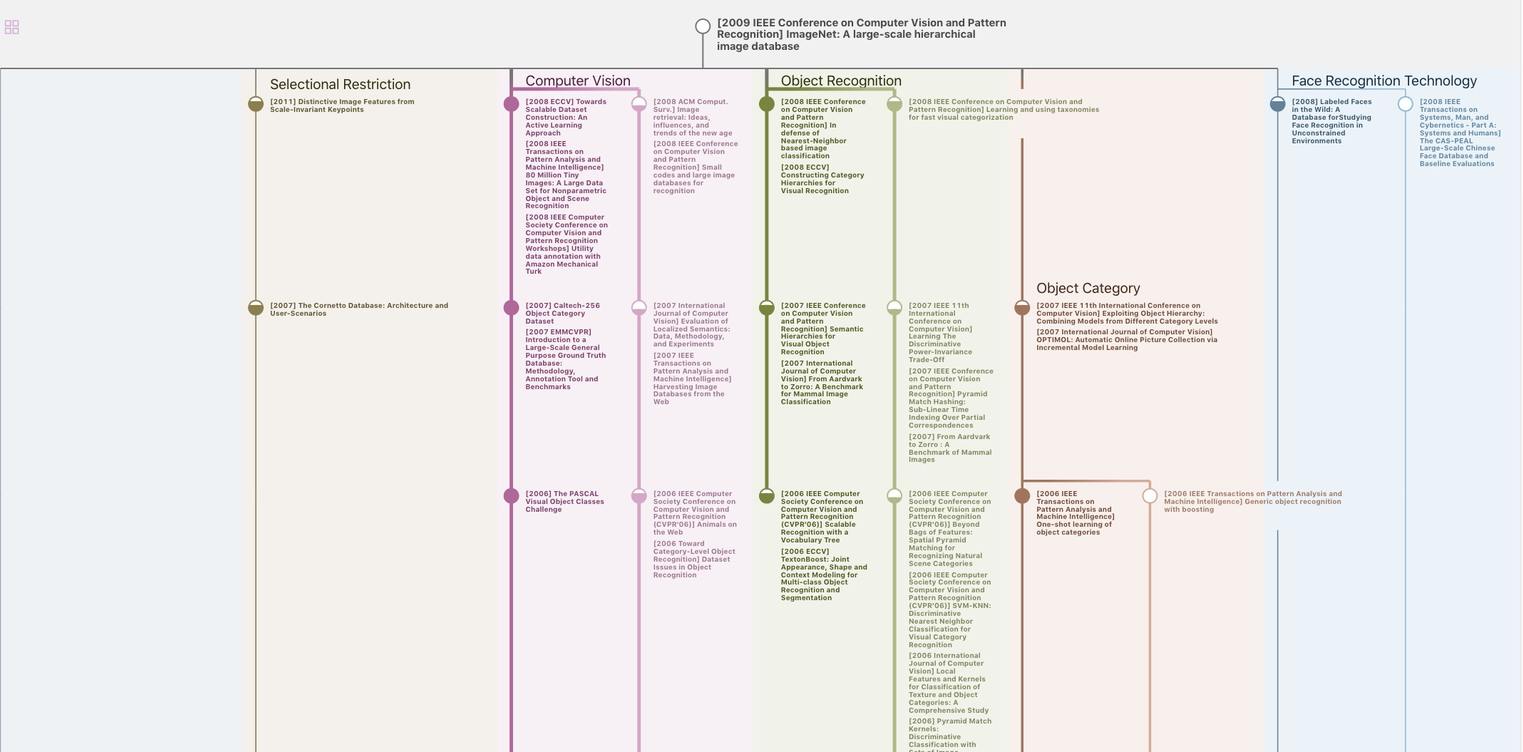
生成溯源树,研究论文发展脉络
Chat Paper
正在生成论文摘要