Towards Better Time-series Data Augmentation for Contrastive Learning.
2023 14th International Conference on Information and Communication Technology Convergence (ICTC)(2023)
摘要
Contrastive learning is now a popular choice for representation learning in various domains, including image and natural language processing. However, contrastive learning for time-series data is relatively limited, due to its unrecognizable, high-dimensional temporal structures. It is still difficult to generate valid augmented views that are semantically accurate, despite the significant research advances in the field of time-series data augmentation. In this work, we survey recent works in time-series contrastive learning and propose a simple augmentation-agnostic technique that can effectively improve the fidelity of the augmented views.
更多查看译文
关键词
Contrastive Learning,Data Augmentation,Time-series,Representation Learning
AI 理解论文
溯源树
样例
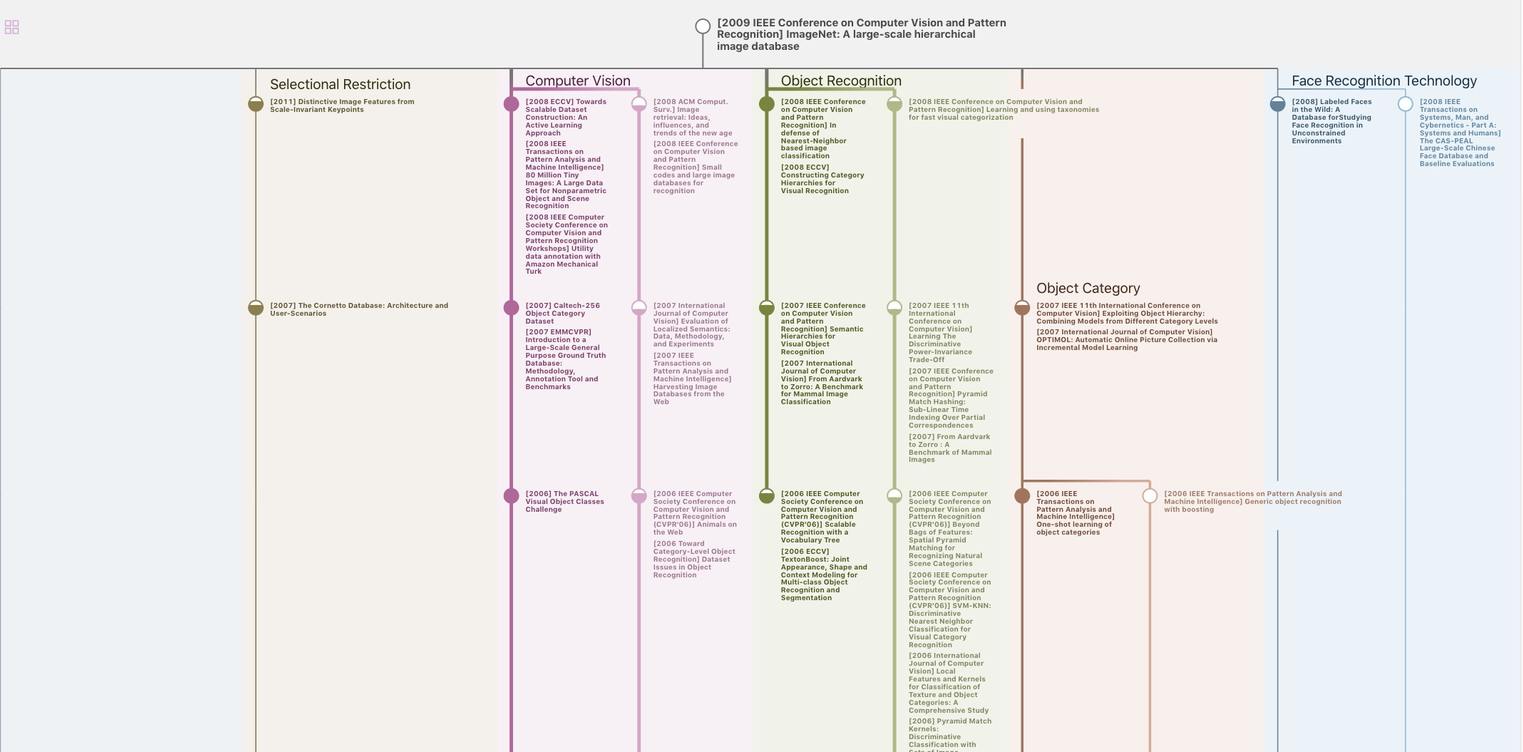
生成溯源树,研究论文发展脉络
Chat Paper
正在生成论文摘要