Development of a Method for Compliance Detection in Wearable Sensors
2023 International Conference on Electrical, Computer and Energy Technologies (ICECET)(2023)
摘要
One of the crucial elements in studies relying on wearable sensors for quantification of human activities (like physical activity or food intake) is the assessment of wear time (compliance). In this paper, we propose a novel method based on the Automatic Ingestion Monitor v2 (AIM-2), deployed for measuring nutrient and energy intake. The proposed method was developed using data from a study of 30 participants for two days each (US dataset) and tested with an independent dataset (Ghana dataset) on 10 households (30 Participants, 3 days for each, a total of 90 days). The signals from the accelerometer sensor of the AIM-2 were used to extract features and train the gradient-boosting tree classifier. To reduce the error in the classification of non-compliance in situations where the sensor changes its position with respect to gravity, a two-stage classifier followed by post-processing was introduced. Previously, we developed an offline compliance classifier, and this work aimed to develop a classifier for a cloud-based feedback system. The accuracy and F1-score of the developed two-phase classifier based on K-fold validation for the training and validation dataset were 95.37% and 96.93%, and for the Ghana dataset, were 95.86% and 92.56%, respectively, showing satisfactory performance results. The trained classifier can be deployed to monitor compliance with device wear in realtime applications. Clinical Relevance– Food Intake and physical activity studies can contribute to detecting, controlling, and even improving eating or physical activity-related problems, like obesity, diabetes, and eating planning. To ensure effective monitoring, compliance with the wearing of the device is crucial.
更多查看译文
关键词
Compliance Detection,Accelerometer Sensor,Embedded Systems,Wearable Sensors
AI 理解论文
溯源树
样例
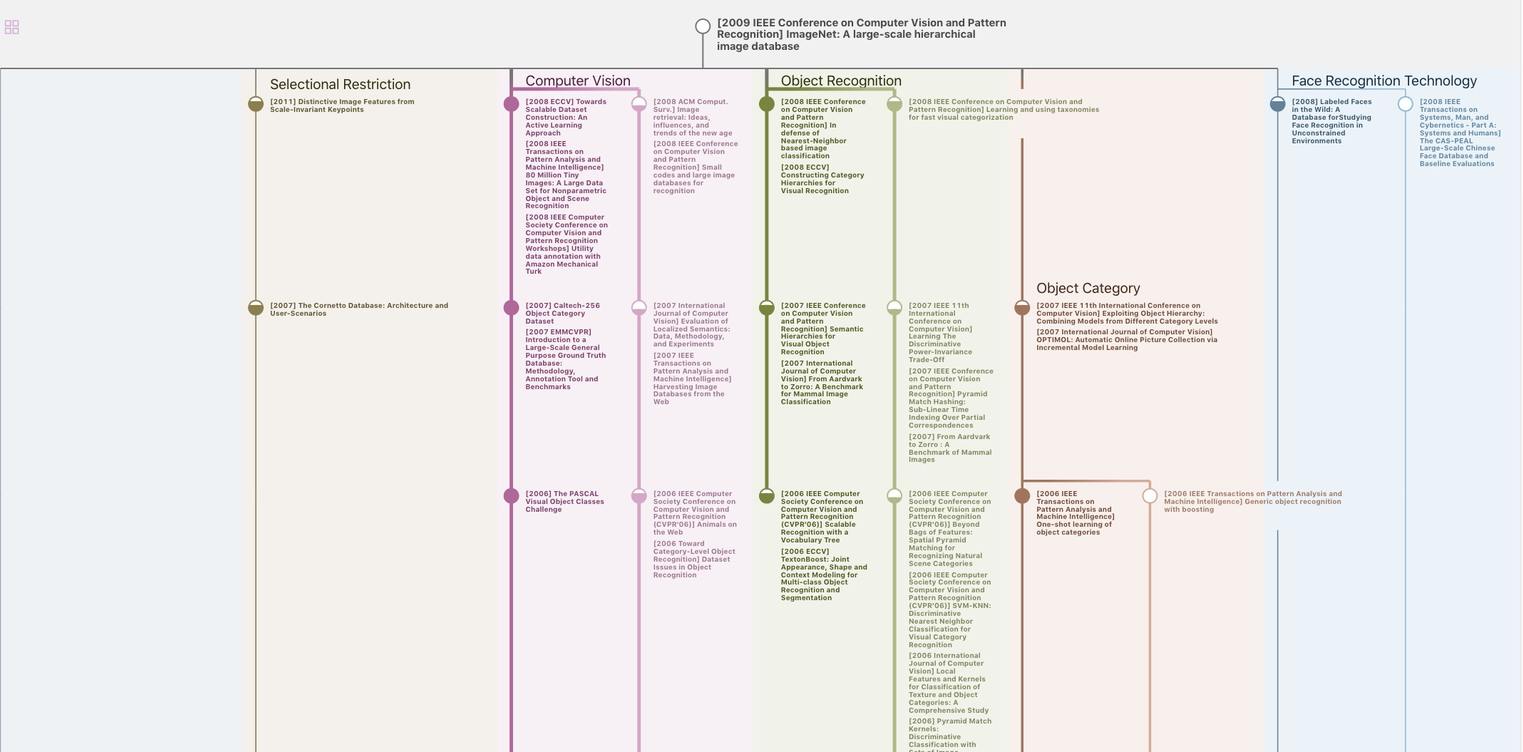
生成溯源树,研究论文发展脉络
Chat Paper
正在生成论文摘要