Subject-specific maximum entropy model of resting state fMRI shows diagnostically distinct patterns of energy state distributions.
bioRxiv : the preprint server for biology(2024)
摘要
Objective:Existing neuroimaging studies of psychotic and mood disorders have reported regional brain activation differences (first-order properties) and alterations in functional connectivity based on pairwise correlations in activation (second-order properties). This study used a generalized Ising model, also called a pairwise maximum entropy model (MEM), to integrate first- and second-order properties to provide a comprehensive picture of BOLD patterns and a system-wide summary measure called energy. This study examines the usefulness of individual level MEMs, attempts to identify image-derived counterparts of the model, and explores potential applications to psychiatry.
Method:MEMs are fit to resting state fMRI data of each individual of a sample of 132 participants consisting of schizophrenia/schizoaffective disorder, bipolar disorder, and major depression, and a demographically matched 132 participants without these diagnoses from the UK Biobank to examine the default mode network (DMN).
Results:The model explained observed brain state occurrence probabilities well across all participants, and model parameters were highly correlated to image-derived parameters for all groups. Within clinical groups, schizophrenia/schizoaffective disorder and bipolar disorder patients showed significant differences in averaged energy distribution compared to controls for all sub-systems of the DMN except for depression, where differences in the energy distributions were only detected in the DMN of the regions from the right hemisphere.
Conclusions:Subject-specific Ising modeling may offer an improved measure of biological functional correlates relative to traditional approaches. The observation of distinct patterns of energy distribution among the three clinical groups compared to controls suggests relative diagnostic specificity and potential for clinical translation.
更多查看译文
AI 理解论文
溯源树
样例
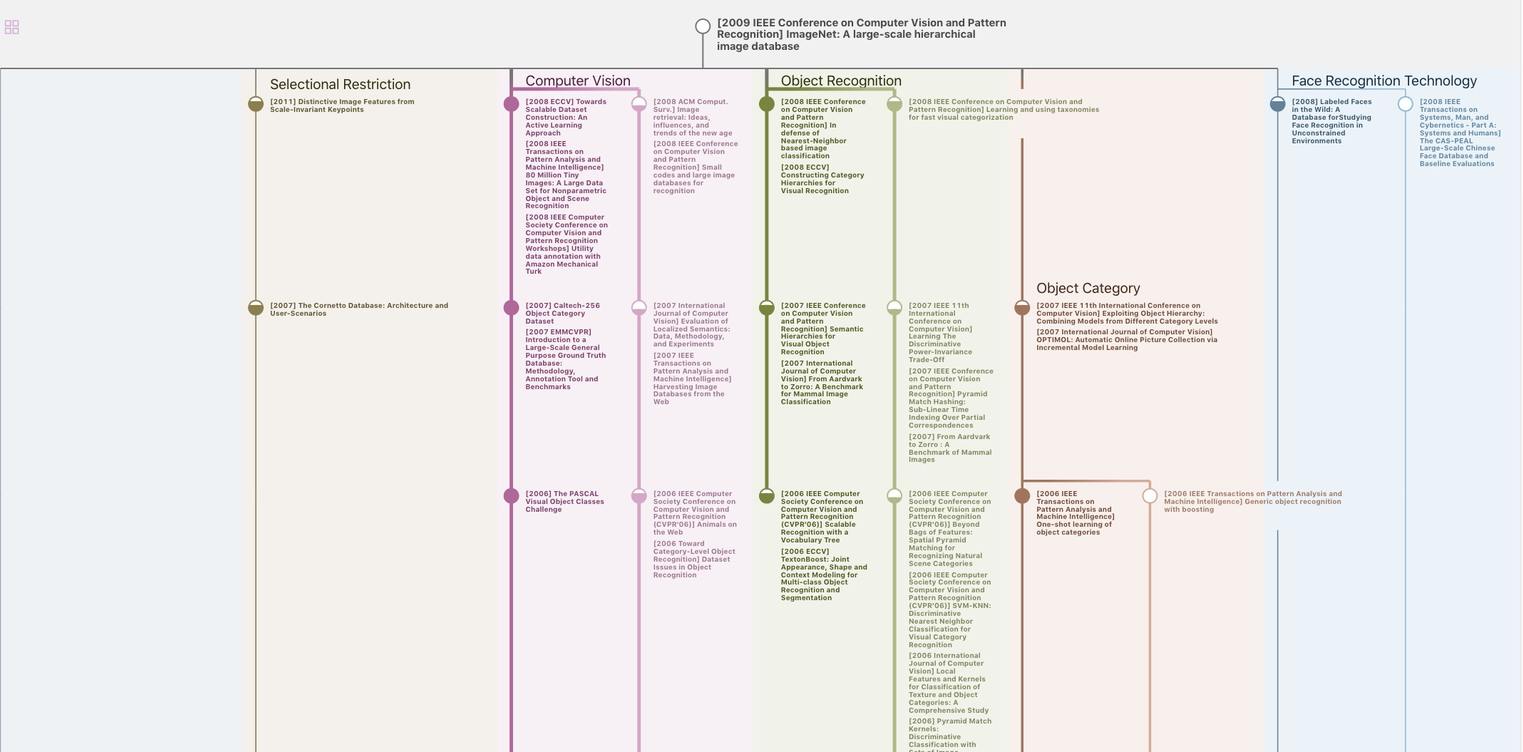
生成溯源树,研究论文发展脉络
Chat Paper
正在生成论文摘要