Winding Clearness for Differentiable Point Cloud Optimization
CoRR(2024)
摘要
We propose to explore the properties of raw point clouds through the
winding clearness, a concept we first introduce for assessing the
clarity of the interior/exterior relationships represented by the winding
number field of the point cloud. In geometric modeling, the winding number is a
powerful tool for distinguishing the interior and exterior of a given surface
∂Ω, and it has been previously used for point normal orientation
and surface reconstruction. In this work, we introduce a novel approach to
assess and optimize the quality of point clouds based on the winding clearness.
We observe that point clouds with reduced noise tend to exhibit improved
winding clearness. Accordingly, we propose an objective function that
quantifies the error in winding clearness, solely utilizing the positions of
the point clouds. Moreover, we demonstrate that the winding clearness error is
differentiable and can serve as a loss function in optimization-based and
learning-based point cloud processing. In the optimization-based method, the
loss function is directly back-propagated to update the point positions,
resulting in an overall improvement of the point cloud. In the learning-based
method, we incorporate the winding clearness as a geometric constraint in the
diffusion-based 3D generative model. Experimental results demonstrate the
effectiveness of optimizing the winding clearness in enhancing the quality of
the point clouds. Our method exhibits superior performance in handling noisy
point clouds with thin structures, highlighting the benefits of the global
perspective enabled by the winding number.
更多查看译文
AI 理解论文
溯源树
样例
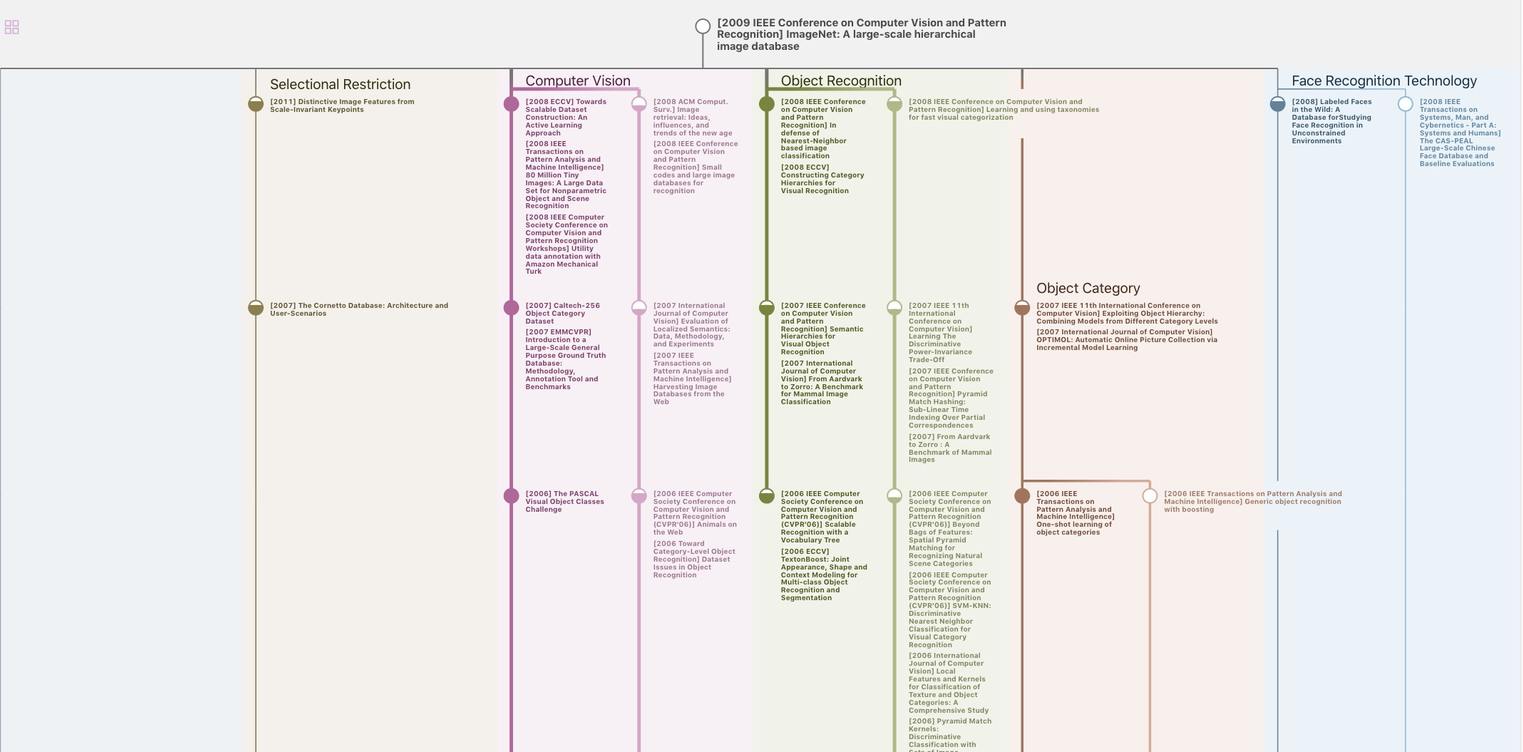
生成溯源树,研究论文发展脉络
Chat Paper
正在生成论文摘要