SpeechDPR: End-to-End Spoken Passage Retrieval for Open-Domain Spoken Question Answering
CoRR(2024)
摘要
Spoken Question Answering (SQA) is essential for machines to reply to user's
question by finding the answer span within a given spoken passage. SQA has been
previously achieved without ASR to avoid recognition errors and
Out-of-Vocabulary (OOV) problems. However, the real-world problem of
Open-domain SQA (openSQA), in which the machine needs to first retrieve
passages that possibly contain the answer from a spoken archive in addition,
was never considered. This paper proposes the first known end-to-end framework,
Speech Dense Passage Retriever (SpeechDPR), for the retrieval component of the
openSQA problem. SpeechDPR learns a sentence-level semantic representation by
distilling knowledge from the cascading model of unsupervised ASR (UASR) and
text dense retriever (TDR). No manually transcribed speech data is needed.
Initial experiments showed performance comparable to the cascading model of
UASR and TDR, and significantly better when UASR was poor, verifying this
approach is more robust to speech recognition errors.
更多查看译文
关键词
Spoken Question Answering,Spoken Language Understanding,Spoken Content Retrieval
AI 理解论文
溯源树
样例
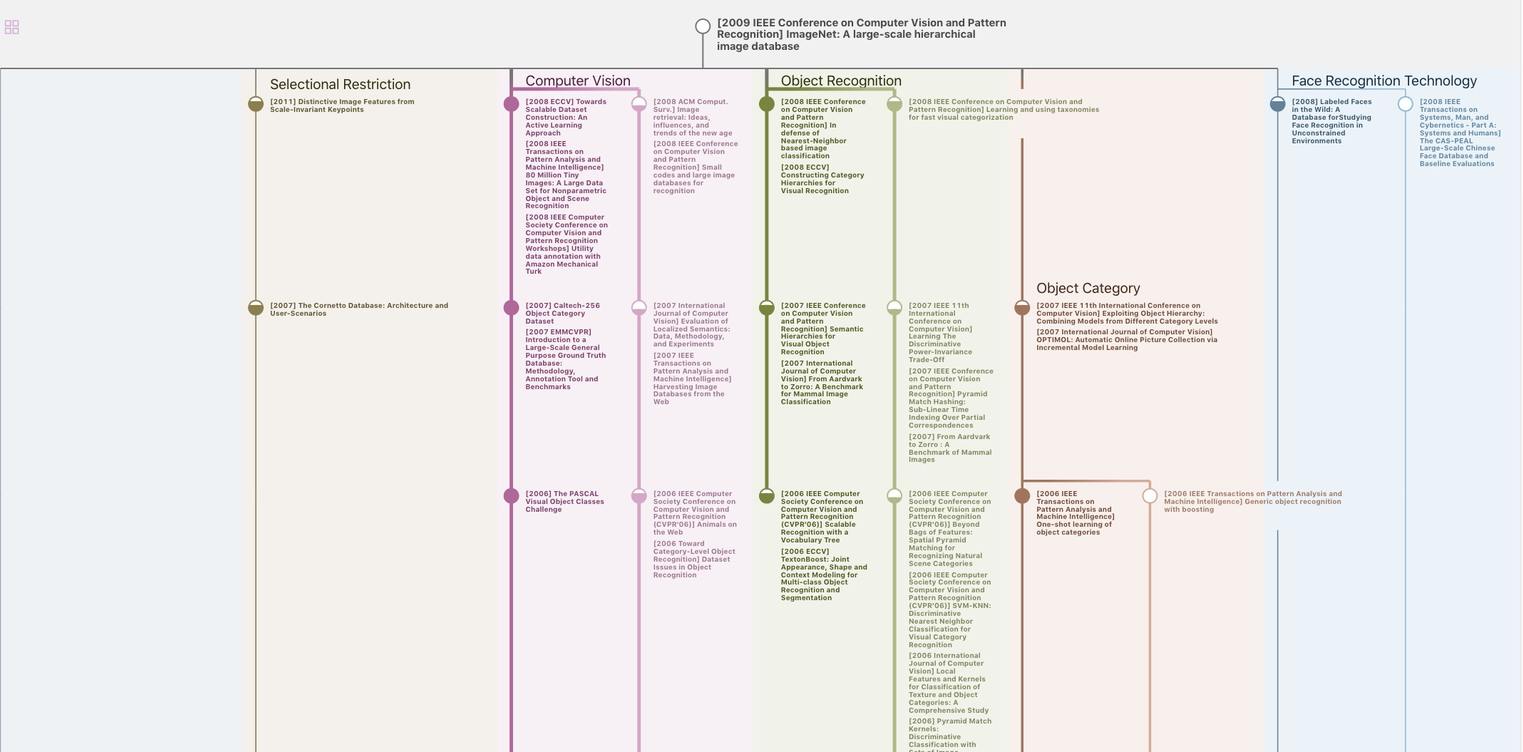
生成溯源树,研究论文发展脉络
Chat Paper
正在生成论文摘要