An explainable framework for cross-platform DNA methylation-based classification of cancer
medrxiv(2024)
摘要
DNA methylation-based classification of brain tumors has emerged as a powerful and indispensable diagnostic technique. Initial implementations have used methylation microarrays for data generation, but different sequencing approaches are increasingly used. Most current classifiers, however, rely on a fixed methylation feature space, rendering them incompatible with other platforms, especially different flavors of DNA sequencing. Here, we describe crossNN, a neural network-based machine learning framework which can accurately classify tumor entities using DNA methylation profiles obtained from different platforms and with different epigenome coverage and sequencing depth. It outperforms other deep- and shallow machine learning models with respect to precision as well as simplicity and computational requirements while still being fully explainable. Validation in a large cohort of >1,900 tumors profiled using different microarray and sequencing platforms, including low-pass nanopore and targeted bisulfite sequencing, demonstrates the robustness and scalability of the model.
### Competing Interest Statement
The authors have declared no competing interest.
### Funding Statement
This study was funded by The Brain Tumour Charity, UK, grant no. GN-000694.
### Author Declarations
I confirm all relevant ethical guidelines have been followed, and any necessary IRB and/or ethics committee approvals have been obtained.
Yes
The details of the IRB/oversight body that provided approval or exemption for the research described are given below:
The local ethics committee (Charite Universitaetsmedizin Berlin, Berlin, Germany; EA2/041/18) approved generation of prospective data in the context of this study. All necessary patient/participant consent has been obtained and the appropriate institutional forms have been archived.
I confirm that all necessary patient/participant consent has been obtained and the appropriate institutional forms have been archived, and that any patient/participant/sample identifiers included were not known to anyone (e.g., hospital staff, patients or participants themselves) outside the research group so cannot be used to identify individuals.
Yes
I understand that all clinical trials and any other prospective interventional studies must be registered with an ICMJE-approved registry, such as ClinicalTrials.gov. I confirm that any such study reported in the manuscript has been registered and the trial registration ID is provided (note: if posting a prospective study registered retrospectively, please provide a statement in the trial ID field explaining why the study was not registered in advance).
Yes
I have followed all appropriate research reporting guidelines, such as any relevant EQUATOR Network research reporting checklist(s) and other pertinent material, if applicable.
Yes
All data produced in the present study are available upon reasonable request to the authors
更多查看译文
AI 理解论文
溯源树
样例
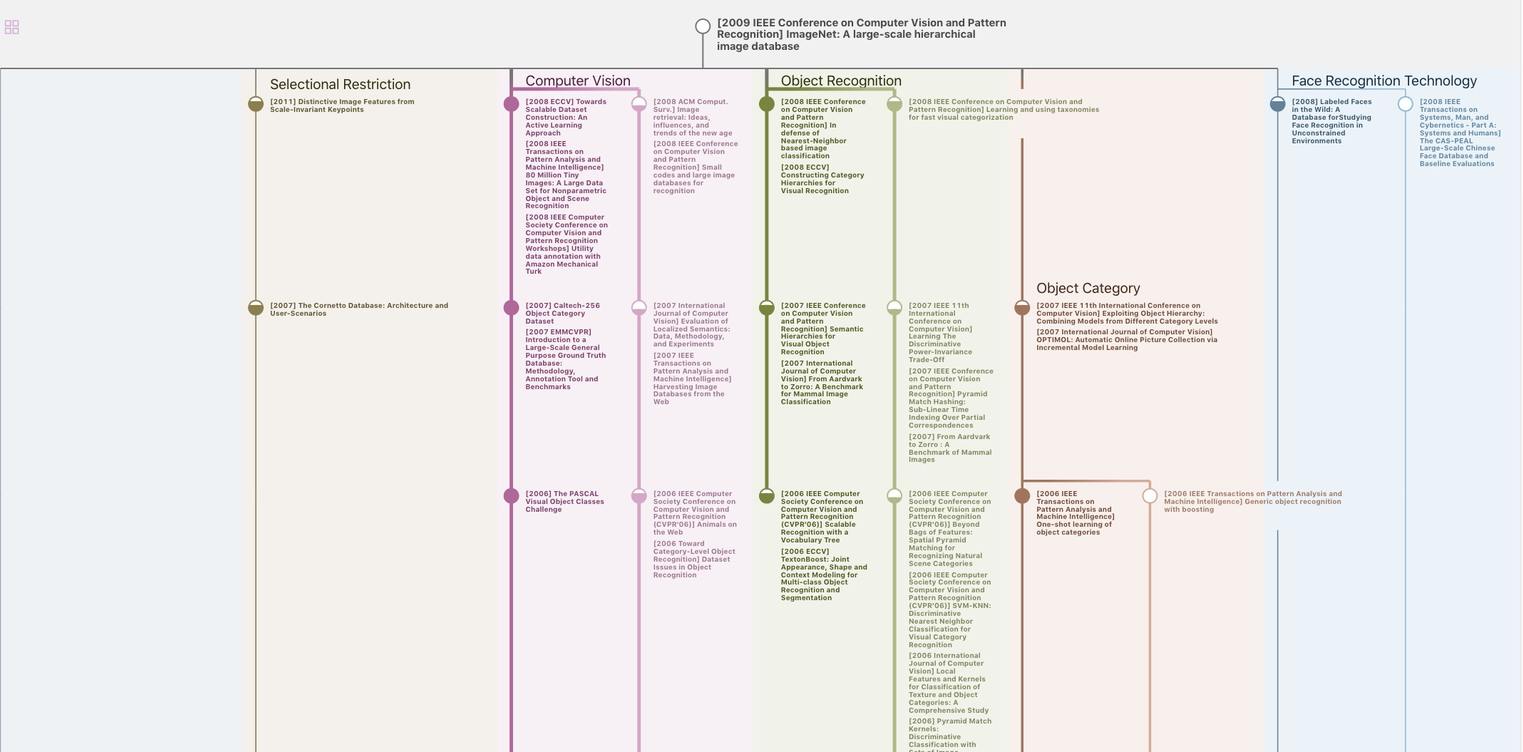
生成溯源树,研究论文发展脉络
Chat Paper
正在生成论文摘要