Multi-Objective Battery Charging Strategy Based on Deep Reinforcement Learning
IEEE Transactions on Transportation Electrification(2023)
摘要
The trade-off between charging duration and battery overheating is a critical issue in battery charging, which is essentially a multi-objective decision problem. In this paper, we propose a battery charging strategy based on deep reinforcement learning. In contrast to conventional methods, reinforcement learning technology empowers our approach to adapt to dynamic environments readily. Furthermore, strategies with various preferences are generated through a single training process. This marks the pioneering attempt to address the intricate multi-objective problem using the innovative approach of multi-objective deep reinforcement learning. Initially, the electrochemical-thermal coupled battery model was developed. The parameters of the electrochemical model are determined based on the metric data provided by the device provider, and the differential evolution algorithm is utilized to determine the parameters of the thermal model. The experiment data then validate the constructed model. Subsequently, the battery charging problem is formulated as a multi-objective Markov Decision Process. Finally, the multi-objective reinforcement learning algorithm is proposed to solve the formulated problem. Through learning, the agent can acquire an approximate Pareto front representing the trade-off between charging duration and battery overheating. Therefore, a set of feasible strategies with various preferences are raised. At last, the experiments are conducted to verify the efficiency of the proposed method.
更多查看译文
关键词
Lithium-ion battery,charging strategy,multi-objective optimization,deep reinforcement learning,Pareto front
AI 理解论文
溯源树
样例
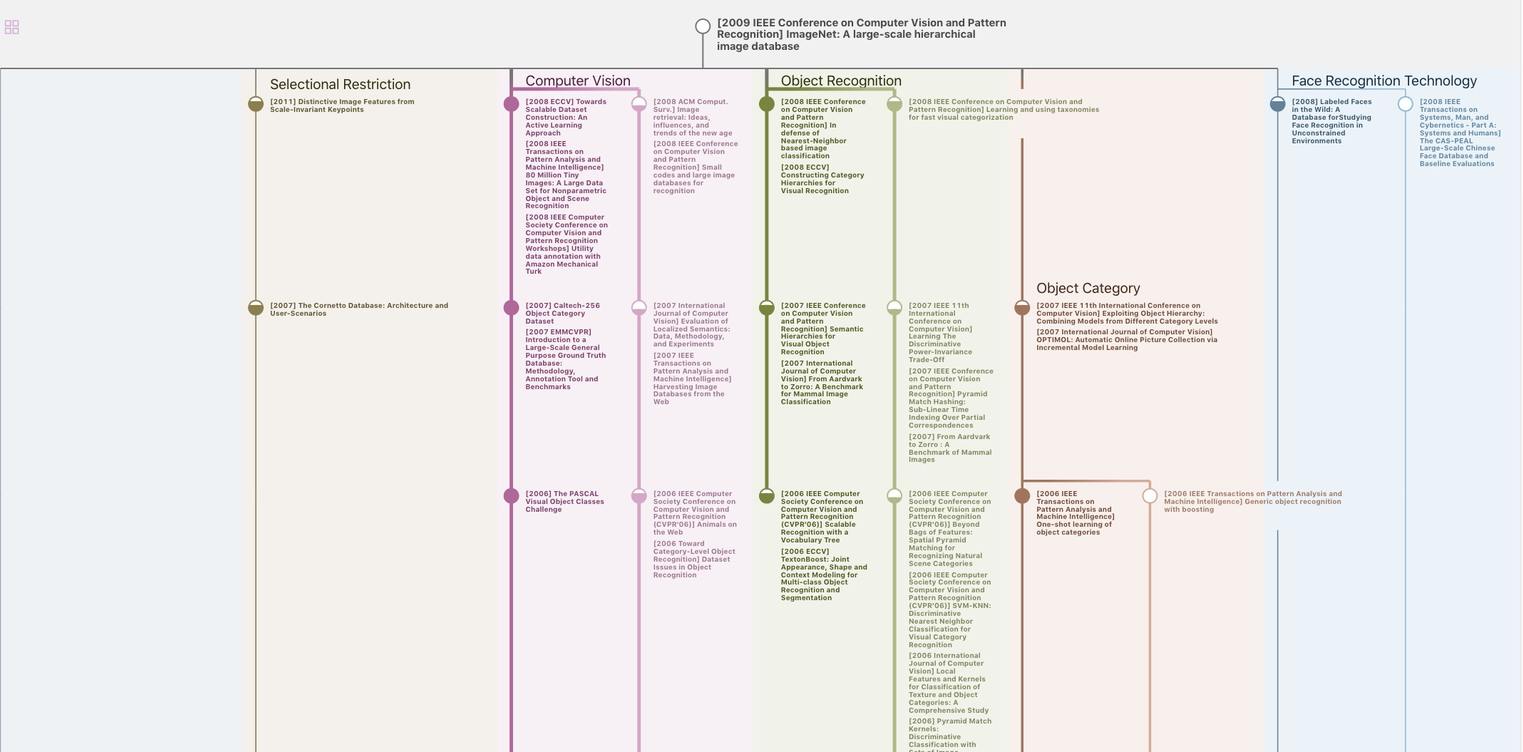
生成溯源树,研究论文发展脉络
Chat Paper
正在生成论文摘要