Domain Mapping Network for Remote Sensing Cross-Domain Few-Shot Classification
IEEE TRANSACTIONS ON GEOSCIENCE AND REMOTE SENSING(2024)
摘要
It is a challenging task to recognize novel categories with only a few labeled remote-sensing images. Currently, meta-learning solves the problem by learning prior knowledge from another dataset where the classes are disjoint. However, the existing methods assume the training dataset comes from the same domain as the test dataset. For remote-sensing images, test datasets may come from different domains. It is impossible to collect a training dataset for each domain. Meta-learning and transfer learning are widely used to tackle the few-shot classification and the cross-domain classification, respectively. However, it is difficult to recognize novel categories from various domains with only a few images. In this article, a domain mapping network (DMN) is proposed to cope with the few-shot classification under domain shift. DMN trains an efficient few-shot classification model on the source domain and then adapts the model to the target domain. Specifically, dual autoencoders are exploited to fit the source and target domain distribution. First, DMN learns an autoencoder on the source domain to fit the source domain distribution. Then, a target autoencoder is initiated from the source domain autoencoder and further updated with a few target images. To ensure the distribution alignment, cycle-consistency losses are proposed to jointly train the source autoencoder and target autoencoder. Extensive experiments are conducted to validate the generalizable and superiority of the proposed method.
更多查看译文
关键词
Training,Task analysis,Adaptation models,Remote sensing,Image recognition,Metalearning,Measurement,Cross-domain classification,few-shot classification,meta-learning,remote sensing scene classification,transfer learning
AI 理解论文
溯源树
样例
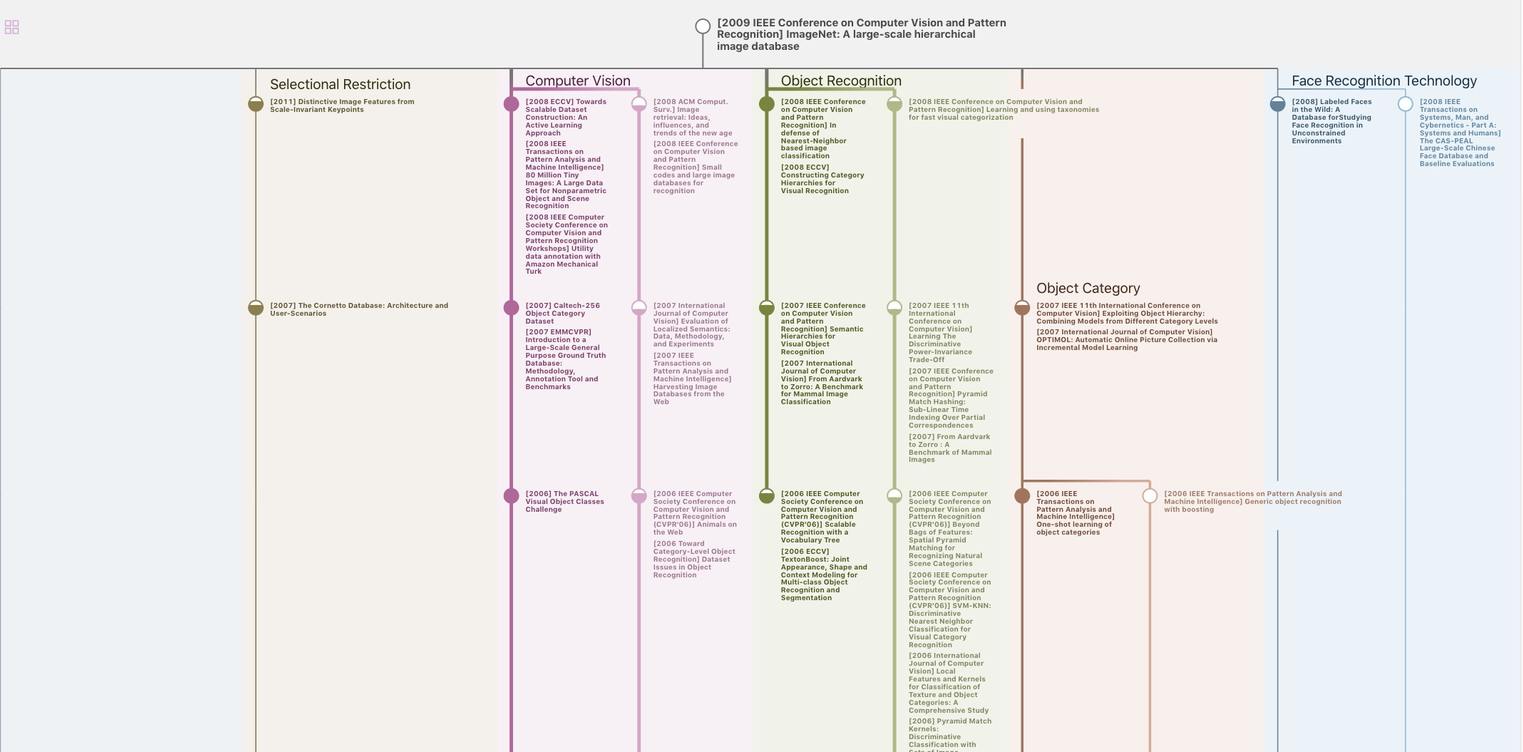
生成溯源树,研究论文发展脉络
Chat Paper
正在生成论文摘要