Physics-Driven Deep Learning Pixel-Based Inversion of Logging While Drilling in Anisotropic Formation
IEEE TRANSACTIONS ON GEOSCIENCE AND REMOTE SENSING(2024)
摘要
The inversion of logging-while-drilling (LWD) measurements using deep learning (DL) (data-driven approach) is a rapidly growing topic of interest in well geosteering. DL inversion constructs a complex nonlinear mapping from data to model and has extremely high inversion efficiency. A purely data-driven approach requires large amounts of representative training samples from both the model and data spaces to build robust networks, which may not conform to the physical constraints of the problem. The lack of physical knowledge can limit the effectiveness of DL networks when applied to new scenarios. The regularization inversion (physics-driven approach) is a very efficient local optimization technique but is prone to be trapped into local minima, and the inversion results cannot be obtained in real time. We propose a coupled physics-driven and data-driven approach to address this issue and construct a DL workflow. A 2.5-D model, including dip, fault, and anisotropic formation, is considered to evaluate the proposed method. Comparing the inversion imaging performance of the proposed physics-driven approach with the traditional residual network (ResNet) shows a significant improvement in the accuracy of the reconstructed resistivity model. Finally, the robustness is evaluated by adding a noise generalization network.
更多查看译文
关键词
Training,Neural networks,Transmitters,Data models,Receivers,Instruments,Conductivity,Deep learning (DL),logging while drilling (LWD),physics-driven approach,pixel-based inversion
AI 理解论文
溯源树
样例
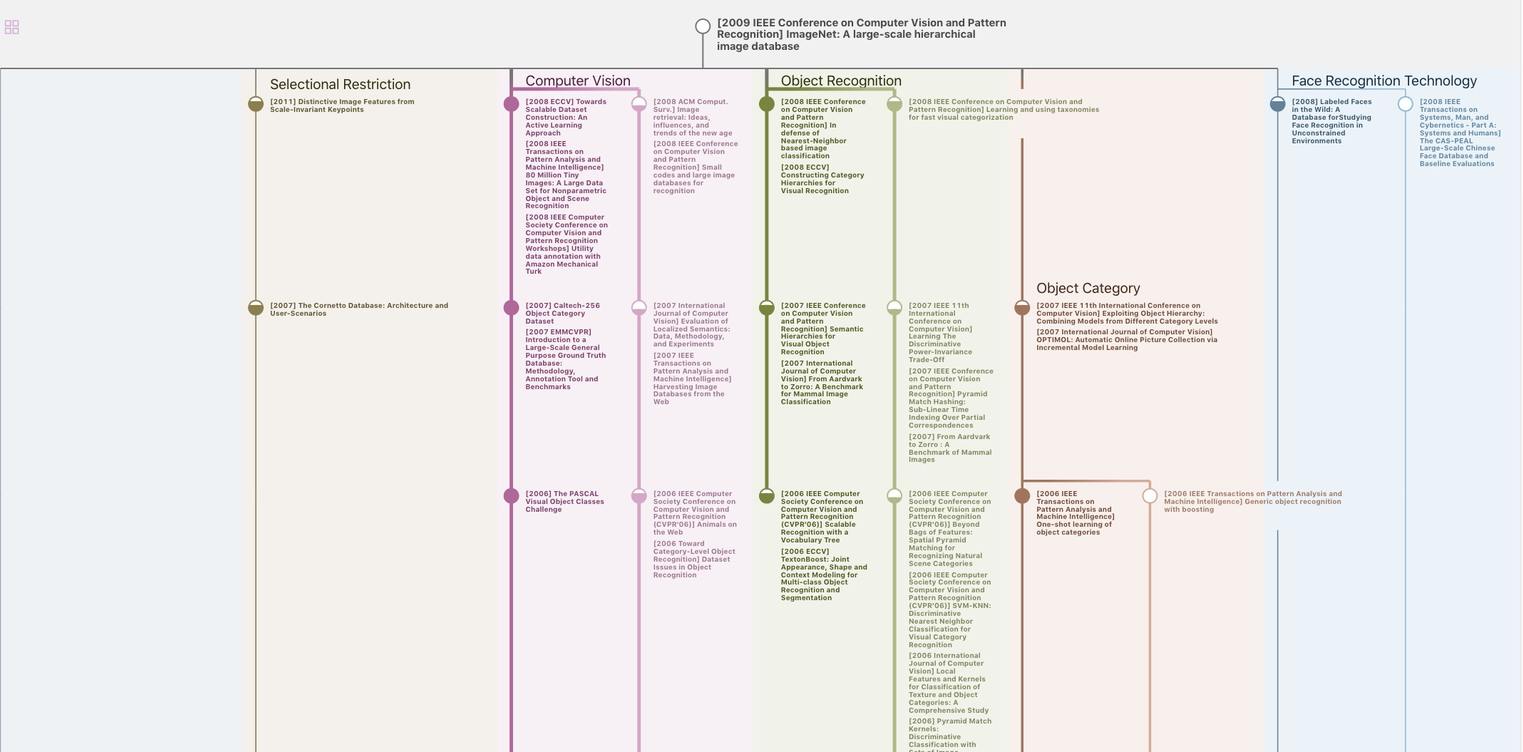
生成溯源树,研究论文发展脉络
Chat Paper
正在生成论文摘要