Advancing Realistic Precipitation Nowcasting With a Spatiotemporal Transformer-Based Denoising Diffusion Model
IEEE TRANSACTIONS ON GEOSCIENCE AND REMOTE SENSING(2024)
摘要
Recent advances in deep learning (DL) have significantly improved the quality of precipitation nowcasting. Current approaches are either based on deterministic or generative models. Deterministic models perceive nowcasting as a spatiotemporal prediction task, relying on distance functions like $L2$ -norm loss for training. While improving meteorological evaluation metrics, they inevitably produce blurry predictions with no reference value. In contrast, generative models aim to capture realistic precipitation distributions and generate nowcasting products by sampling within these distributions. However, designing a generative model that produces realistic samples satisfying meteorological evaluation indexes in real-time remains challenging, given the triple dilemma of generative learning: achieving high sample quality, mode coverage, and fast sampling simultaneously. Recently, diffusion models exhibit impressive sample quality but suffer from time-consuming sampling, severely hindering their application in nowcasting. Moreover, samples generated by the U-Net denoiser of the current denoising diffusion model are prone to yield poor meteorological evaluation metrics such as CSI. To this end, we propose a spatiotemporal transformer-based conditional diffusion model with a rapid diffusion strategy. Concretely, we incorporate an adversarial mapping-based rapid diffusion strategy to overcome the time-consuming sampling process for standard diffusion models, enabling timely nowcasting. In addition, a meticulously designed spatiotemporal transformer-based denoiser is incorporated into diffusion models, remedying the defects in U-Net denoisers by estimating diffusion scores and improving nowcasting skill scores. Case studies of typical weather events such as thunderstorms, as well as quantitative indicators, demonstrate the effectiveness of the proposed method in generating sharper and more precise precipitation forecasts while maintaining satisfied meteorological evaluation metrics.
更多查看译文
关键词
Precipitation,Noise reduction,Predictive models,Spatiotemporal phenomena,Radar,Radar imaging,Measurement,Backbone design for diffusion models,conditional denoising diffusion model,generative learning,nowcasting
AI 理解论文
溯源树
样例
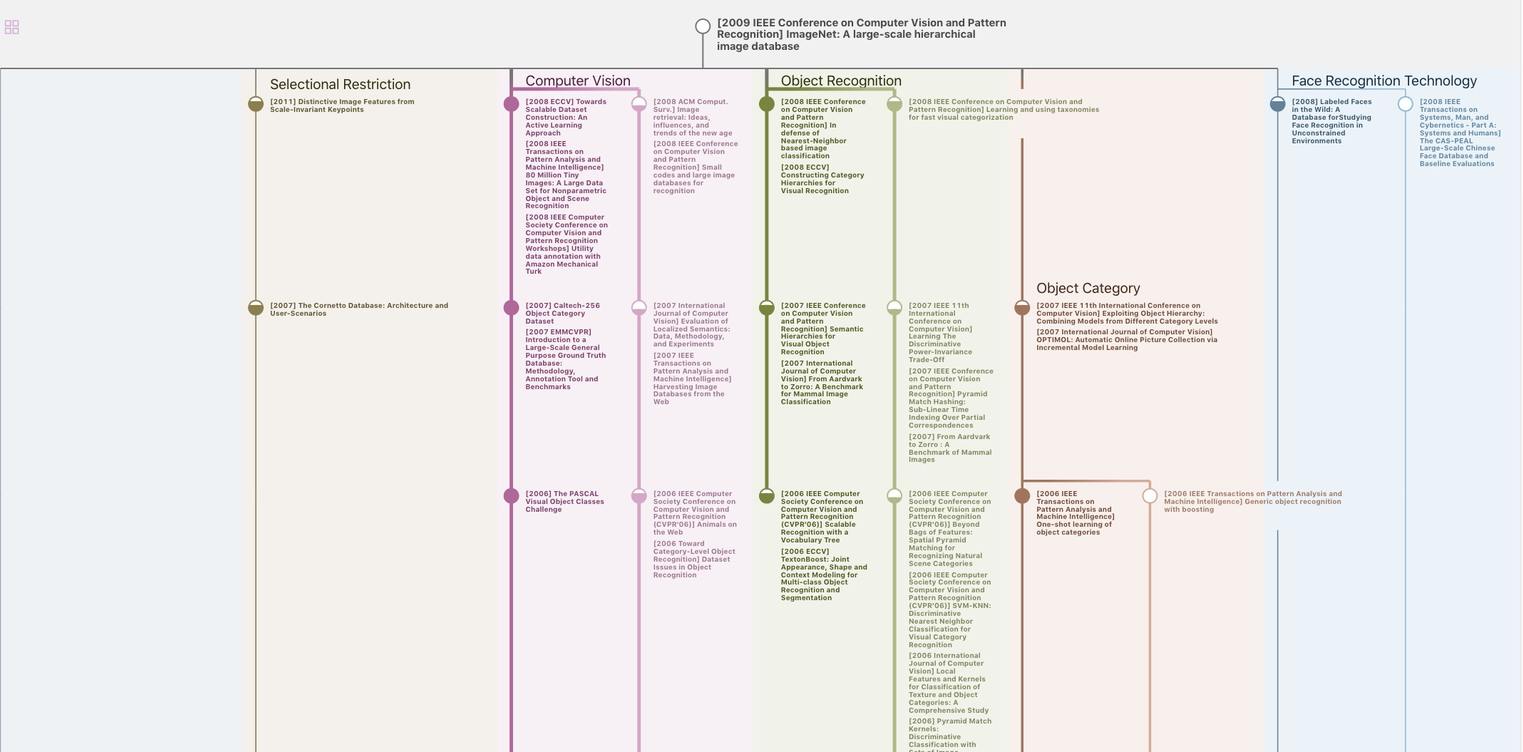
生成溯源树,研究论文发展脉络
Chat Paper
正在生成论文摘要