Transfer Learning of Spatial Features From High-Resolution RGB Images for Large-Scale and Robust Hyperspectral Remote Sensing Target Detection
IEEE TRANSACTIONS ON GEOSCIENCE AND REMOTE SENSING(2024)
摘要
Target detection is a critical task in interpreting hyperspectral remote sensing images. Small target (such as airplanes) detection is challenging, especially in large-scale complex scenes with high spectral variability of different land cover types. In this article, we propose a transfer learning-based, large-scale, robust hyperspectral target detector ((TLHTD)-T-2) to improve the accuracy of hyperspectral target detection (HTD) in large-scale complex scenes. (TLHTD)-T-2 learns the spatial features of hyperspectral targets from high-resolution remote sensing images and achieves high-precision HTD with fused spatial-spectral features. It comprises three parts. First, the coupled target-background sample expansion (CTBSE) module is designed to expand the labeled hyperspectral target and background samples with sufficient high-resolution, labeled RGB images, and a few labeled hyperspectral samples. Second, the hard positive and negative example mining (HPNEM) module trains the hard positive and negative samples to enhance the discriminative ability of the network, addressing the problem of inadequate sample training in large-scale hyperspectral images (HSIs). Third, the spatial-spectral weighted subspace (SSWS) module is designed to fuse the spatial features extracted from the target detection network and the spectral features based on the Mahalanobis distance. The results show that: 1) the (TLHTD)-T-2 achieves average area under the curve (AUC) values of 0.96, 0.96, and 0.93 on small-, medium-, and large-sized HSIs, respectively, achieving the highest accuracy compared with other HTD algorithms; 2) the (TLHTD)-T-2 exhibits the highest detection time efficiency for medium- and large-sized HSIs; and 3) for large-sized HSIs, when most HTD algorithms fail, (TLHTD)-T-2 exhibits significantly higher accuracy and time efficiency than other methods. This is an important achievement in meeting the robust target detection tasks of large-scale hyperspectral remote sensing images.
更多查看译文
关键词
Hyperspectral imaging,Feature extraction,Spatial resolution,Object detection,Training,Transfer learning,Satellites,Hyperspectral images (HSIs),hyperspectral target detection (HTD),large-scale complex scenes,spatial-spectral feature fusion,transfer learning
AI 理解论文
溯源树
样例
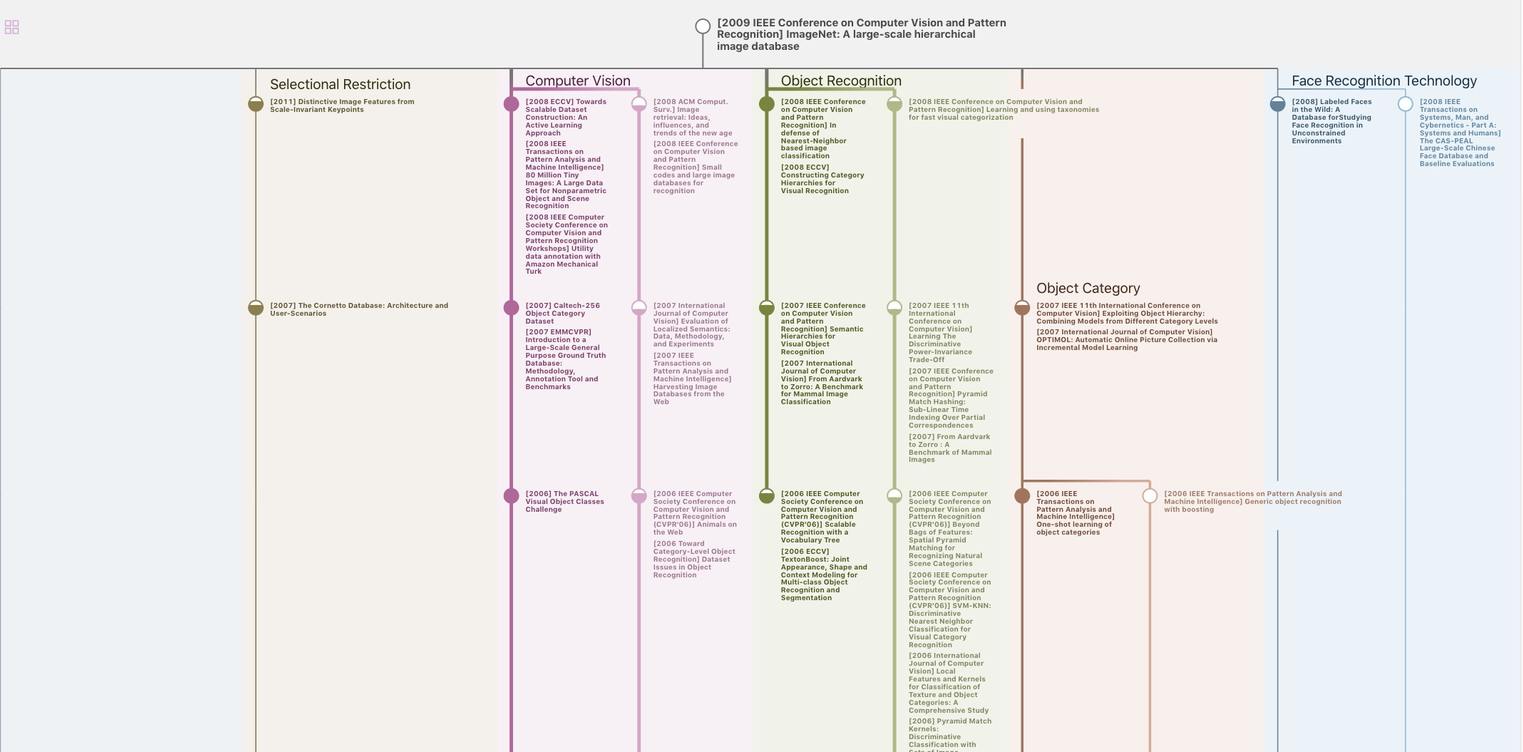
生成溯源树,研究论文发展脉络
Chat Paper
正在生成论文摘要