Dynamic Quantification with Constrained Error Under Unknown General Dataset Shift
IEEE Transactions on Knowledge and Data Engineering(2024)
摘要
Quantification research has sought to accurately estimate class distributions under dataset shift. While existing methods perform well under assumed conditions of shift, it is not always clear whether such assumptions will hold in a given application. This work extends the analysis and experimental evaluation of our Gain-Some-Lose-Some (GSLS) model for quantification under general dataset shift and incorporates it into a method for dynamically selecting the most appropriate quantification method. Selection by a Kolmogorov-Smirnov test for any shift followed by a newly proposed “Adjusted Kolmogorov-Smirnov” test for non-prior shift is found to best balance quantification and runtime performance. We also present a framework for constraining quantification prediction intervals to user-specified limits by requesting a smaller set of instance class labels from the user than required with confidence-based rejection.
更多查看译文
关键词
Quantification,dataset shift,prediction intervals,shift detection,machine learning
AI 理解论文
溯源树
样例
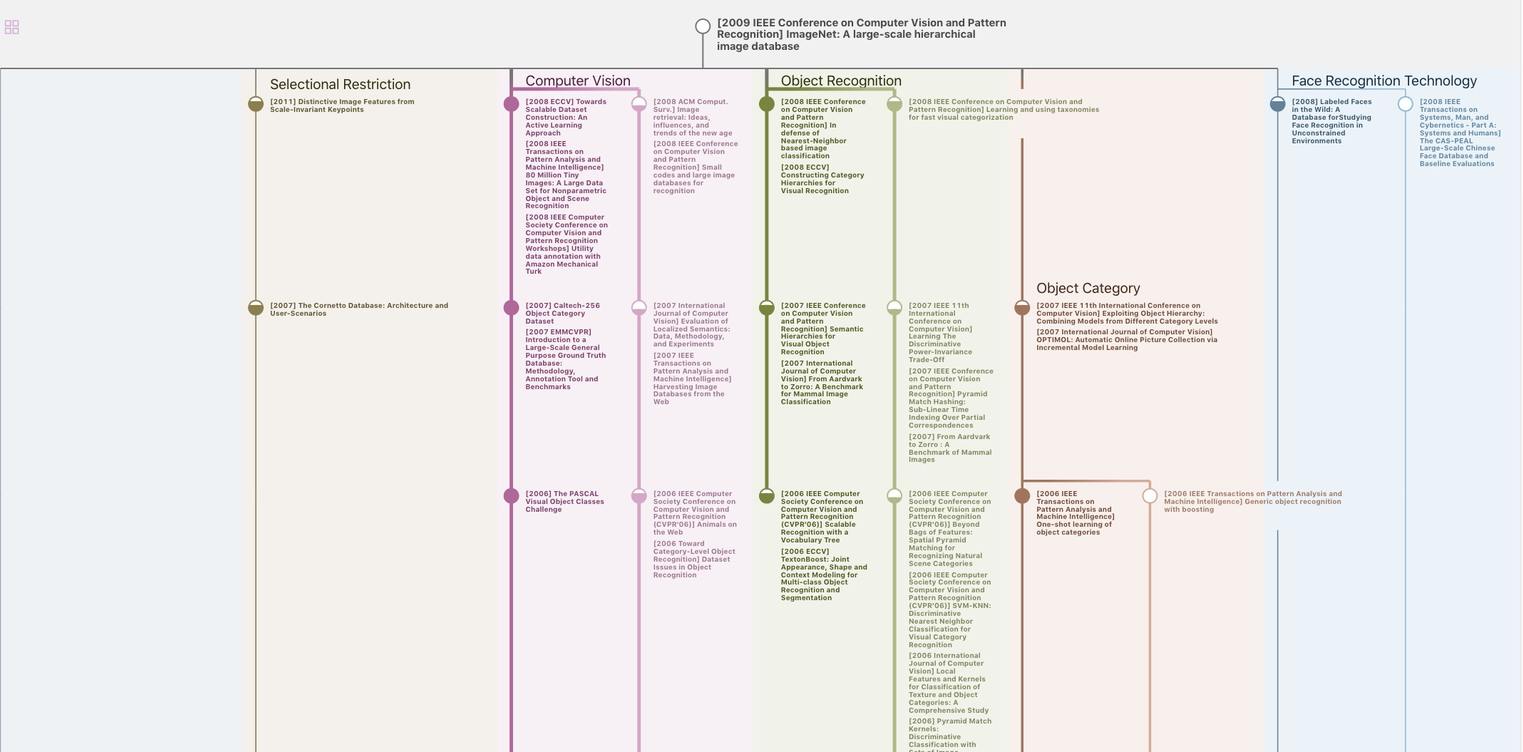
生成溯源树,研究论文发展脉络
Chat Paper
正在生成论文摘要