Rapid Test and Assessment of Lithium-ion Battery Cycle Life Based on Transfer Learning
IEEE Transactions on Transportation Electrification(2024)
摘要
The cycle life test provides crucial support for using and maintenance of lithium-ion batteries. The mainstream way to obtain the battery life is uninterrupted charge-discharge testing, which usually takes one year or even longer and hinders the industry development. How to rapidly assess the life of new battery is a challenging task. To solve this problem, a rapid life test method is proposed in this paper, which replaces the continuous test with prediction to suit for different types of battery. This approach unites feature-based transfer learning (TL) and prediction for the first time in life assessment. The similarities and joint characteristics between different type of batteries can be learned in feature-based TL, which includes charge/discharge protocol, and degradation trend. The fine-tuning operation is avoided compared with traditional TL to make life assessment more effective with less effort, which can ensure the interpretability and generalization. The battery internal characteristics are contained in these features, which are extracted from charge-discharge data. Hence, the prediction accuracy is not affected by the capacity regeneration and cliff drop. To prove the validity, hundreds of thousands of samples are collected and utilized from 139 LIB cells produced by 4 different manufacturers. Experimental results show that the life test speed is increased by 8 times at least compared with the mainstream method. The error is less than 8.7% using the first 100 cycles to predict life with 3000 cycles. By calculation, 653kW·h electricity is saved, and 651kg carbon dioxide emission is reduced in each test. The proposed method is promising for rapid battery cycle life acquisition under various applications such as electric vehicles and energy storage services.
更多查看译文
关键词
Battery life test,Lithium-ion batteries,End of life prediction,Transfer learning (TL),Feature extraction
AI 理解论文
溯源树
样例
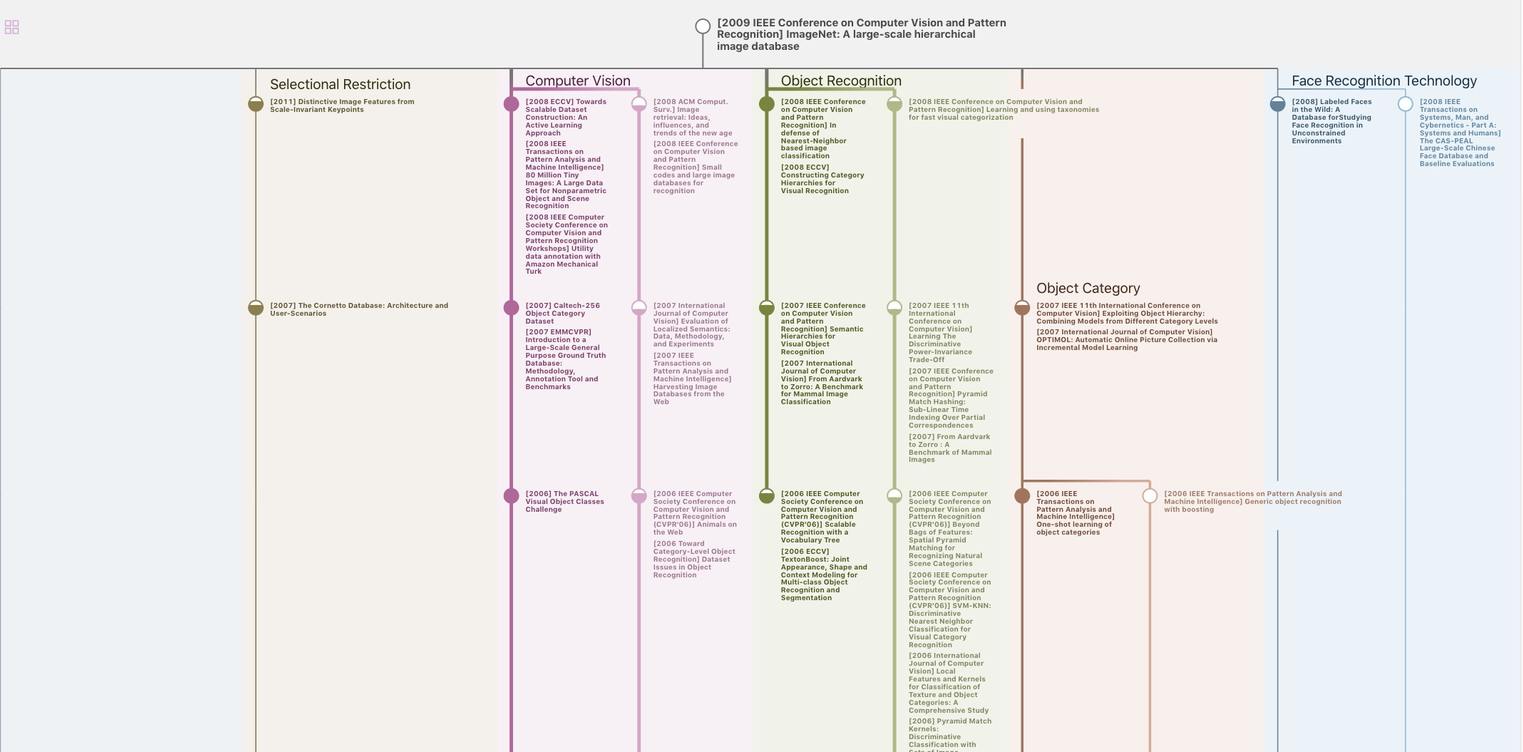
生成溯源树,研究论文发展脉络
Chat Paper
正在生成论文摘要