Multiscale Attention Fusion Graph Network for Remote Sensing Building Change Detection.
IEEE Transactions on Geoscience and Remote Sensing(2024)
摘要
With the development of imaging systems and satellite technology, higher quality high-resolution RS images are being applied in building change detection (BCD) techniques. Methods based on convolutional neural network (CNN) have achieved excellent success in BCD techniques due to their excellent feature discrimination ability. However, CNN relies heavily on the geometry of prior conditions and is limited by the size of the convolution kernel, making it easy to ignore global information. This makes it difficult to capture the long-range dependence of different building targets and handle complex spatial relationships in high-resolution satellite RS images. Considering that graph convolutional neural networks (GCN) have powerful internal relationship learning capabilities, we propose a multi-scale attention fusion graph network (MAFGNet) in this paper. MAFGNet uses a dual graph convolution module (DGM), which includes a spatial graph convolution network (SGCN) and a channel graph convolution network (CGCN), to effectively explore the long-range relationship between the detection target and the global at the spatial and channel levels. We also design a multi-scale attention fusion encoder that includes channel and spatial attention fusion modules to effectively combine valuable information from multi-scale features. In addition, an atrous context self-attention pyramid (ACSP) is designed to combine multi-scale context to enhance the feature representation of change information. We conducted qualitative and quantitative comparative experiments on different datasets to validate the effectiveness of our model. The experimental results show that our method performs better than advanced methods in terms of overall accuracy and visualization details. Our code is available at https://github.com/ShangGY805/MAFG.
更多查看译文
关键词
Building change detection,high-resolution remote sensing images,attention fusion module,dual graph-convolution module,atrous context self-attention pyramid
AI 理解论文
溯源树
样例
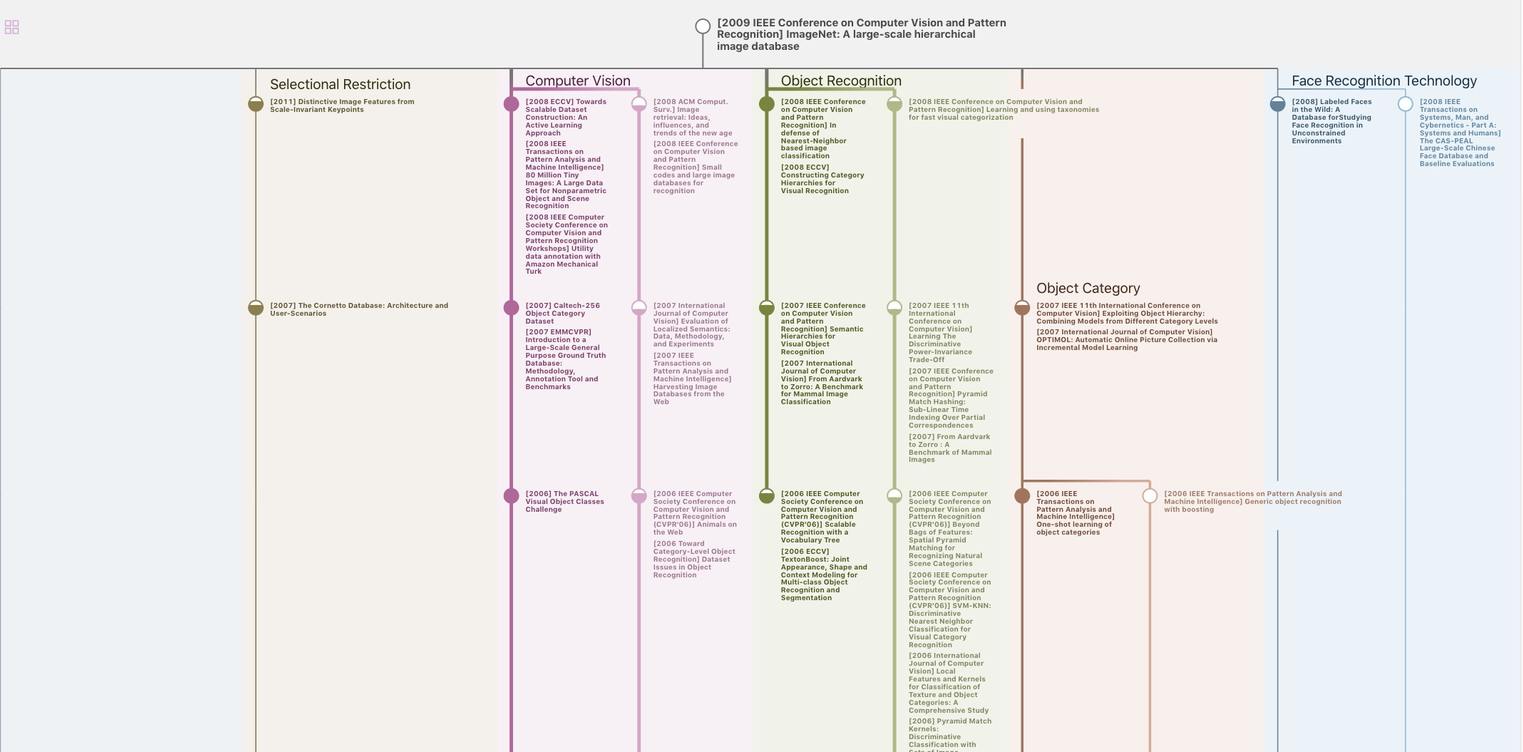
生成溯源树,研究论文发展脉络
Chat Paper
正在生成论文摘要