Sample Weighting with Hierarchical Equalization Loss for Dense Object Detection
IEEE TRANSACTIONS ON MULTIMEDIA(2024)
摘要
Label assignment (LA) is one of the essential phases in the object detection paradigm and aims to classify samples as foreground or background. Current LA strategies generally discriminate samples by explicit thresholds and then calculate weighted losses based on their significances. However, existing methods mostly neglect to consider the importance of samples comprehensively due to the uneven distribution of objects and the limitations of detector structures. In this paper, we propose a hierarchical equalization loss (HEL) by reconsidering the underlying factors affecting sample weights. First, we mitigate sample imbalance at three progressive levels. (1) Task level. We propose task-reconciled weights (TRW) to overcome the effects caused by inter-task inconsistencies (i.e., the inherent differences of classification and localization). (2) Instance level. We propose instance-aware normalization (IAN) for reconstructing the distribution of sample weights within an instance to suppress environmental noise. (3) Pyramid level. We propose hierarchical modulation (HM) to alleviate the unbalanced distribution of multi-scale objects on feature pyramids. Then, we stack the above three mechanisms and formulate the effective weighted loss. Moreover, we propose a staggered candidate bag construction (SCBC) mechanism to further improve the robustness of our method. Without adding any extra overhead, HEL can improve the performance of representative detectors by an impressive margin. Equipped with HEL, a single "ResNet-50+FPN+Head" detector can achieve a performance of 41.9 AP on COCO under 1x schedule, outperforming other existing LA methods. Extensive experiments conducted on multiple backbones and datasets demonstrate the effectiveness of our method.
更多查看译文
关键词
Object detection,label assignment,hierarchical equalization,weighted loss
AI 理解论文
溯源树
样例
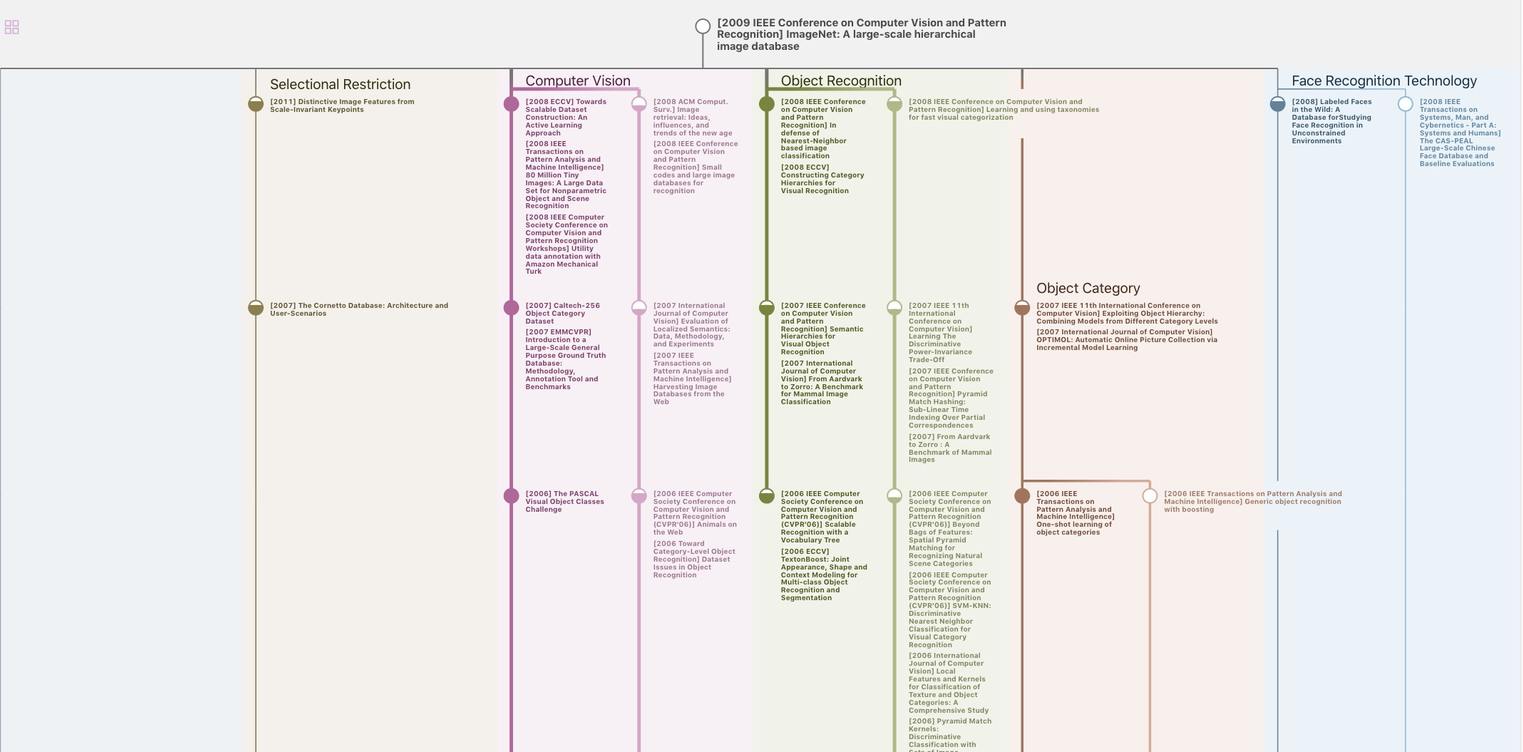
生成溯源树,研究论文发展脉络
Chat Paper
正在生成论文摘要