Adaptive Structure and Texture Similarity Metric for Image Quality Assessment and Optimization.
IEEE Trans. Multim.(2024)
摘要
Objective Image Quality Assessment (IQA) aims to design computational models that can automatically predict the perceived quality of images. The state-of-the-art full-reference IQA metric – Deep Image Structure and Texture Similarity (DISTS), neglects the fact that natural images often consist of local structure and texture, and requires supervised training on the annotated dataset. In this paper, we introduce multiple adaptive strategies to improve DISTS, resulting in an opinion-unaware IQA metric, named A-DISTS. Specifically, A-DISTS first uses the dispersion index as a statistical feature to adaptively localize structure and texture regions at different scales. Second, it adaptively assigns the spatial weights between local structure and texture similarity measurements according to the estimated structure or texture probability maps. Finally, it calculates the entropy of image representation to adaptively weigh the importance of each feature map. As a result, A-DISTS is adapted to local image content and does not require any training. The experimental results demonstrated that the proposed metric correlates well with human rating in the standard and algorithm-dependent IQA databases, and exhibits competitive performance in the optimization tasks of single image super-resolution, motion deblurring, and multi-distortion removal.
更多查看译文
关键词
Full-reference image quality assessment,structure similarity,texture similarity,perceptual optimization
AI 理解论文
溯源树
样例
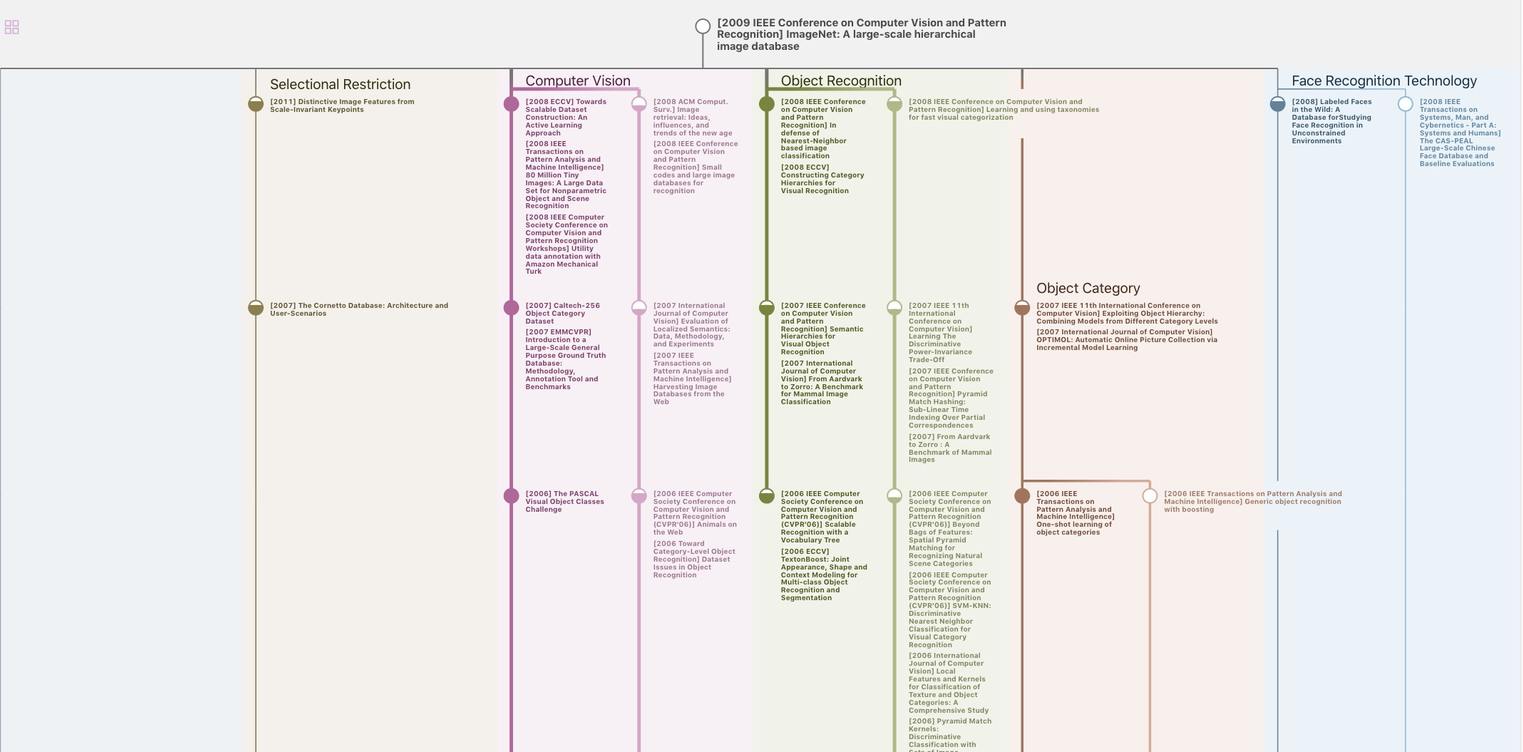
生成溯源树,研究论文发展脉络
Chat Paper
正在生成论文摘要