SYRER: Synergistic Relational Reasoning for RGB-D Cross-Modal Re-Identification.
IEEE Trans. Multim.(2024)
摘要
RGB-D cross-modal person re-identification is designed to match the people across the RGB and depth image modalities, where the large modality discrepancy makes this task intractable to tackle. To alleviate the negative effect brought by the discrepancy, this paper proposes a novel SYnergistic RElational Reasoning (SYRER) method, which targets at exploring the synergy between hetero-modalities for recognizing persons. We design a heterogeneous relationship contrast branch to establish intra-class and inter-class cross-modal relationships, which implements the cross-modal relation contrast learning to cope with imperceptible cross-modal inter-class differences and large cross-modal intra-class discrepancy. Additionally, in order to adequately represent the irregular depth images, we propose a point-wise depth extractor to extract non-uniform discriminative point features from depth images. Experimental results on two public datasets indicate the proposed SYRER surpasses the state-of-the-arts. And we also perform a series of analytic experiments to verify the effectiveness of each submodule of our SYRER.
更多查看译文
关键词
RGB-D Cross-modal Person Re-identification,Cross-modal Relational Reasoning,Relation Contrast learning,Point-wise Depth Extractor
AI 理解论文
溯源树
样例
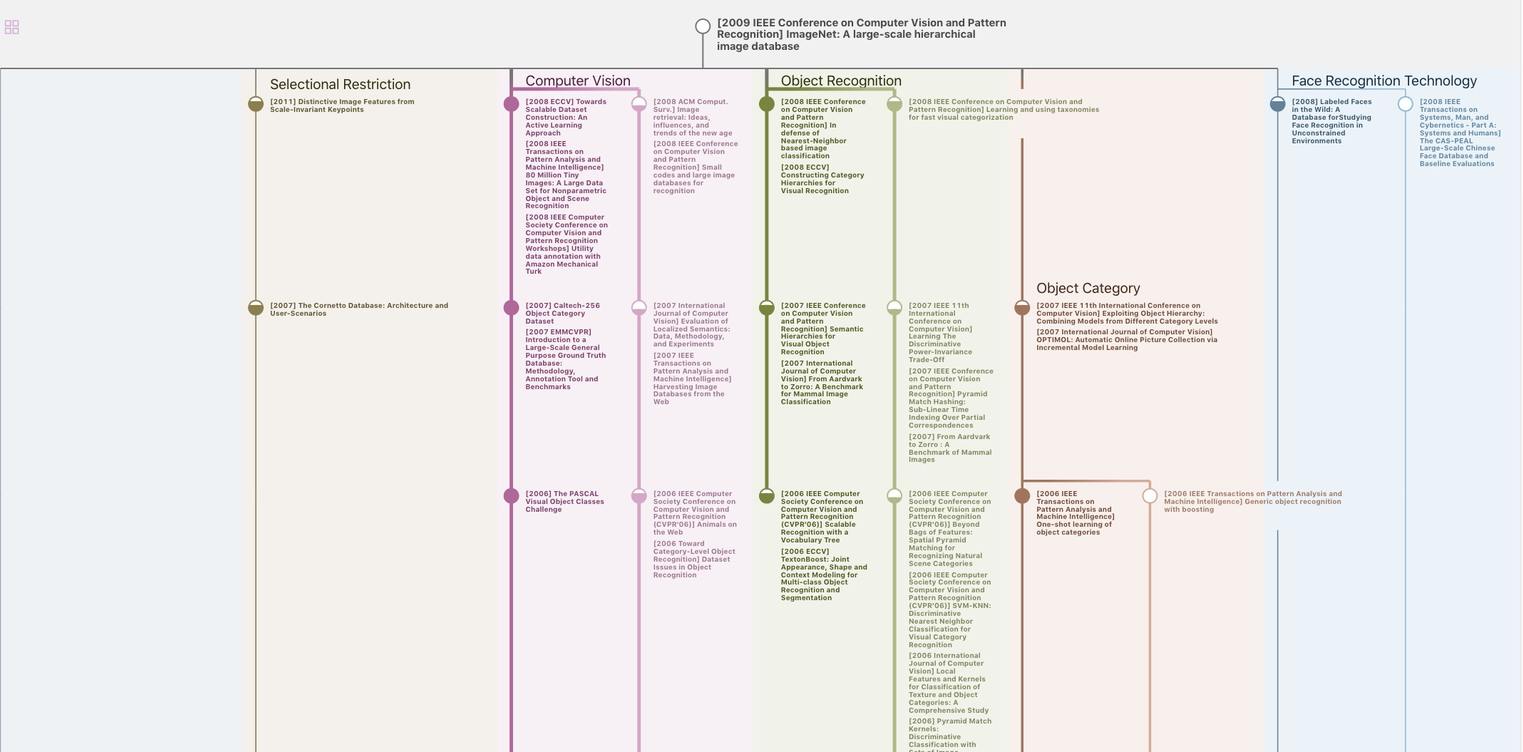
生成溯源树,研究论文发展脉络
Chat Paper
正在生成论文摘要