Perceptual Quality Assessment of Retouched Face Images
IEEE TRANSACTIONS ON MULTIMEDIA(2024)
摘要
Nowadays, it is a common practice to retouch face images before sharing them on websites, social media, and even identification cards. In response, increased criticisms have appeared about taking photo retouching to an extreme. This naturally leads to the necessity of designing perceptual quality assessment methods that can measure how much a retouched face image has strayed from reality. However, such an issue has seldom been considered. In this paper, we conduct both subjective and objective studies to advance this field. Firstly, we construct a benchmark database (termed SZU-RFD) via subjective experiments. SZU-RFD consists of 200 high-quality images with Asian faces and 1,600 retouched images generated by three popular photo-editing tools under different settings. Secondly, considering that retouching usually distorts the image texture, we propose a novel no-reference (NR) quality assessment method, named TANet, for retouched face images by taking the textural artifact into account. Specifically, a texture enhancement module is embedded into the shallow layer to help the network focus on textural information, and a multi-task learning strategy is applied to improve the performance of the main task with the assistance of an auxiliary task, i.e., texture recognition. Extensive experiments on the constructed SZU-RFD show that our proposed TANet correlates well with subjective perceptual judgments and is superior to 19 mainstream NR image quality assessment methods in evaluating retouched face images.
更多查看译文
关键词
Perceptual quality,facial retouching,no-reference,image quality assessment,multi-task learning
AI 理解论文
溯源树
样例
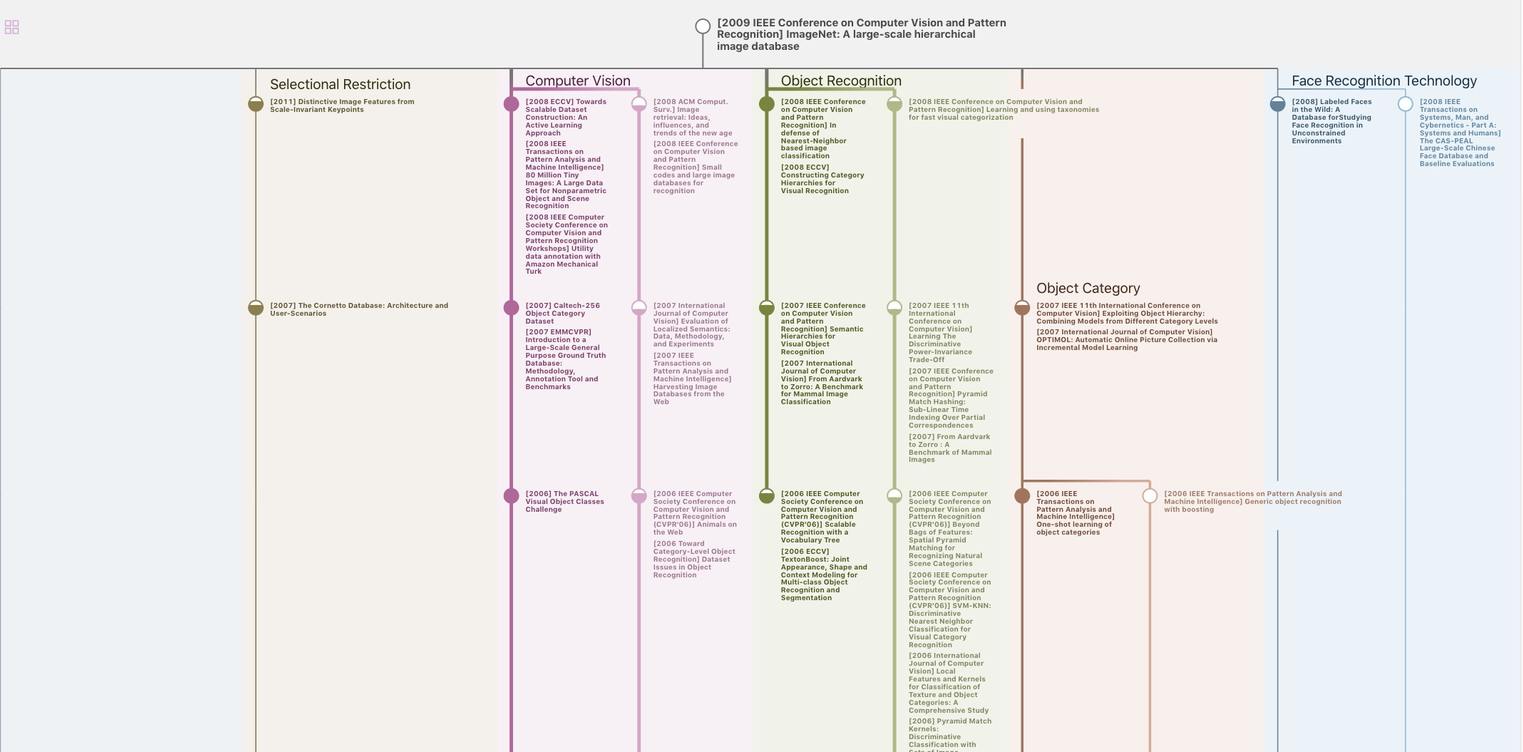
生成溯源树,研究论文发展脉络
Chat Paper
正在生成论文摘要