CMCF-Net: An End-to-End Context Multiscale Cross-Fusion Network for Robust Copy-Move Forgery Detection
IEEE Transactions on Multimedia(2023)
摘要
Image copy-move forgery detection (CMFD) has become a challenging problem due to increasingly powerful editing software that makes forged images increasingly realistic. Existing algorithms that directly connect multiple scales of features in the encoder part may not effectively aggregate contextual information, resulting in poor performance. In this paper, an end-to-end context multiscale cross-fusion network (CMCF-Net) is proposed to detect image copy-move forgery. The proposed network consists of a multiscale feature extraction fusion (MSF) module and a multi-information fusion decoding (MFD) module. Multiscale information is efficiently extracted and fused in the MSF module utilizing stacked-scale feature fusion, which improves the network's forgery localization ability on objects of different scales. The MFD module employs contextual information combination and weighted fusion of multiscale information to guide the network in obtaining relevant clues from correlated information at multiple different scales. Experimental results and analysis have demonstrated that the proposed CMCF-Net achieves the best localization results with higher robustness.
更多查看译文
关键词
copy-move forgery detection,deep neural network,image forensics,depthwise separable convolution
AI 理解论文
溯源树
样例
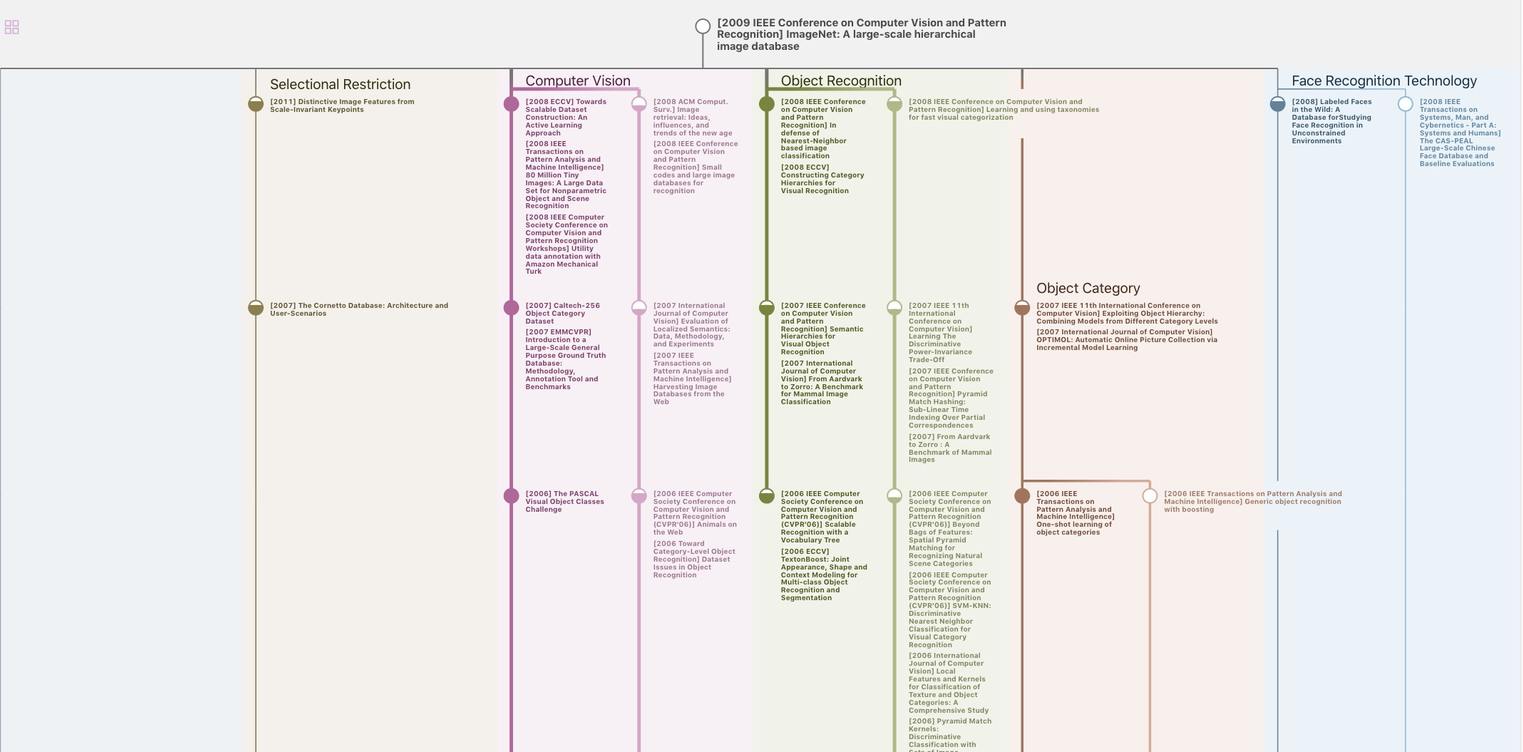
生成溯源树,研究论文发展脉络
Chat Paper
正在生成论文摘要