Learning-Based Auction for Matching Demand and Supply of Holographic Digital Twin Over Immersive Communications
IEEE TRANSACTIONS ON MULTIMEDIA(2024)
摘要
Digital Twin (DT) technologies create digital models of physical entities frequently in multimedia forms, which are crucial for concurrent simulation and analysis of real-world systems. In displaying DTs, Holographic-Type Communication (HTC) provides immersive multimedia access for users to interact with Holographic DTs (HDTs) by transmitting holographic data such as Light Field (LF) and other multisensory information. HDT has applications in remote education, work, and social interactions. However, the effective matching of demand and supply between HDT users and providers remains a challenge. To address this issue, we propose a hierarchical architecture that integrates the DT and HTC paradigms. This architecture incorporates a marketplace for HDT services, leveraging a formulated Double Dutch Auction (DDA) mechanism to optimize matching and pricing based on user and provider valuation. Furthermore, We employ an actor-critic-based Deep Reinforcement Learning (DRL) algorithm to train a DDA auctioneer that dynamically adjusts auction clocks during the auction process. As an alternative to the Multi-layer Perceptron (MLP), we experiment with a Deep Simplistic Variational Quantum Circuit (DSVQC) to reduce the number of parameters and enhance performance stability. Our simulations reveal that the proposed learning-based auctioneer achieves 92% optimal social welfare at a 37% auction information exchange cost for an MLP-based actor and 99% optimal social welfare at a 77% auction information exchange cost for a DSVQC-based actor.
更多查看译文
关键词
Auction theory,digital twin,holographic-type communication,immersive communication,machine learning
AI 理解论文
溯源树
样例
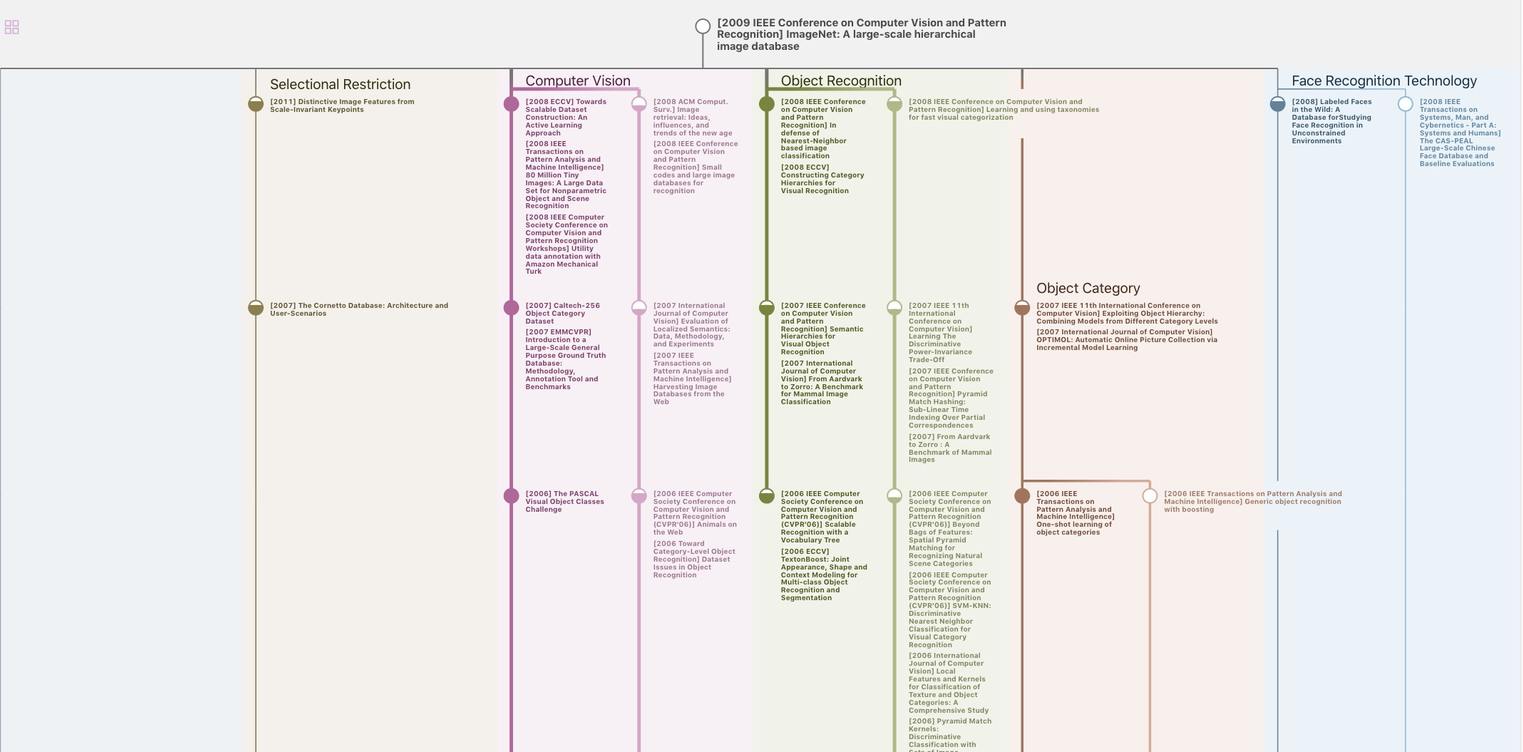
生成溯源树,研究论文发展脉络
Chat Paper
正在生成论文摘要