Structural Analysis of the Stochastic Influence Model for Identifiability and Reduced-Order Estimation
IEEE TRANSACTIONS ON SYSTEMS MAN CYBERNETICS-SYSTEMS(2024)
摘要
The influence model (IM) is a reduced-order stochastic network model that captures the spatiotemporal dynamics in a network of interactive Markov chains. Identifiability and reduced-order estimation of the IM from observation data are crucial for IM applications. Despite the tractability of IM analysis with its reduced-order representation, the identifiability and estimation of IM are challenging due to the tight coupling of both network and local level interactions. The limited identifiability studies in the literature only apply to homogeneous IMs and existing methods for IM estimation incur high-computational cost. In this article, we solve the identifiability problem by providing succinct if-and-only-if conditions for both the homogeneous and heterogeneous IMs. This is obtained through a structural analysis that establishes a novel connection between the high-order and low-order representations of the IMs. The identifiability analysis further leads to reduced-order parameter estimation algorithms of the homogeneous and heterogeneous IMs with reduced computation.
更多查看译文
关键词
Markov processes,Estimation,Analytical models,Computational modeling,Topology,Parameter estimation,Network topology,identifiability,influence model (IM),stochastic networks
AI 理解论文
溯源树
样例
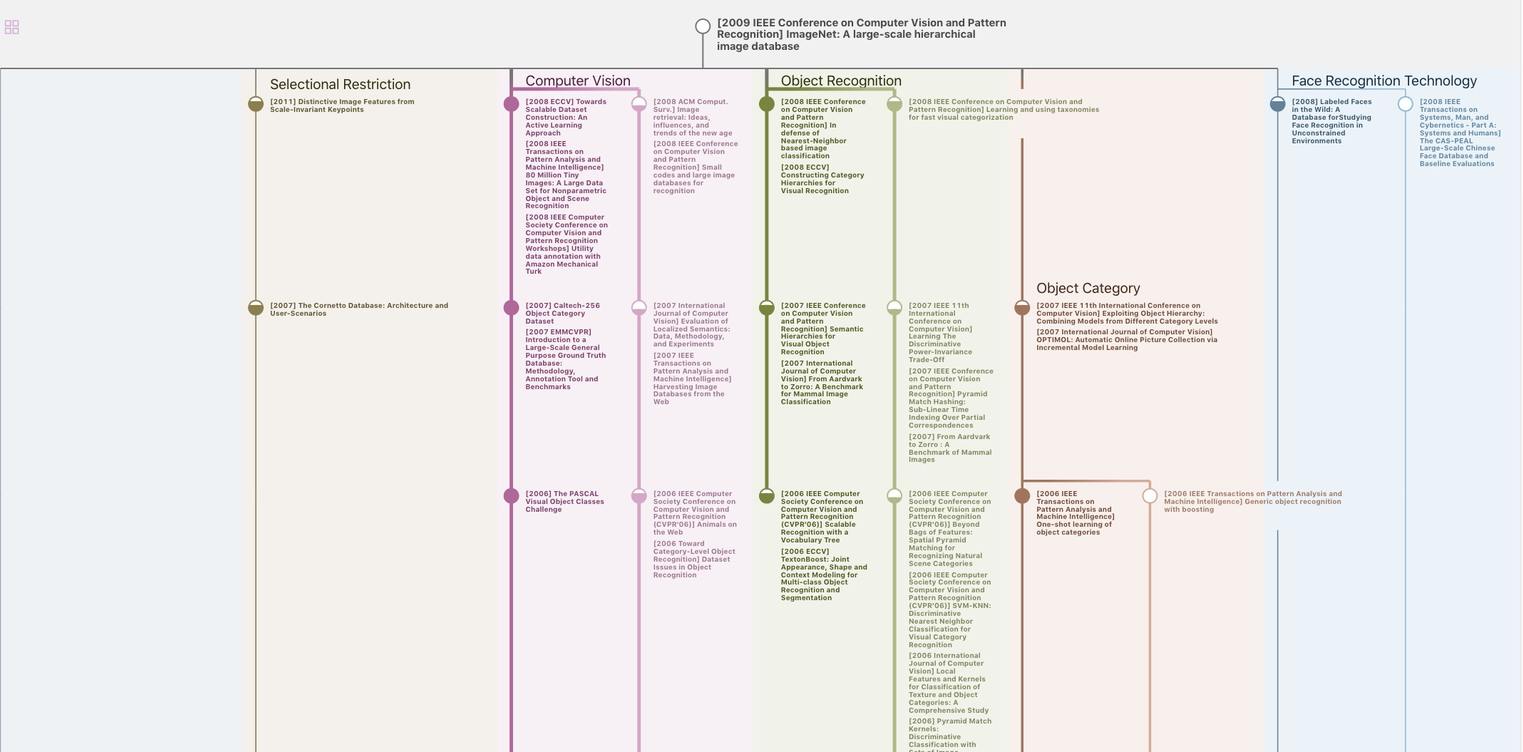
生成溯源树,研究论文发展脉络
Chat Paper
正在生成论文摘要