Wi-Fi and Radar Fusion for Head Movement Sensing Through Walls Leveraging Deep Learning
IEEE Sensors Journal(2023)
摘要
The detection of head movement plays a crucial role in human-computer interaction systems. These systems depend on control signals to operate a range of assistive and augmented technologies, including wheelchairs for Quadriplegics, as well as virtual/augmented reality and assistive driving. Driver drowsiness detection and alert systems aided by head movement detection can prevent major accidents and save lives. Wearable devices, such as MagTrack consist of magnetic tags and magnetic eyeglasses clips and are intrusive. Vision-based systems suffer from ambient lighting, line of sight, and privacy issues. Contactless sensing has become an essential part of next-generation sensing and detection technologies. Wi-Fi and radar provide contactless sensing, however in assistive driving they need to be inside enclosures or dashboards, which for all practical purposes in this paper have been considered as through walls. In this study, we propose a contactless system to detect human head movement with and without walls. We used ultra-wideband(UWB) radar and Wi-Fi signals, leveraging machine and deep learning techniques. Our study analyzes the six common head gestures: right, left, up, and down movements. Time-frequency multi-resolution analysis based on wavelet scalograms is used to obtain features from channel state information values, along with spectrograms from radar signals for head movement detection. Feature fusion of both radar and Wi-Fi signals is performed with state-of-the-art deep learning models. A high classification accuracy of 83.33% and 91.8% is achieved overall with the fusion of VGG16 and InceptionV3 model features trained on radar and Wi-Fi time-frequency maps with and without the walls, respectively.
更多查看译文
关键词
RF sensing,behavior monitoring,micro-Doppler signatures,channel state information,deep learning,machine learning,features fusion
AI 理解论文
溯源树
样例
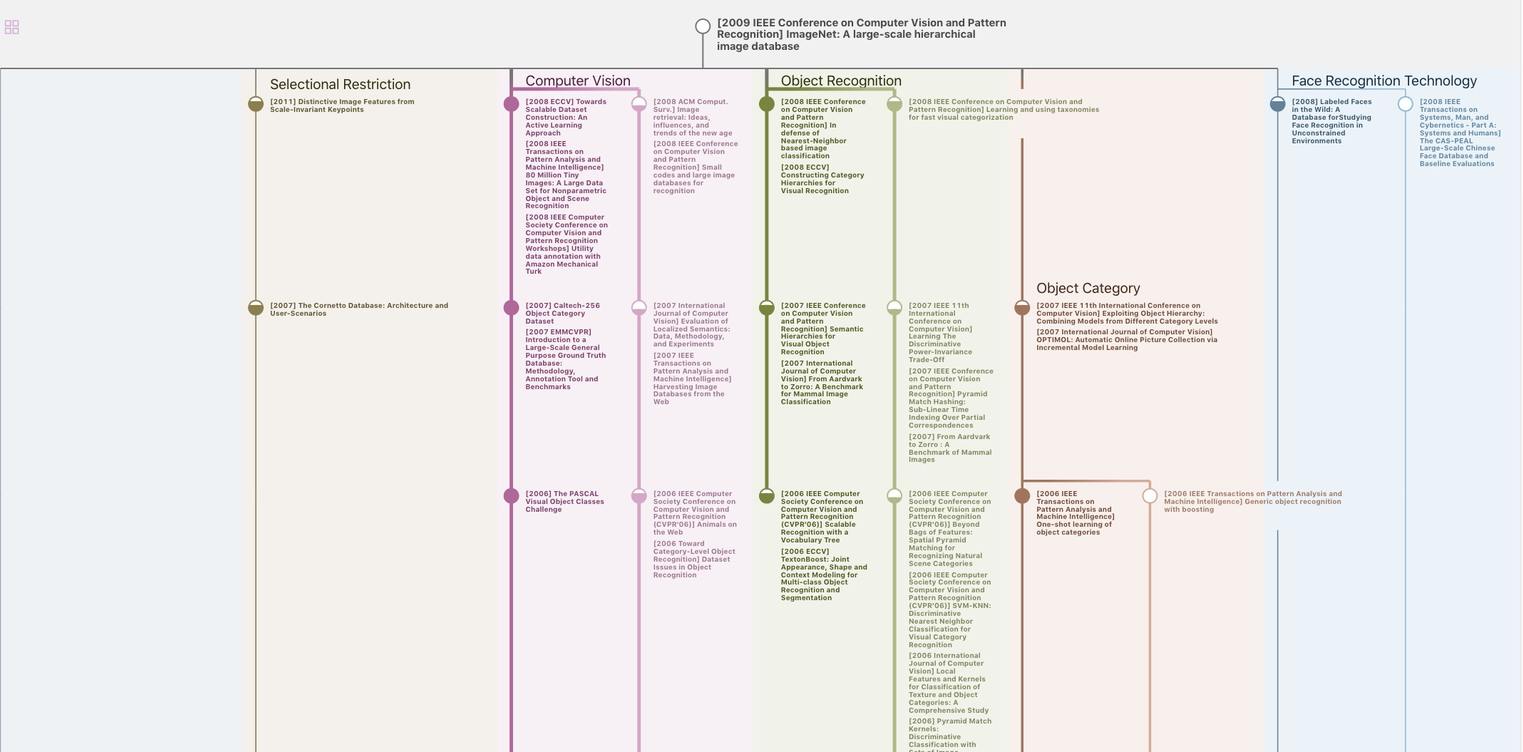
生成溯源树,研究论文发展脉络
Chat Paper
正在生成论文摘要