Seizure detection algorithm based on multidimensional covariance matrix and binary Harris hawks optimization with Cauthy-Gaussian mutation
IEEE Sensors Journal(2023)
摘要
This paper proposes a novel seizure detection algorithm based on multidimensional covariance matrix and hybrid mutation of binary Harris hawks optimizer. First, raw electroencephalography (EEG) signals are preprocessed using empirical mode decomposition (EMD) and discrete wavelet transform (DWT) to obtain several subband signals. Second, single-channel single-subband matrices (SCSS), single-channel multi-subband matrices (SCMS) and multi-channel single-subband matrices (MCSS), are constructed and transformed into covariance matrices. After computing the eigenvalues of each covariance matrix, statistical parameters which include mean, variance, and skewness are extracted to form a feature set. Then, binary Harris hawks optimization (BHHO) algorithm with Cauchy-Gaussian mutation (CGBHHO) is proposed to enhance global search capability and local search capability of BHHO algorithm to accomplish iterative feature selection. Finally, random forest (RF) classifier is employed for automatic seizure detection. The proposed method achieves favorable experimental results with a ten-fold cross-validation evaluation on CHB-MIT database. The results show that the accuracy (ACC), specificity (SPE), sensitivity (SEN), and F1 score (F1) of the method can reach 99.32%, 99.22%, 99.45% and 99.36%, respectively.
更多查看译文
关键词
Muti-channel sensor data,covariance matrix,Cauchy mutation,Gaussian mutation,Harris hawks optimization algorithm,seizure detection
AI 理解论文
溯源树
样例
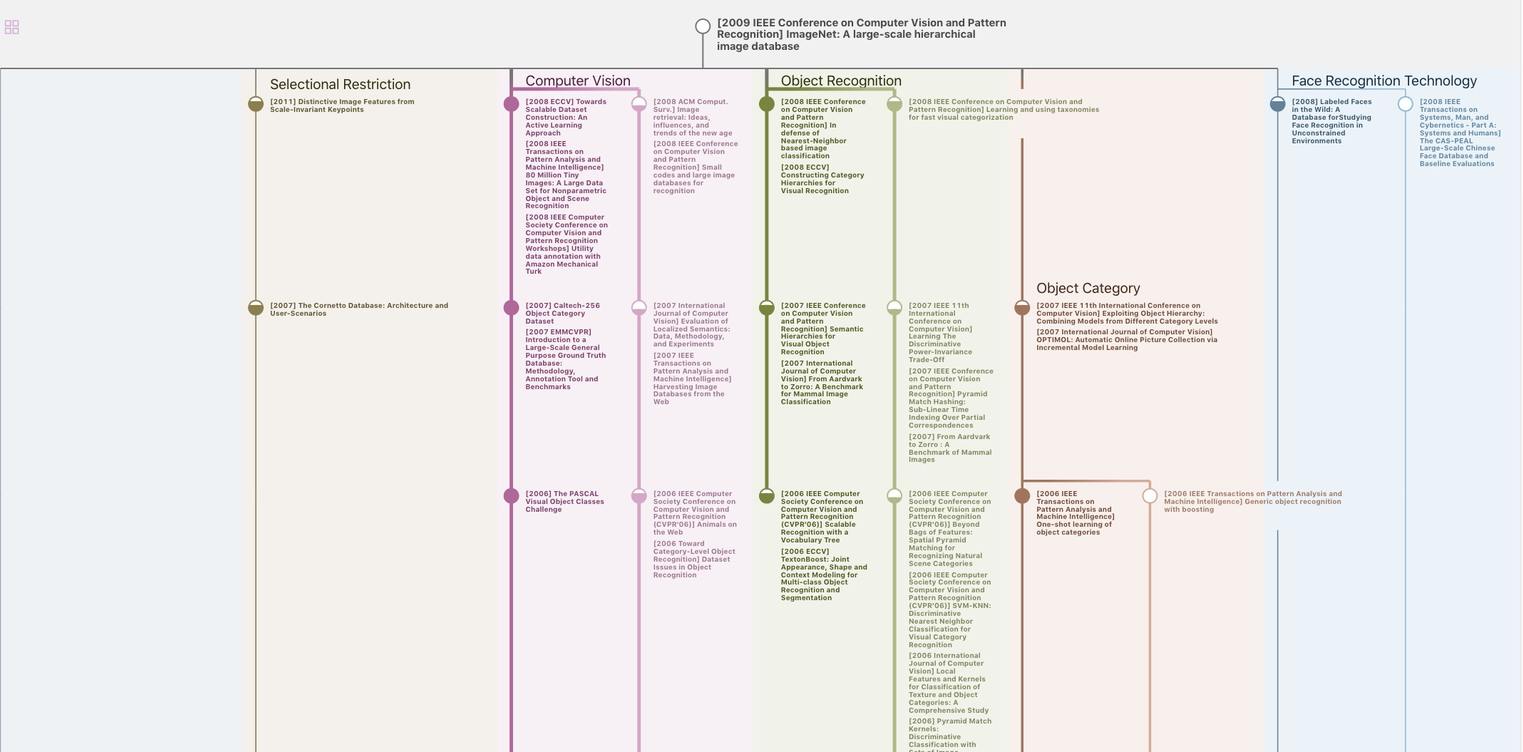
生成溯源树,研究论文发展脉络
Chat Paper
正在生成论文摘要