GTEA: Guided Taylor Expansion Approximation Network for Optical Flow Estimation
IEEE SENSORS JOURNAL(2024)
摘要
Deep neural network (DNN) has proven its powerful ability in feature representation and has been widely applied in computer vision tasks, especially optical flow estimation (OFE). However, these DNN-based OFE approaches have a black-box nature, resulting in lacking a clear interpretation for their success. More unfortunately, they usually suffer from the loss of objects' shape, which is not beneficial for performance improvement. To address these issues, we develop a novel guided Taylor expansion approximation (GTEA) network, including the following key procedures: First, we present the detail analysis of the relationship between Taylor expansion and optical flow. Then, a convolutional guided filter (ConvGF) network is developed to refine the shape of objects. Finally, the final output is obtained by employing basic network, ConvGF, and detail network to approximate the Taylor expansion. Quantitatively and qualitatively experimental results on the Sintel and KITTI datasets demonstrate that the proposed GTEA is effective and even performs better than the state-of-the-art OFE methods on performance improvement and shape preservation.
更多查看译文
关键词
Optical sensors,Taylor series,Estimation,Shape,Optical network units,Task analysis,Optical flow,Endpoint-error (EPE),guided filter,optical flow estimation (OFE),shape preservation,Taylor expansion
AI 理解论文
溯源树
样例
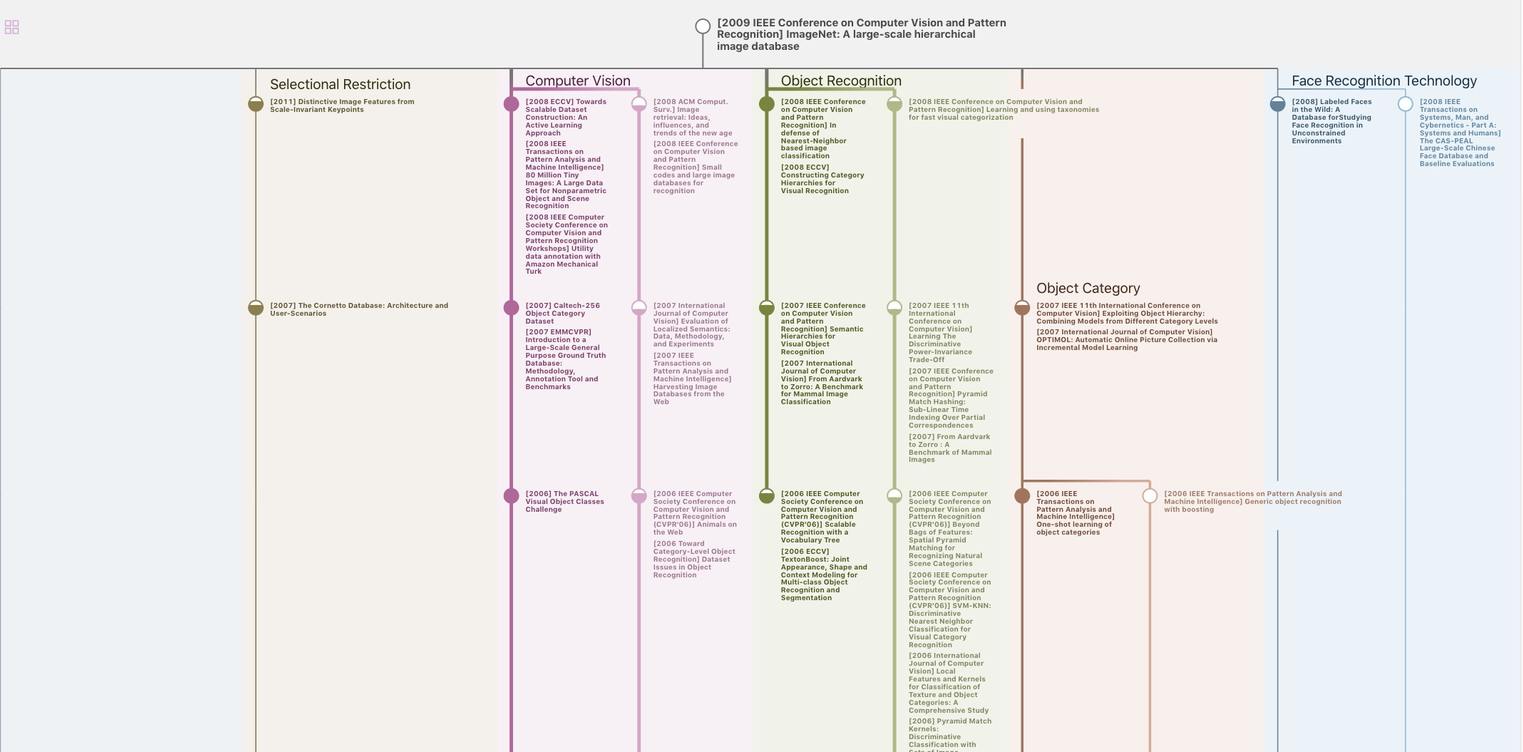
生成溯源树,研究论文发展脉络
Chat Paper
正在生成论文摘要