A Novel Reinforcement Learning Method for Autonomous Driving with Intermittent Vehicle-to-Everything (V2X) Communications
IEEE Transactions on Vehicular Technology(2024)
摘要
Most autonomous driving studies incorporating vehicle-to-everything (V2X) communications assume the existence of continuous V2X communications, and ignore the possibility that V2X communications can be interrupted intermittently. However, when V2X communications are interrupted, autonomous vehicles that rely on the information from V2X communications can fall into disasters. To address these issues, we propose a novel reinforcement learning method named RL4V2X for decision-making and motion-controlling of autonomous driving. This method is composed of a convolutional neural network (CNN), a gate recurrent unit (GRU) and three gate networks. Specifically, the CNN extracts a vehicle spatial distribution to better represent the traffic. The GRU constructs the sequence features to characterize the dynamics of traffic, which can be used to compensate for the lack of information when V2X communications are interrupted. In addition, the gate networks assign the confidence to different features according to the interruption situations. Extensive simulation results in different traffic conditions demonstrate the superior performance of the proposed method.
更多查看译文
关键词
Reinforcement learning,autonomous driving,V2X communications
AI 理解论文
溯源树
样例
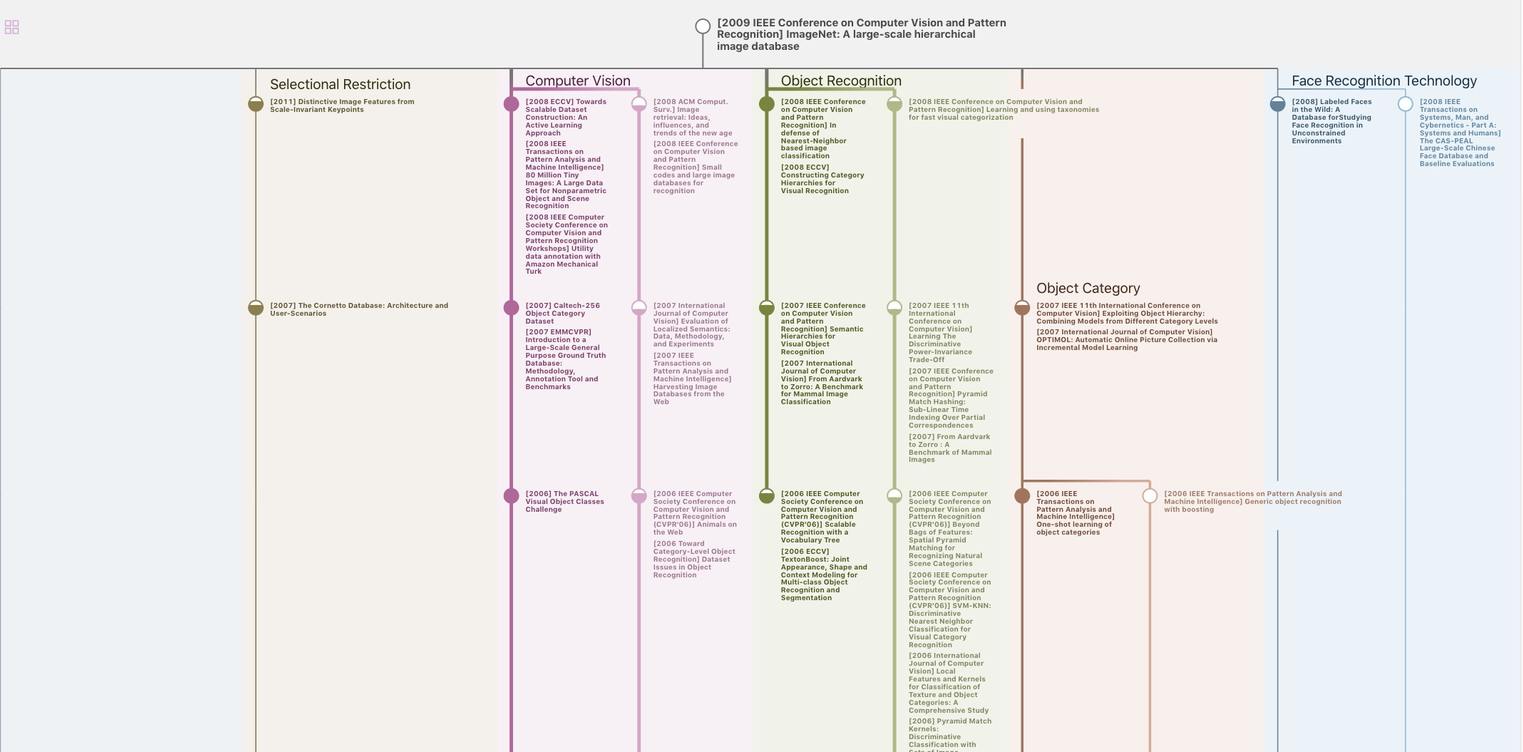
生成溯源树,研究论文发展脉络
Chat Paper
正在生成论文摘要