Deep Data Imputation for UAV Low-Altitude Sensing Considering Spatial-Temporal Interaction
IEEE Sensors Journal(2024)
摘要
With the rapid development of unmanned aerial vehicle (UAV) technology, UAV low-altitude sensing has been gradually applied in multiple fields of data collection and monitoring. However, limited conditions in complex sensor environment lead to the serious deviation or partial loss of data collection. In order to build a complete data base for UAV low-altitude sensing, the imputation method needs to be designed. Previous related work usually focused on separate spatial domain or single time series dimension, regardless of spatial-temporal coordination. In addition, traditional methods such as simple interpolation can not meet the requirements of special monitoring environment. In this paper, we propose a hybrid deep learning model to achieve spatial-temporal data imputation for UAV low-altitude sensing. Specially, the proposed method can be divided into following parts: three dimensional dilated convolution (3D-D-CNN), squeeze with excitation (SE), optimization considering impact of missing data, the targeted Bi-directional long short-term memory (T-Bi-LSTM), and the final fusion module. In addition, we improve the proposed overall architecture by using mathematical methods. On the one hand, we conduct the data preprocessing via Z-score normalization before deep model input. On the other hand, we also take regularized regression based on sparsity and low rank to improve the efficiency. Experiments show that the proposed hybrid deep learning model can effectively finish UAV low-altitude sensing data imputation, and shows its superiority in the development of UAV acquisition and monitoring.
更多查看译文
关键词
UAV low-altitude sensing,spatial-temporal data imputation,hybrid deep learning,Bi-directional LSTM,three dimensional dilated convolution
AI 理解论文
溯源树
样例
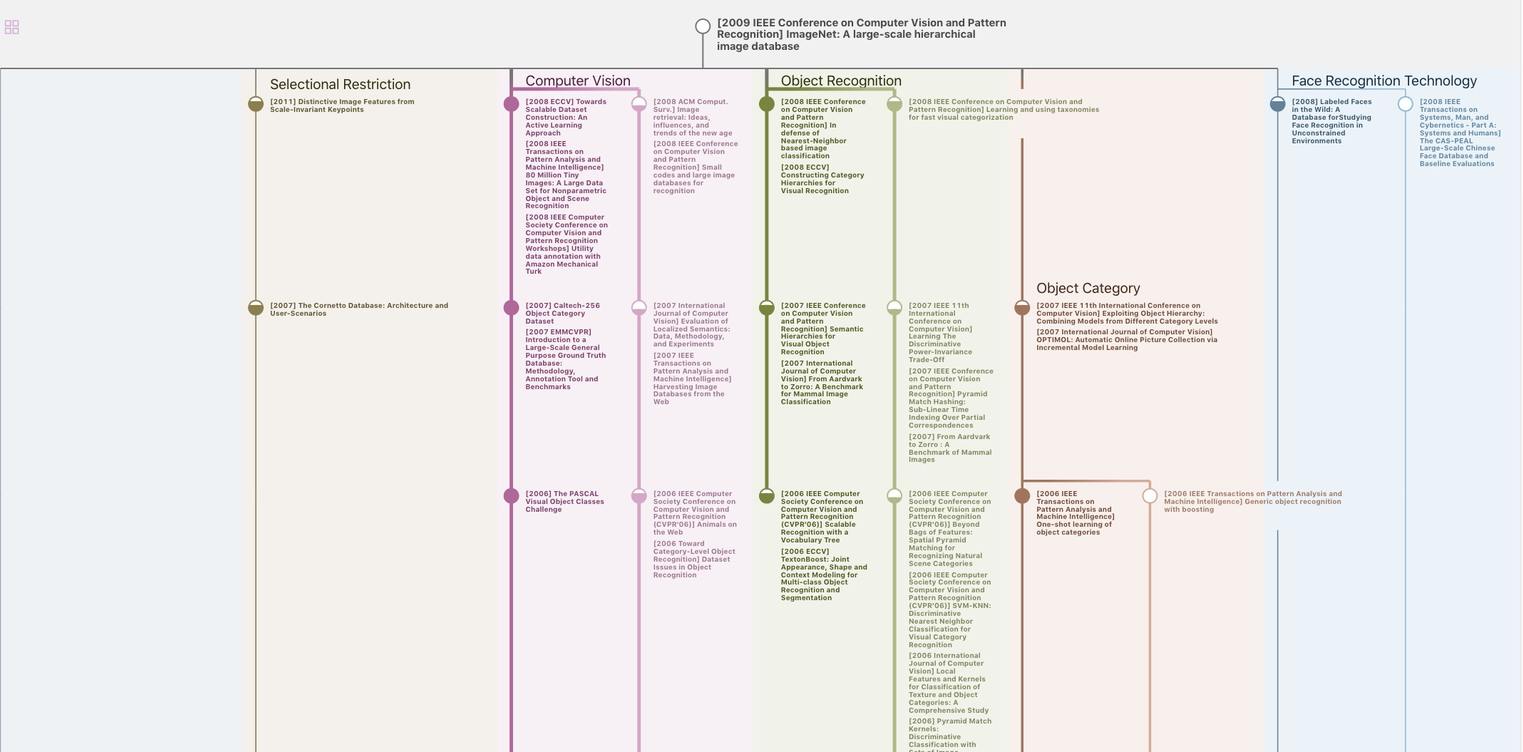
生成溯源树,研究论文发展脉络
Chat Paper
正在生成论文摘要