Feature Matching Machine for Cold-Start Recommendation
IEEE TRANSACTIONS ON SERVICES COMPUTING(2024)
摘要
In recommendation systems, the cold-start issue is a long-standing problem where no historical interaction records are given for certain users or items. Under this circumstance, recommendations for new users or new items become challenging. To address this problem, most existing approaches seek to discover a latent common space for users and items. However, these methods require a strong assumption that a shared space exists where the distributions of users and items are identical, which may limit the recommendation performance. In this article, we propose a novel model called Feature Matching Machine (FMM) to learn latent informative user and item representations. Different from previous methods, for warm users (or items), FMM learns two kinds of latent features, i.e., one is constructed by a hypergraph auto-encoder based on historical interactions between users and items, and the other is built by a multi-layer perceptron based on users (or items). Subsequently, FMM matches these two latent feature representations so as to discover the relationships across users (or items) and cold-start items (or users). We conduct extensive experiments on several real-world datasets and compare the proposed method with well-known baseline methods. Promising results demonstrate the effectiveness and efficiency of the proposed model.
更多查看译文
关键词
Training,Task analysis,Collaboration,Recommender systems,Metalearning,Data models,Correlation,Cold-start recommendation,hypergraph auto- encoder,hypergraph convolution,multi-layer perceptron,recommendation systems
AI 理解论文
溯源树
样例
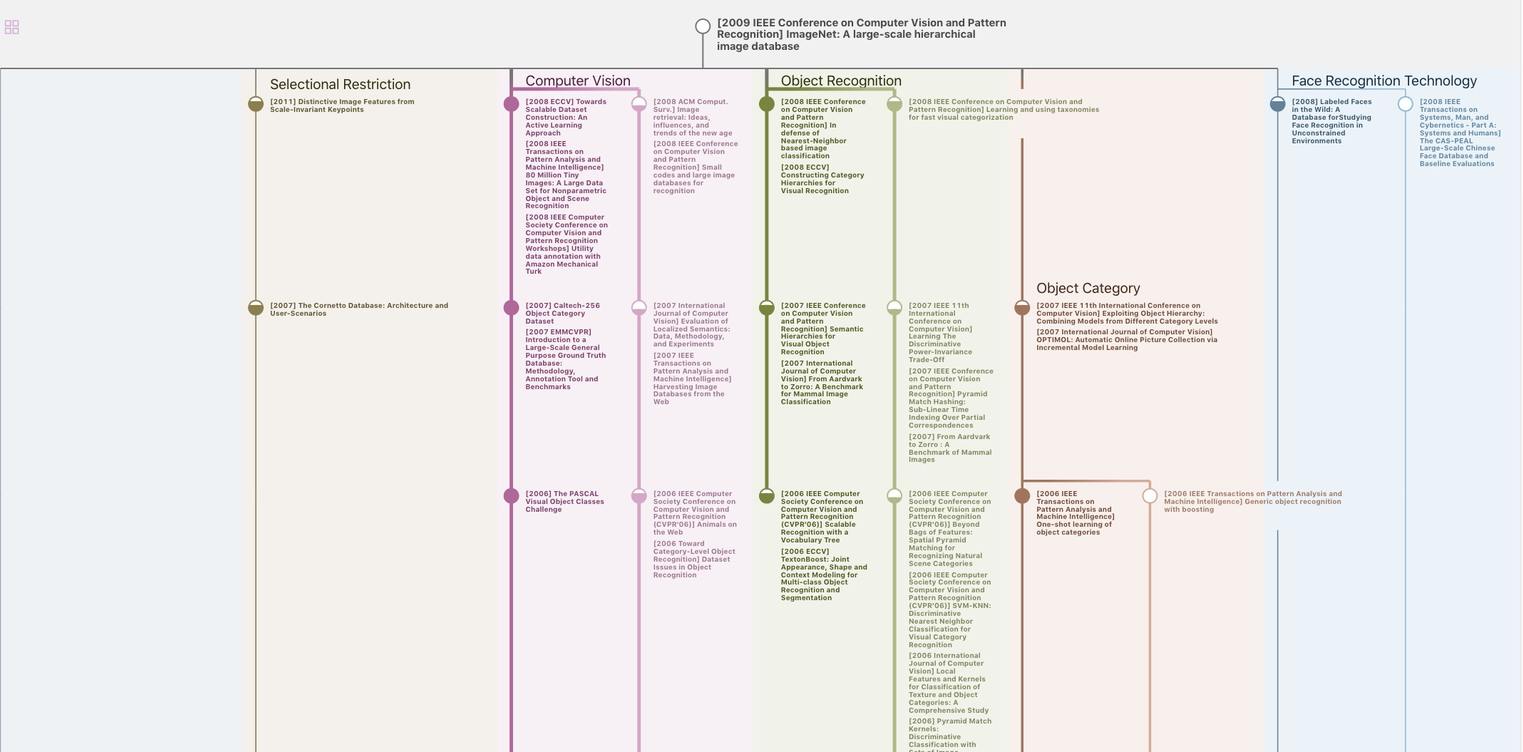
生成溯源树,研究论文发展脉络
Chat Paper
正在生成论文摘要