A Novel Self-Supervised Learning-Based Anomalous Node Detection Method Based on an Autoencoder for Wireless Sensor Networks
IEEE SYSTEMS JOURNAL(2024)
摘要
To address the issue that existing wireless sensor network (WSN)-based anomaly detection methods only consider and analyze temporal features, in this article, a self-supervised learning-based anomalous node detection method based on an autoencoder is designed. This method integrates the extraction of temporal WSN data flow feature, spatial position feature, and intermodal WSN correlation feature into the autoencoder design to make full use of the spatial and temporal information of the WSN for anomaly detection. First, a fully connected network is used to extract the temporal features of nodes by considering a single mode from a local spatial perspective. Second, a graph neural network is used to introduce the WSN topology from a global spatial perspective for anomaly detection and to extract the spatial and temporal features of the data flows of nodes and their neighbors by considering a single mode. Then, an adaptive fusion method involving weighted summation is used to extract the relevant features between different modes. In addition, the gated recurrent unit network structure is introduced to solve the long-term dependence problem in the time dimension. Eventually, the reconstructed output of the decoder and the hidden layer representation of the autoencoder are fed into a fully connected network to calculate the anomaly probability for the current system. Since the spatial feature extraction operation is performed first, the designed method can be applied for anomaly detection in large-scale networks with the addition of a clustering operation. Experiments show that the designed method outperforms baseline methods, and the F1 score reaches 90.6%, at least 5.2% higher than those of existing anomaly detection methods based on unsupervised reconstruction and prediction.
更多查看译文
关键词
Wireless sensor networks,Feature extraction,Anomaly detection,Data models,Correlation,Graph neural networks,Training,Fault detection,machine learning,neural networks,unsupervised learning,wireless sensor networks (WSN)
AI 理解论文
溯源树
样例
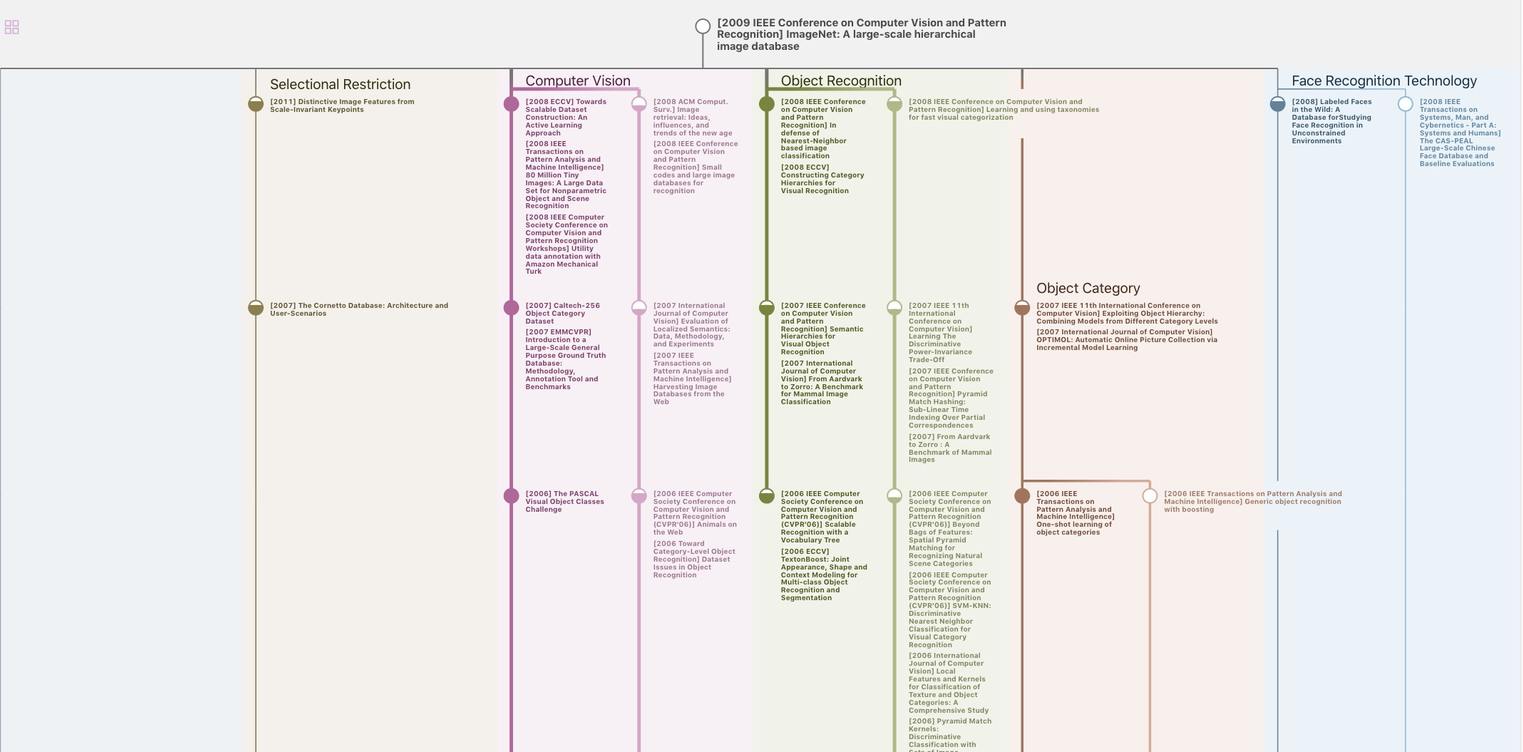
生成溯源树,研究论文发展脉络
Chat Paper
正在生成论文摘要