Understanding and Mitigating Dimensional Collapse in Federated Learning
IEEE Transactions on Pattern Analysis and Machine Intelligence(2023)
摘要
Federated learning aims to train models collaboratively across different clients without sharing data for privacy considerations. However, one major challenge for this learning paradigm is the
data heterogeneity
problem, which refers to the discrepancies between the local data distributions among various clients. To tackle this problem, we first study how data heterogeneity affects the representations of the globally aggregated models. Interestingly, we find that heterogeneous data results in the global model suffering from severe
dimensional collapse
, in which representations tend to reside in a lower-dimensional space instead of the ambient space. This dimensional collapse phenomenon severely curtails the expressive power of models, leading to significant degradation in the performance. Next, via experiments, we make more observations and posit two reasons that result in this phenomenon: 1) dimensional collapse on local models; 2) the operation of global averaging on local model parameters. In addition, we theoretically analyze the gradient flow dynamics to shed light on how data heterogeneity result in dimensional collapse. To remedy this problem caused by the data heterogeneity, we propose
FedDecorr
, a novel method that can effectively mitigate dimensional collapse in federated learning. Specifically,
FedDecorr
applies a regularization term during local training that encourages different dimensions of representations to be uncorrelated.
FedDecorr
, which is implementation-friendly and computationally-efficient, yields consistent improvements over various baselines on five standard benchmark datasets including CIFAR10, CIFAR100, TinyImageNet, Office-Caltech10, and DomainNet. Our code can be found at
https://github.com/bytedance/FedDecorr
.
更多查看译文
关键词
Dimensional collapse,distribution shift,federated learning,representation learning
AI 理解论文
溯源树
样例
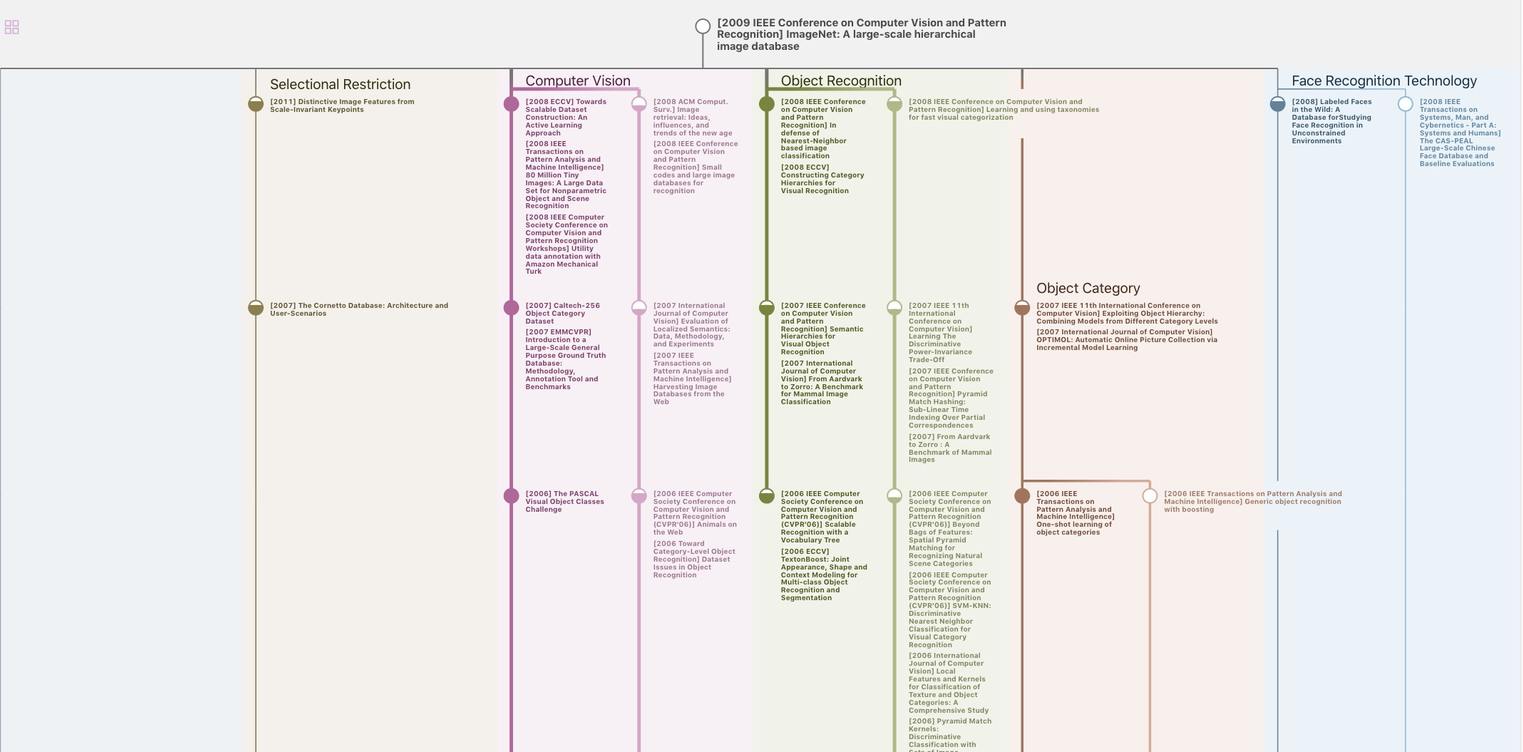
生成溯源树,研究论文发展脉络
Chat Paper
正在生成论文摘要