Real-time dispatch via expert knowledge driven deep reinforcement learning
CSEE Journal of Power and Energy Systems(2023)
摘要
The power system real-time dispatch (RTD) problem optimizes generator outputs considering operational constraints and high-dimensional system information such as nodal loads and network topology. Conventional deep reinforcement learning methods aim to find the optimal dispatch decisions through random exploration and lead to low computational efficiency. In this paper, we decompose RTD into three subproblems, namely the unit commitment, the power adjustment and the voltage adjustment. A reinforcement agent is constructed using the expert knowledge-based twin delayed deep deterministic policy gradient algorithm (EK-TD3) to give the power adjustment decisions, while the unit commitment and voltage adjustment decisions are based on capacity-rules and volt-var sensitivity rules respectively. The regularization terms based on expert knowledge are introduced to guide the exploration of the agent, including generation-load mismatch, midpoint-maintaining rule of balance generator, renewable energy consumption rate and reserve capacity availability. The weight of different regularization terms can be adjusted periodically to reflect the priority of dispatch objectives. The proposed method is tested on the State Grid 126-bus system (SG-126). The results prove that the proposed method has better convergence performance compared with the conventional deep reinforcement learning method, enabling its application in large-scale power systems.
更多查看译文
关键词
real-time dispatch,deep reinforcement learning,twin delayed deep deterministic policy gradient,expert knowledge,regularization terms
AI 理解论文
溯源树
样例
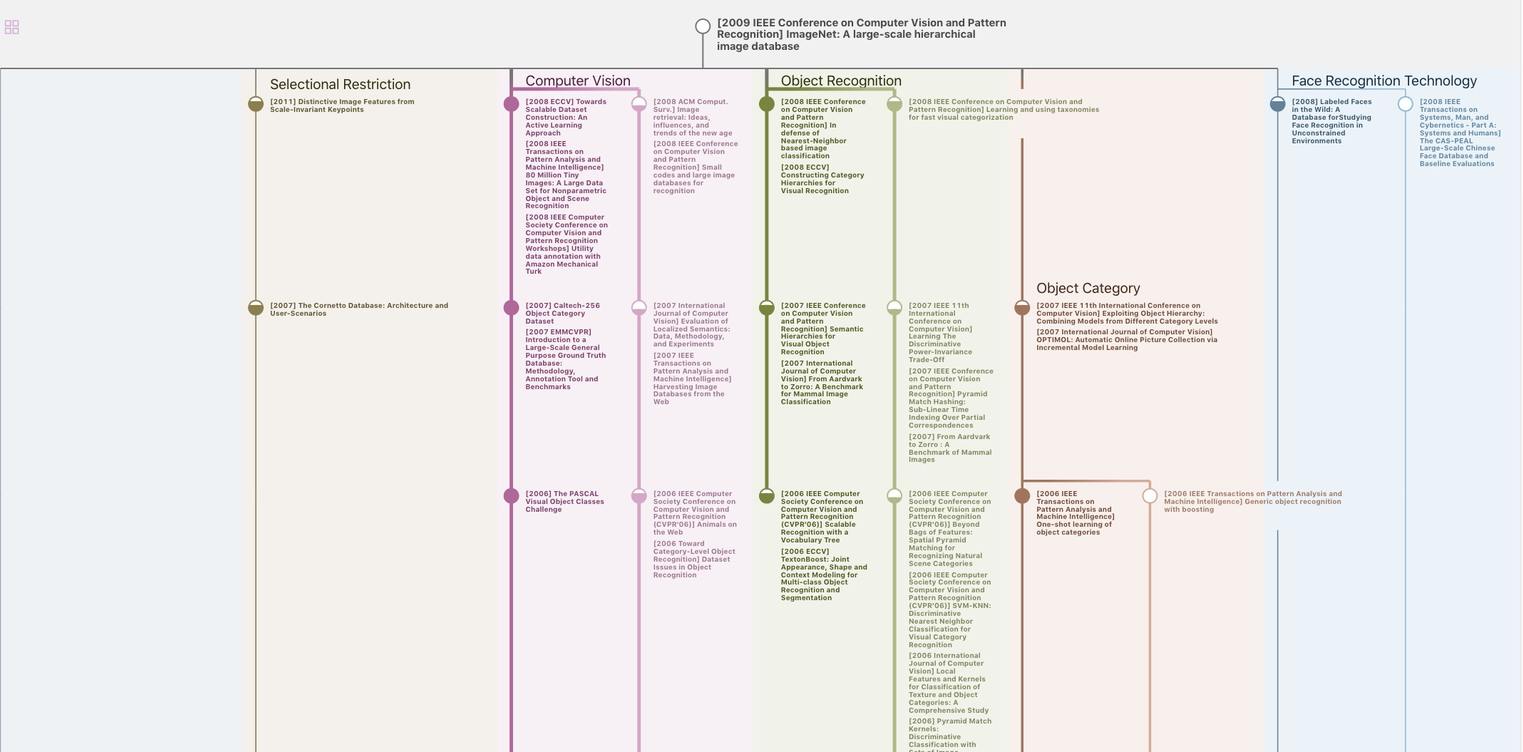
生成溯源树,研究论文发展脉络
Chat Paper
正在生成论文摘要