Efficient Semi-Supervised Segmentation of Medical Images with RESA-Enhanced U-Net.
2023 8th IEEE International Conference on Network Intelligence and Digital Content (IC-NIDC)(2023)
摘要
Medical image analysis relies on accurately identifying anatomical structures. With the emergence of deep learning frameworks, it is now possible to create systems that can automate the segmentation of such data. However, challenges related to polycentric and spatiotemporal inefficiencies persist. Furthermore, the high costs associated with manual labeling and the scarcity of labeled data are significant obstacles in this domain. In this work, we provide a brand-new semi-supervised approach and an enhanced segmentation network to address these issues. By integrating the Recurrent Feature-Shift Aggregator(RESA) structure into the network, we leverage multiple step sizes for information transmission, mitigating potential information loss. In the FLARE2022 examples, our suggested quantitative assessment technique was able to get a DSC of 0.80 and an NSD of 0.75, indicating the effectiveness and resilience of our strategy.
更多查看译文
关键词
U-Net,Multi-organ Segmentation,RESA,Semi-supervised Learning
AI 理解论文
溯源树
样例
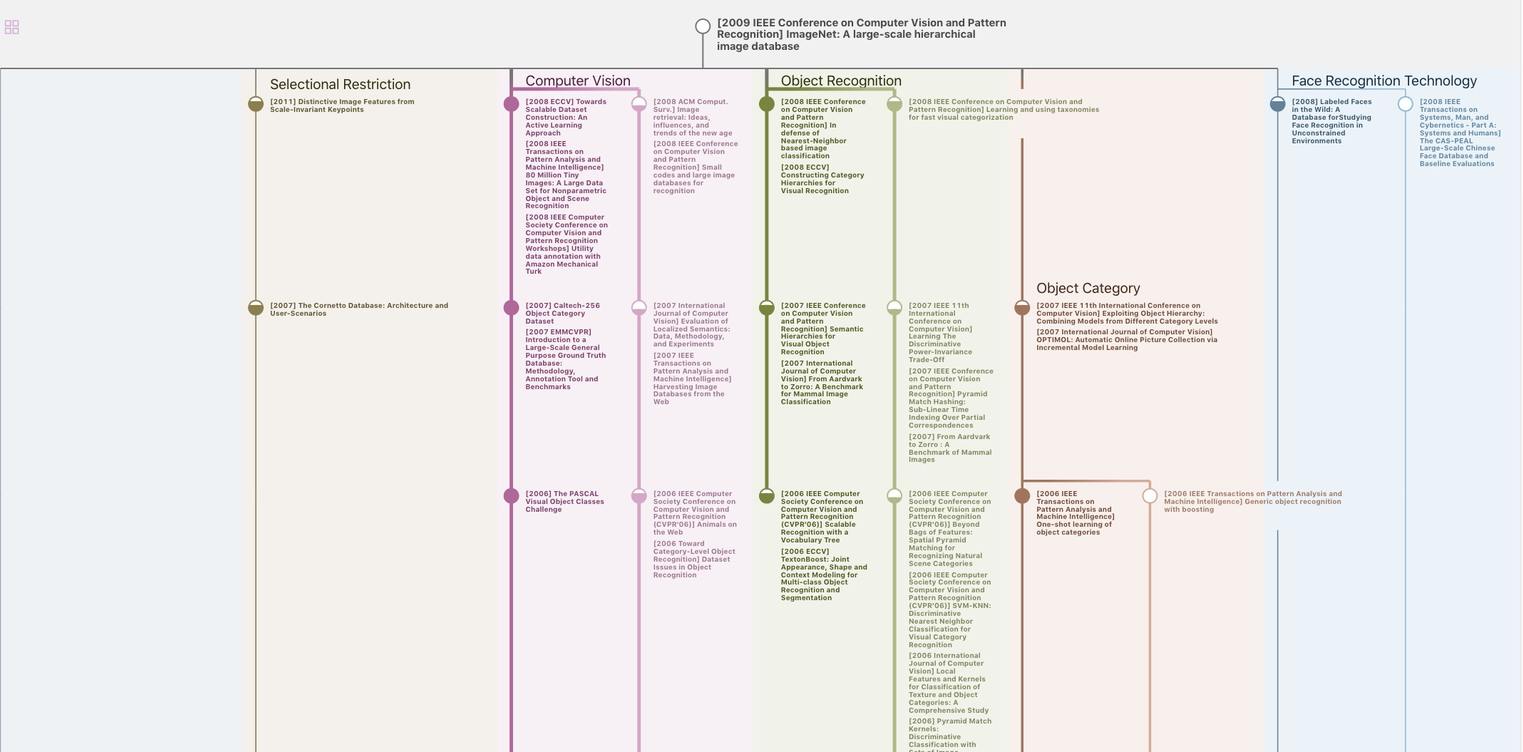
生成溯源树,研究论文发展脉络
Chat Paper
正在生成论文摘要