A Novel Robotic Grasp Detection Framework Using Low-Cost RGB-D Camera for Industrial Bin Picking
IEEE TRANSACTIONS ON INSTRUMENTATION AND MEASUREMENT(2024)
摘要
Industrial bin picking is a challenging task as lots of highly reflective and textureless objects are stacked. Previous methods generally predict gripper configurations or 6-D object pose by expensive industrial 3-D cameras, ignoring the role of consumer-grade red, green, blue, depth (RGB-D) cameras in this task. For highly reflective objects, depth maps of consumer-grade RGB-D cameras are generally of a lower quality with much stronger noise, making it challenging to detect robust grasp poses in stacked industrial scenarios. To address these challenges, we propose a novel grasp detection framework, including a synthetic data generation pipeline, a novel local grasp detection method, and a global search algorithm (GS). Specifically, in the training phase, we utilize the Pybullet and OpenGL to generate synthetic data by approximate 3-D models. We introduce a novel grasp representation and propose MixStyle (MS)-ResUnet to predict and achieve sim2real transfer for this representation. In the testing phase, the object singleness metric (OSM) is proposed for the global search block to evaluate the graspable probability. In addition, the RGB image enhanced by the edge feature is utilized as the input of the network, which can bridge the domain shift. Extensive experiments in real scenes demonstrate that our method can achieve competitive results in grasping disordered metal parts. In over 2400 robotic grasp trials, our method achieves an average success rate of 91.1% in dense stack scenarios. We also confirm that the proposed method can be applied to unseen objects that are not included in the training dataset.
更多查看译文
关键词
Cameras,Three-dimensional displays,Heating systems,Robots,Synthetic data,Service robots,Task analysis,Deep learning,grasp representation,industrial bin picking,low-cost red,green,blue,depth (RGB-D) camera,sim2real
AI 理解论文
溯源树
样例
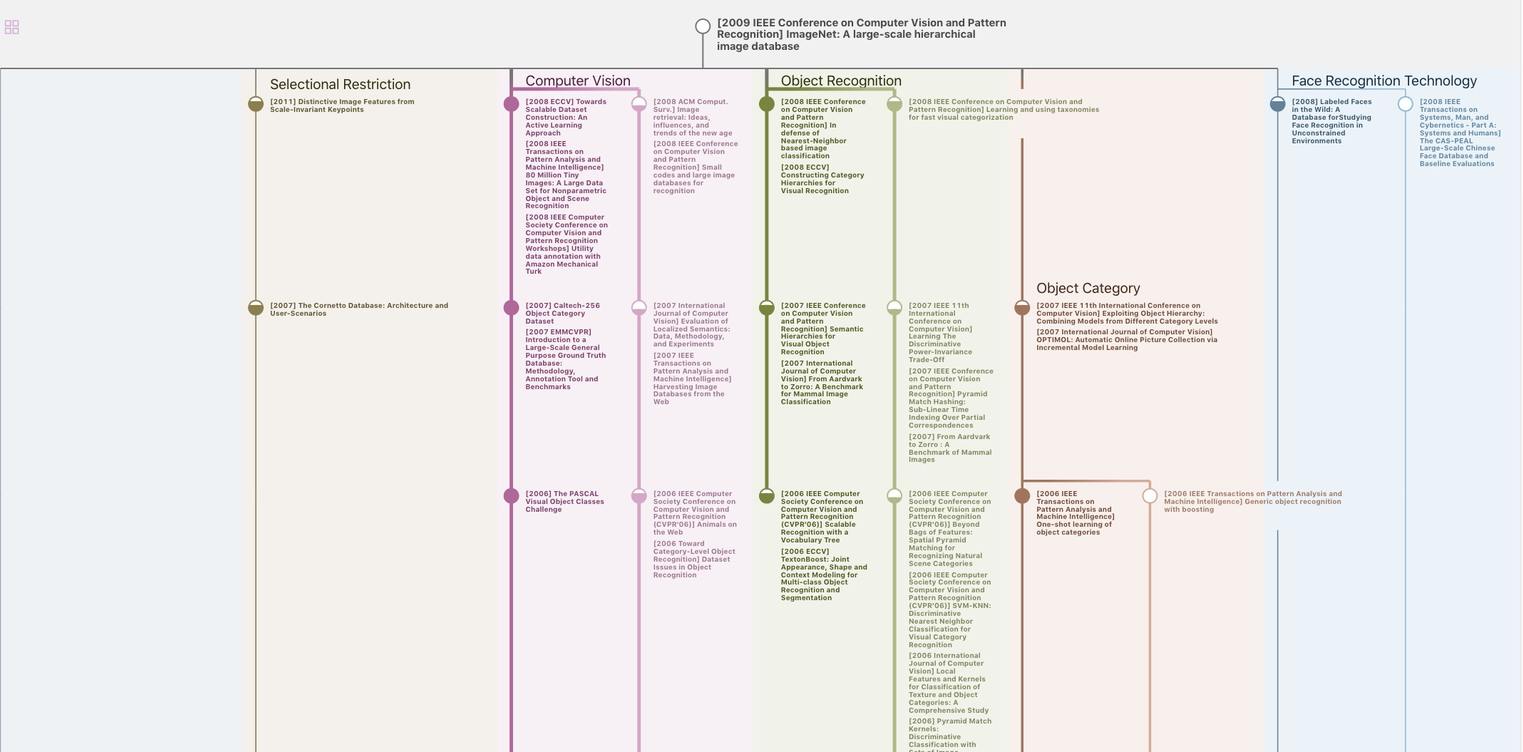
生成溯源树,研究论文发展脉络
Chat Paper
正在生成论文摘要