Physics-Informed Neural Networks With Embedded Analytical Models: Inverse Design of Multilayer Dielectric-Loaded Rectangular Waveguide Devices
IEEE TRANSACTIONS ON MICROWAVE THEORY AND TECHNIQUES(2023)
摘要
The deepening research of physics-informed neural networks (PINNs) demonstrates the advantages of this method to electromagnetic inverse design. Some studies have deeply embedded the physics information into the neural networks, obtaining better solution performance and providing a new way for the PINN electromagnetic inverse design problems. This article explores PINN with embedded analytical models (EAM-PINN) to design waveguide devices. We first develop a multilayer dielectric-loaded rectangular waveguide model and derive its S-21 parameter expressions. Considering the inevitable limits of the S-21 response achievable by the waveguide model, we propose a method to solve the model bounds based on the interior point method to determine the number of dielectric layers. To improve the solution effect of PINN, we also present a hard constraint method based on the activation function. Then, we embed the S-21 parameter expressions, as an S-21 analytical model, into the traditional PINN framework, reducing the scale of loss functions. We also theoretically analyze the performance of EAM-PINN and summarize its parameter selection scheme. The EAM-PINN implements three inverse design cases: a known analytical solution retrieving, a bandpass filter, and a dispersive delay line. Finally, we conclude the advantages of EAM-PINN compared to various current methods and validate our inverse design results through simulation. We find that EAM-PINN has high solving efficiency and strong stability, which can realize the inverse design of complex microwave devices, showing the great potential of this method.
更多查看译文
关键词
Hard constraints,inverse design,model bounds,physics-informed neural networks (PINNs),waveguide device
AI 理解论文
溯源树
样例
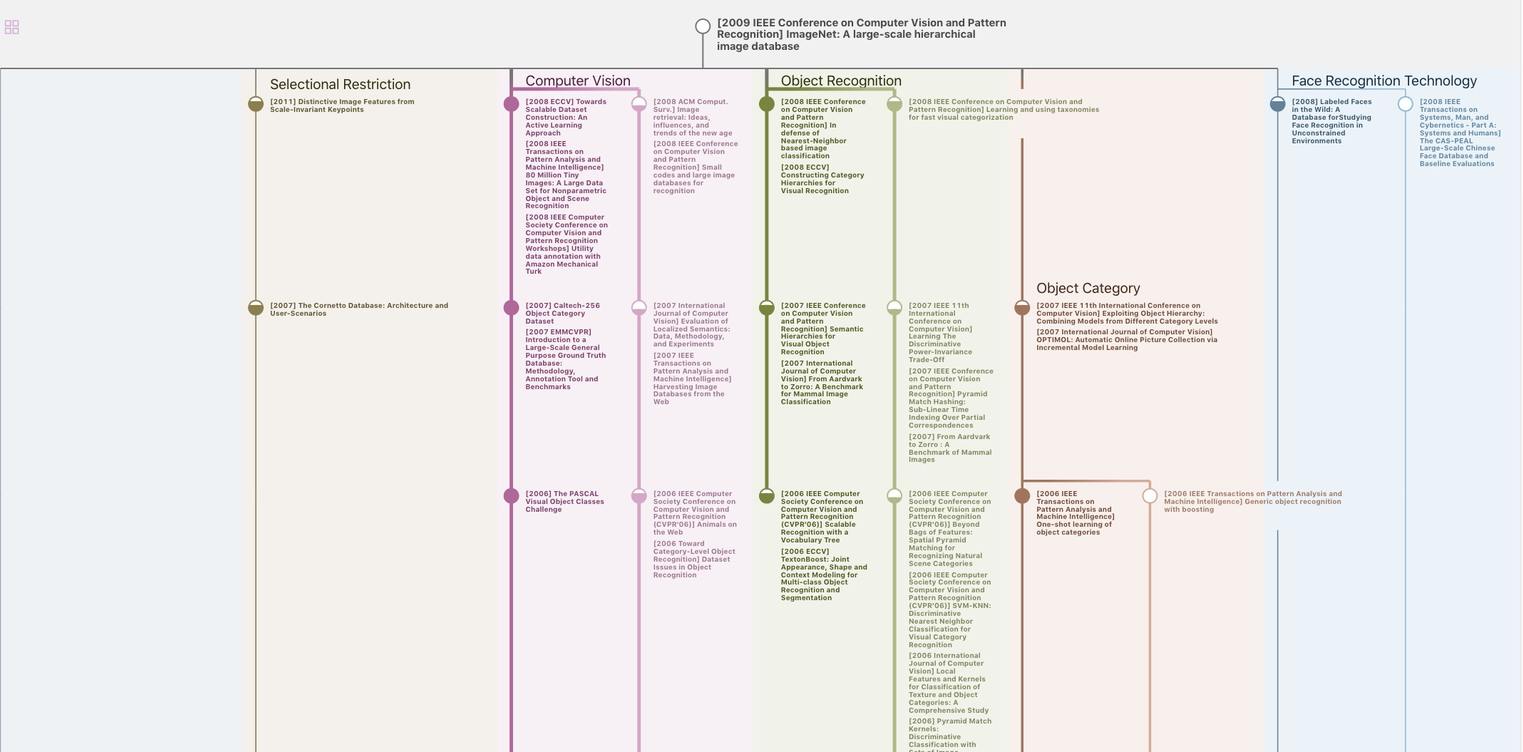
生成溯源树,研究论文发展脉络
Chat Paper
正在生成论文摘要