Sparsely Gated Mixture of Experts Neural Network For Linearization of RF Power Amplifiers
IEEE TRANSACTIONS ON MICROWAVE THEORY AND TECHNIQUES(2023)
摘要
This article presents a piecewise neural network (NN) with dynamic sparsity for modeling and linearization of radio frequency (RF) power amplifiers (PAs). A mixture of experts NN (MENN) approach is employed to combine several smaller real-valued time-delay NNs (RVTDNNs) by means of a gating NN. Furthermore, we complement the MENN framework with top- $K$ sparse gating, such that only a subset of experts is activated during each sample inference, reducing the computational complexity at run time. An end-to-end training approach is presented, to optimize the gating alongside with specializing the expert NNs, enabling the experts to collaborate. We experimentally investigate the scaleability of the proposed model in terms of modeling accuracy and linearization performance, as well as run time and model complexity, using RF measurements with two different gallium-nitride Doherty PAs at 1.8 and 3.5 GHz, respectively. Our experiments confirm a significant reduction in run-time complexity due to the sparse gating, with only a small penalty on accuracy, linearization capability and scaleability. Furthermore, the proposed approach is shown to offer favorable complexity-performance trade-offs, outperforming the existing state-of-the-art.
更多查看译文
关键词
Artificial neural networks,Complexity theory,Training,Radio frequency,Context modeling,Behavioral sciences,Nonlinear distortion,Behavioral modeling,digital predistortion,linearization,mixture of experts neural network (MENN),power efficiency,radio frequency (RF) power amplifiers (PAs),time-delay neural network (NN)
AI 理解论文
溯源树
样例
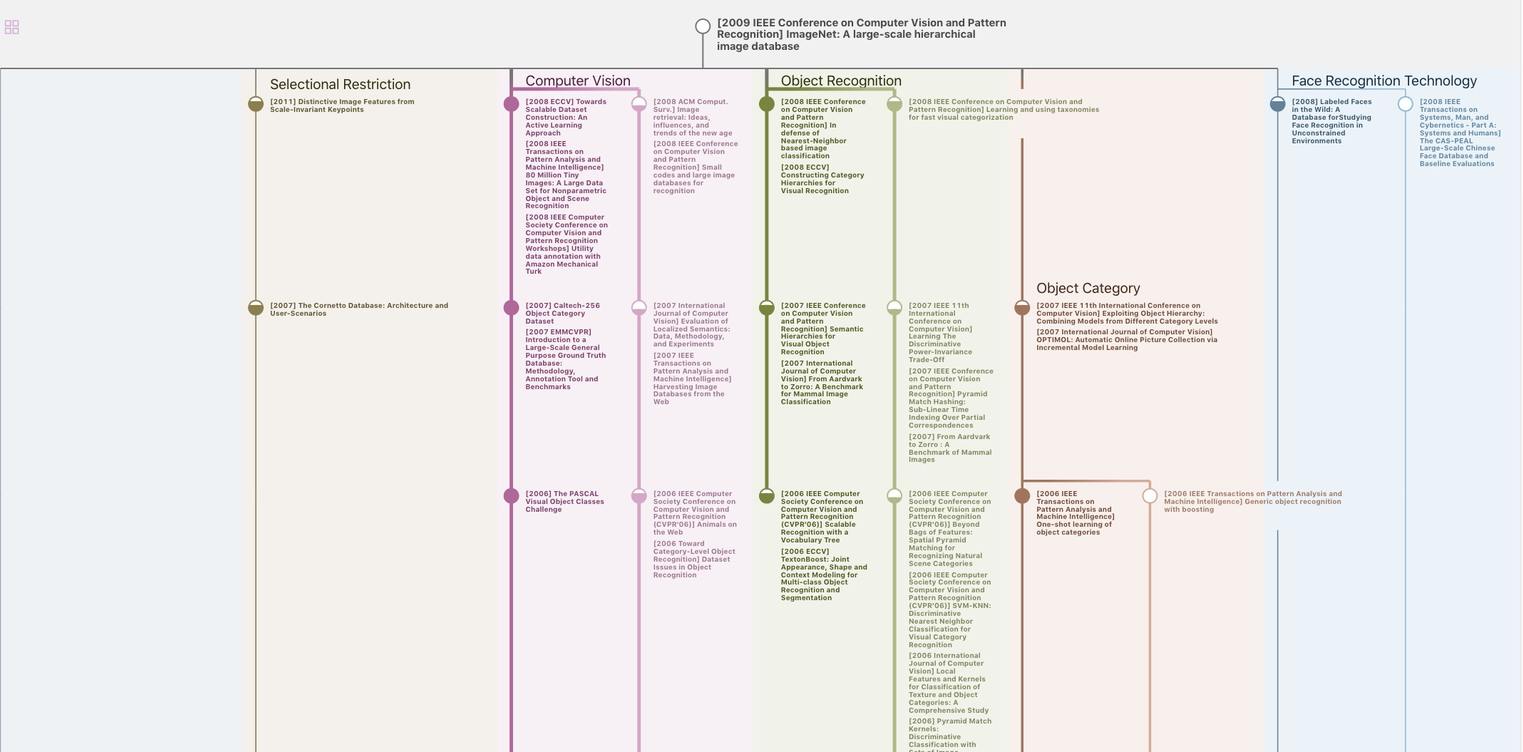
生成溯源树,研究论文发展脉络
Chat Paper
正在生成论文摘要