LIVER: A Tightly Coupled LiDAR-Inertial-Visual State Estimator With High Robustness for Underground Environments
IEEE ROBOTICS AND AUTOMATION LETTERS(2024)
摘要
In this letter, we propose a tightly coupled LiDAR-inertial-visual (LIV) state estimator termed LIVER, which achieves robust and accurate localization and mapping in underground environments. LIVER starts with an effective strategy for LIV synchronization. A robust initialization process that integrates LiDAR, vision, and IMU is realized. A tightly coupled, nonlinear optimization-based method achieves highly accurate LiDAR-inertial-visual odometry (LIVO) by fusing LiDAR, visual, and IMU information. We consider scenarios in underground environments that are unfriendly to LiDAR and cameras. A visual-IMU-assisted method enables the evaluation and handling of LiDAR degeneracy. A deep neural network is introduced to eliminate the impact of poor lighting conditions on images. We verify the performance of the proposed method by comparing it with the state-of-the-art methods through public datasets and real-world experiments, including underground mines. In underground mines test, tightly coupled methods without degeneracy handling lead to failure due to self-similar areas (affecting LiDAR) and poor lighting conditions (affecting vision). In these conditions, our degeneracy handling approach successfully eliminates the impact of disturbances on the system.
更多查看译文
关键词
Laser radar,Simultaneous localization and mapping,Visualization,Feature extraction,Odometry,Cameras,Robustness,SLAM,sensor fusion,localization,LiDAR-inertial-visual SLAM,underground environments
AI 理解论文
溯源树
样例
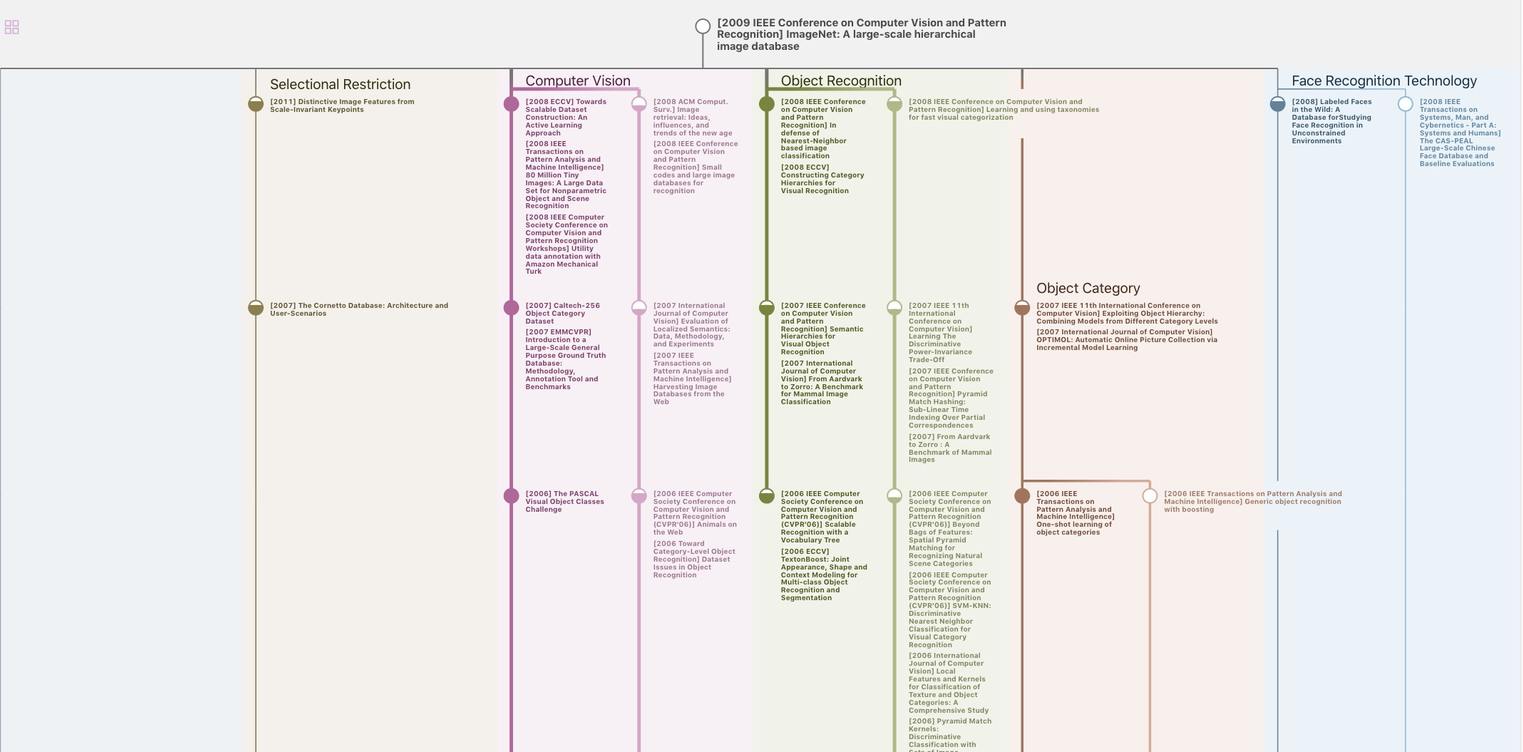
生成溯源树,研究论文发展脉络
Chat Paper
正在生成论文摘要