Deep Reinforcement Learning-Based Task Offloading for Vehicular Edge Computing With Flexible RSU-RSU Cooperation
IEEE TRANSACTIONS ON INTELLIGENT TRANSPORTATION SYSTEMS(2024)
摘要
Vehicle edge computing (VEC) acts as an enhancement to provide low latency and low energy consumption for internet of vehicles (IoV) applications. Mobility of vehicles and load difference of roadside units (RSUs) are two important issues in VEC. The former results in task result reception failures owing to vehicles moving out of the coverage of their current RSUs; the latter leads to system performance degradation owing to load imbalance among the RSUs. They can be well solved by exploiting flexible RSU-RSU cooperation, which has not been fully studied by existing works. In this paper, we propose a novel resource management scheme for joint task offloading, computing resource allocation for vehicles and RSUs, vehicle-to-RSU transmit power allocation, and RSU-to-RSU transmission rate allocation. In our scheme, a task result can be transferred to the RSU where the vehicle is currently located, and a task can be further offloaded from a high-load RSU to a low-load RSU. To minimize the total task processing delay and energy consumption of all the vehicles, we design a twin delayed deep deterministic policy gradient (TD3)-based deep reinforcement learning (DRL) algorithm, where we embed an optimization subroutine to solve 2 sub-problems via numerical methods, thus reducing the training complexity of the algorithm. Extensive simulations are conducted in 6 different scenarios. Compared with 4 reference schemes, our scheme can reduce the total task processing cost by 17.3%-28.4%.
更多查看译文
关键词
Edge computing,internet of vehicles,vehicle mobility,resource management,task offloading
AI 理解论文
溯源树
样例
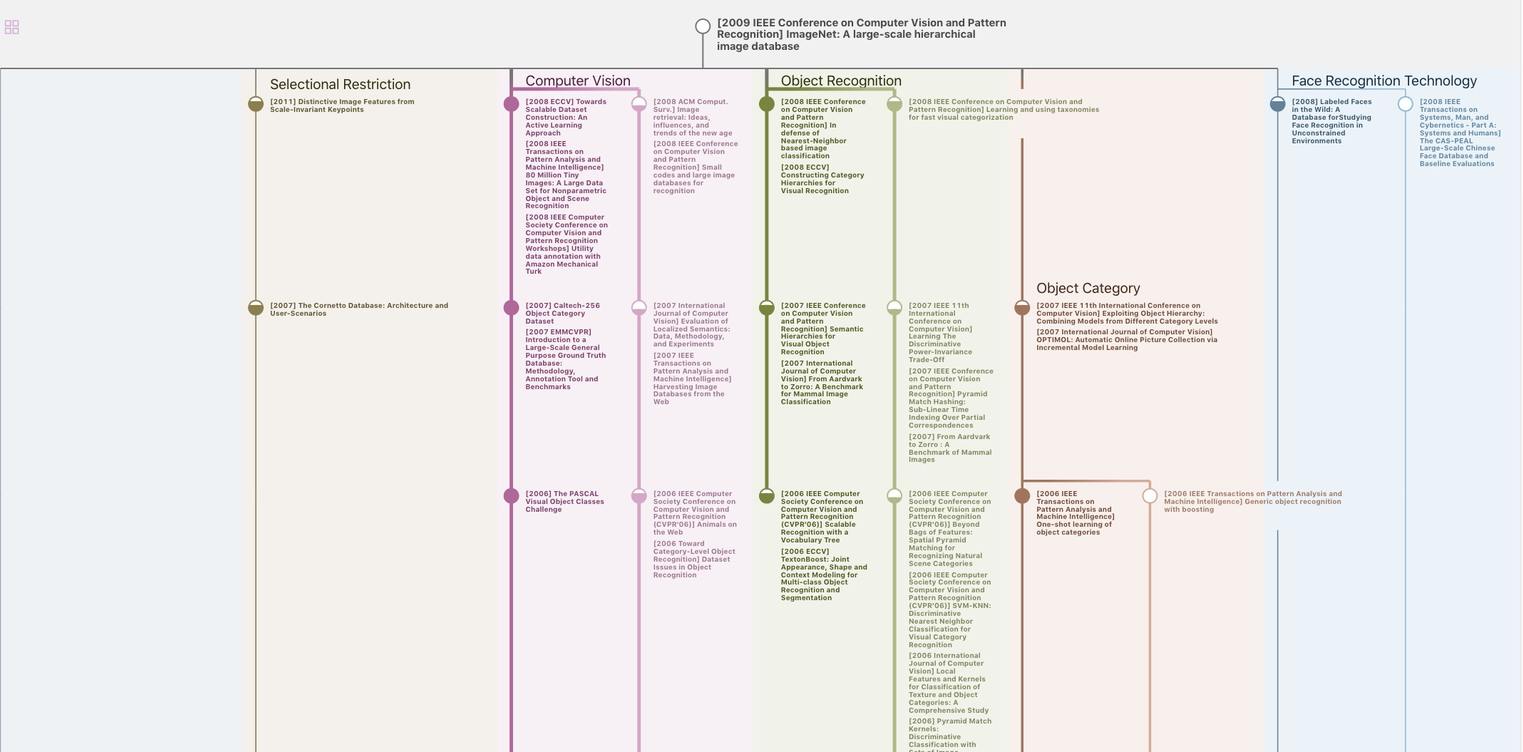
生成溯源树,研究论文发展脉络
Chat Paper
正在生成论文摘要