Hybrid Machine Learning Approach for Resource Allocation of Digital Twin in UAV-Aided Internet-of-Vehicles Networks.
IEEE Trans. Intell. Veh.(2024)
摘要
In this study, we present a novel approach for efficient resource allocation in a digital twin (DT) framework for task offloading in a UAV-aided Internet-of-Vehicles (IoV) network. Our approach incorporates a hybrid machine learning approach that combines asynchronous federated learning (AFL) and multi-agent deep reinforcement learning (DRL) to jointly optimize task completion rate, energy consumption, and delay parameters, enhancing overall system efficiency. We instantiate a DT infrastructure within a UAV-assisted IoV network for V2V and V2I task offloading with three task processing modes and three types of tasks. The DT network is composed of three distinct DTs: task vehicles, service vehicles, and roadside units. Subsequently, we formulate an optimization problem aimed at maximizing the system efficiency while concurrently minimizing delay and total energy consumption. To address this challenging non-convex problem, we introduce a multi-agent DRL algorithm named MARS for resource allocation within the DT-assisted IoV network. This innovative algorithm, MARS, is trained to utilize a hybrid AFL approach referred to as HAFL. MARS optimizes the allocation of resources across various modes of computation, striving to maximize the system's overall utility. Finally, our proposed approach's effectiveness is validated through comprehensive simulation results, where it is compared against various benchmark schemes for evaluation.
更多查看译文
关键词
Internet of Vehicles (IoV),digital twin (DT),deep reinforcement learning (DRL),asynchronous federated learning (AFL)
AI 理解论文
溯源树
样例
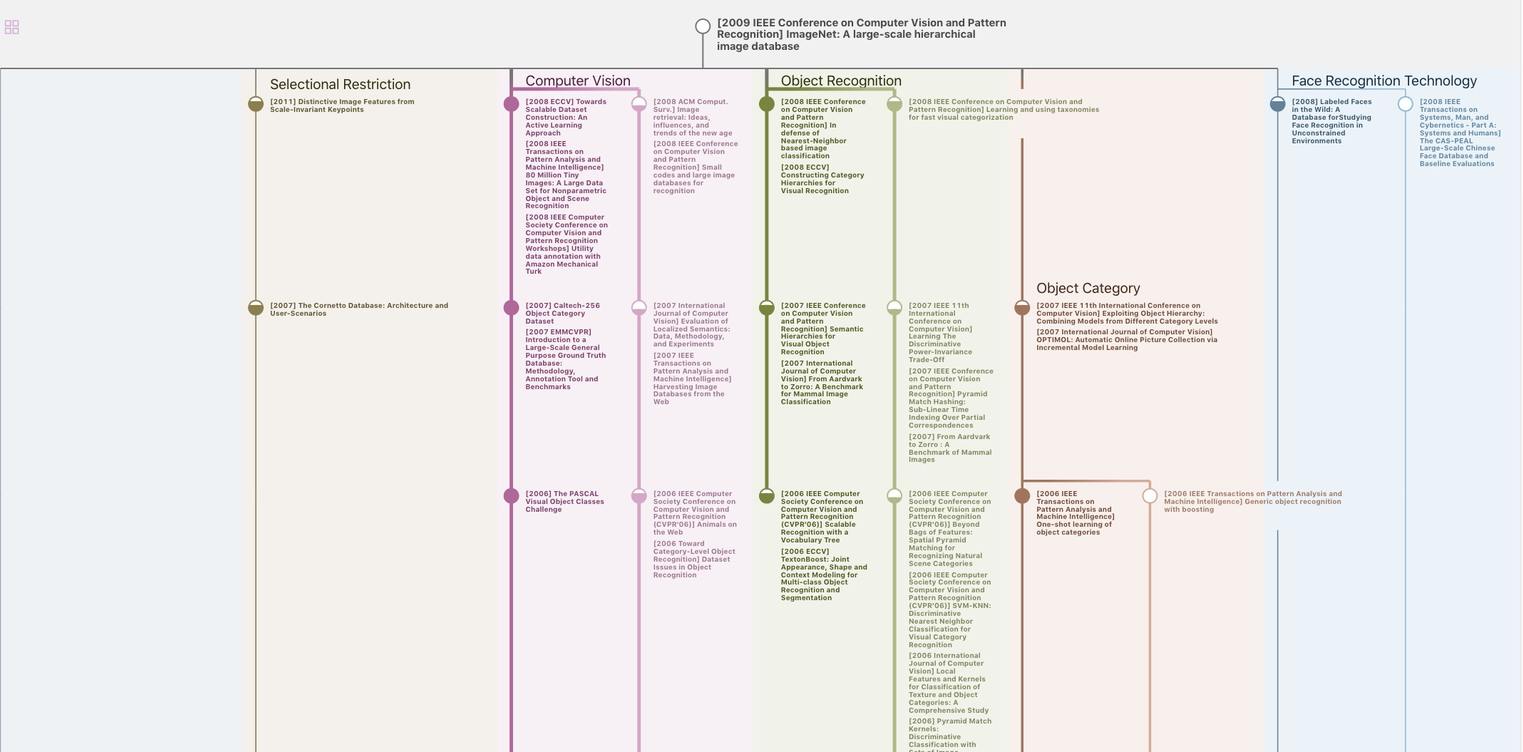
生成溯源树,研究论文发展脉络
Chat Paper
正在生成论文摘要