A Novel Framework Combining MPC and Deep Reinforcement Learning With Application to Freeway Traffic Control
IEEE TRANSACTIONS ON INTELLIGENT TRANSPORTATION SYSTEMS(2024)
摘要
Model predictive control (MPC) and deep reinforcement learning (DRL) have been developed extensively as two independent techniques for traffic management. Although the features of MPC and DRL complement each other very well, few of the current studies consider combining these two methods for application in the field of freeway traffic control. This paper proposes a novel framework for integrating MPC and DRL methods for freeway traffic control that is different from existing MPC-(D)RL methods. Specifically, the proposed framework adopts a hierarchical structure, where a high-level efficient MPC component works at a low frequency to provide a baseline control input, while the DRL component works at a high frequency to modify online the output generated by MPC. The control framework, therefore, needs only limited online computational resources and is able to handle uncertainties and external disturbances after proper learning with enough training data. The proposed framework is implemented on a benchmark freeway network in order to coordinate ramp metering and variable speed limits, and the performance is compared with standard MPC and DRL approaches. The simulation results show that the proposed framework outperforms standalone MPC and DRL methods in terms of total time spent (TTS) and constraint satisfaction, despite model uncertainties and external disturbances.
更多查看译文
关键词
Traffic control,Uncertainty,Mathematical models,Optimization,Sun,Safety,Reinforcement learning,Freeway network management,model predictive control,deep reinforcement learning,hierarchical structure
AI 理解论文
溯源树
样例
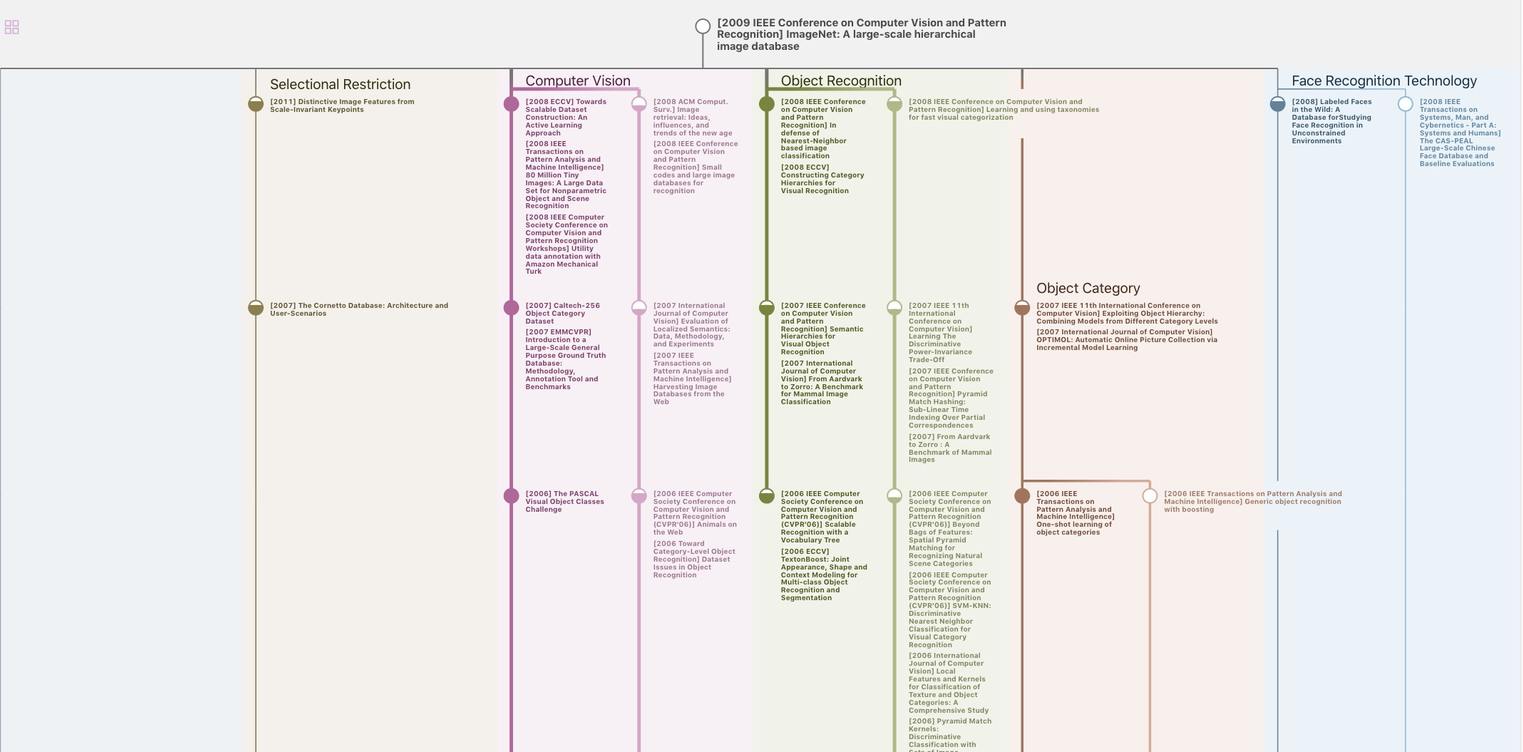
生成溯源树,研究论文发展脉络
Chat Paper
正在生成论文摘要