A Variational Graph Partitioning Approach to Modeling Protein Liquid-liquid Phase Separation
biorxiv(2024)
摘要
Protein Liquid-Liquid Phase Separation (LLPS) plays an essential role in cellular processes and is known to be associated with various diseases. However, our understanding of this enigmatic phenomena remains limited. In this work, we propose a graph-neural-network(GNN)-based interpretable machine learning approach to study the intricate nature of protein structure-function relationships associated with LLPS. For many protein properties of interest, information relevant to the property is expected to be confined to local domains. For LLPS proteins, the presence of intrinsically disordered regions (IDR)s in the molecule is arguably the most important information; an adaptive GNN model which preferentially shares information within such units and avoids mixing in information from other parts of the molecule may thus enhance the prediction of LLPS proteins. To allow for the accentuation of domain restricted information, we propose a novel graph-based model with the ability to partition each protein graph into task-dependent subgraphs. Such a model is designed not only to achieve better predictive performance but also to be highly interpretable, and thus have the ability to suggest novel biological insights. In addition to achieving state-of-the-art results on the prediction of LLPS proteins from protein structure for both regulator and scaffold proteins, we examine the properties of the graph partitions identified by our model, showing these to be consistent with the annotated IDRs believed to be largely responsible for LLPS. Moreover, our method is designed in a generic way such that it can be applied to other graph-based predictive tasks with minimal adaption.
### Competing Interest Statement
The authors have declared no competing interest.
更多查看译文
AI 理解论文
溯源树
样例
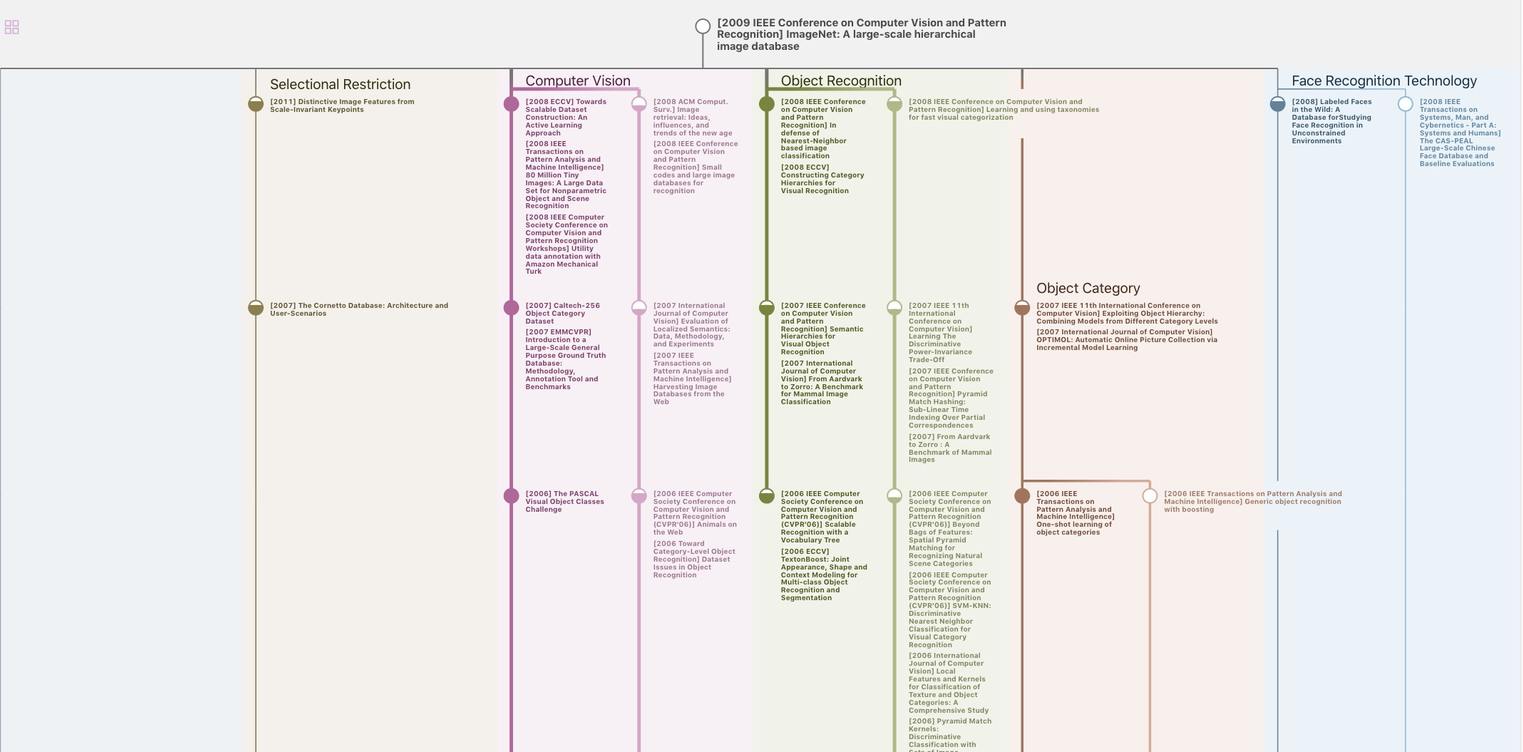
生成溯源树,研究论文发展脉络
Chat Paper
正在生成论文摘要